Region of interest selection for functional features
Neurocomputing(2021)
摘要
Feature selection is a critical component in supervised learning to improve model performance. Searching for the optimal feature candidates can be NP-hard. With limited data, cross-validation is widely used to alleviate overfitting, which unfortunately suffers from high computational cost. We propose a highly innovative strategy in feature selection to reduce the overfitting risk but without cross-validation. Our method selects the optimal sub-interval, i.e., region of interest (ROI), of a functional feature for functional linear regression where the response is a scalar and the predictor is a function. For each candidate sub-interval, we evaluate the overfitting risk by calculating a necessary sample size to achieve a pre-specified statistical power. Combining with a model accuracy measure, we rank these sub-intervals and select the ROI. The proposed method has been compared with other state-of-the-art feature selection methods on several reference datasets. The results show that our proposed method achieves an excellent performance in prediction accuracy and reduces computational cost substantially.
更多查看译文
关键词
Feature selection,Functional data,Machine learning
AI 理解论文
溯源树
样例
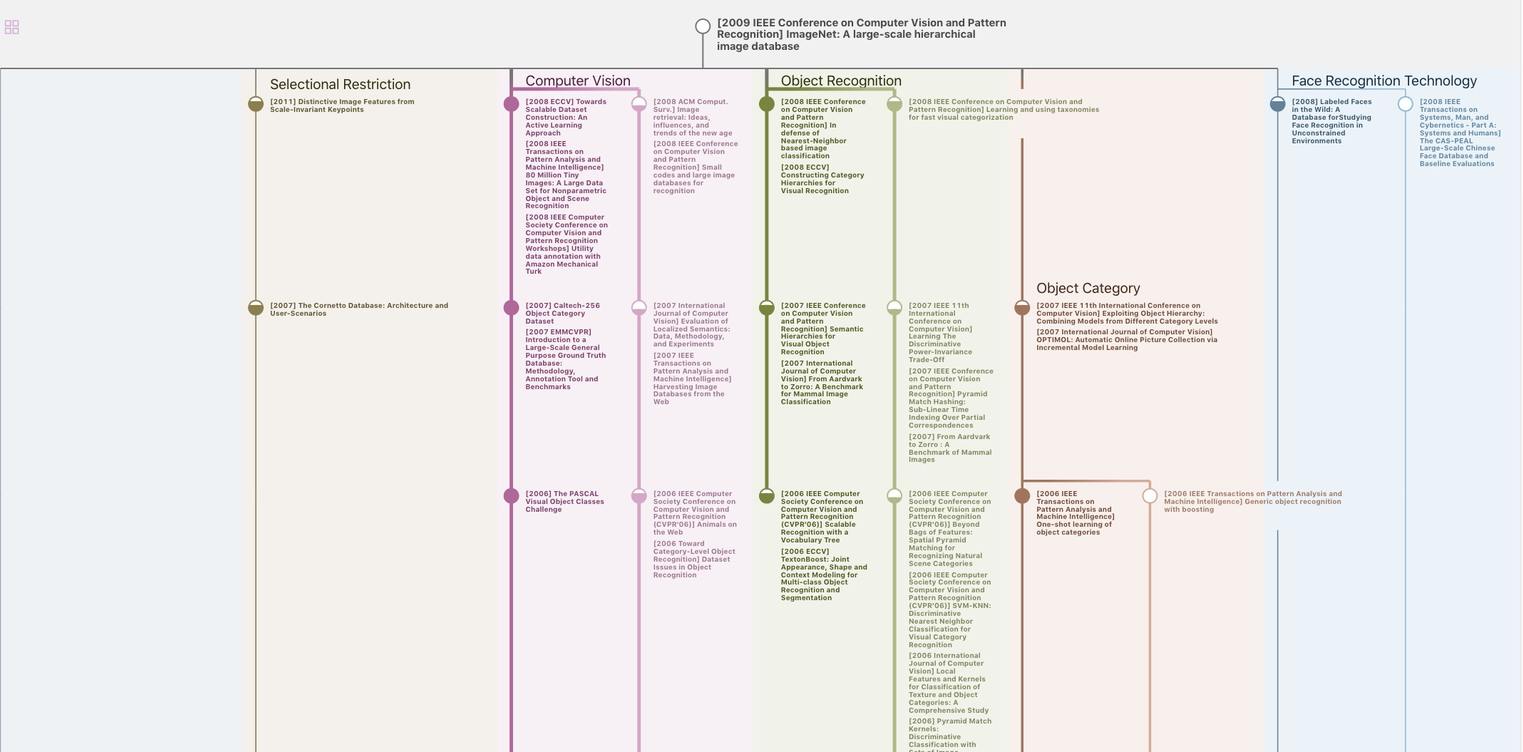
生成溯源树,研究论文发展脉络
Chat Paper
正在生成论文摘要