Multidimensional Machine Learning Personalized Prognostic Model In An Early Invasive Breast Cancer Population-Based Cohort In China: Algorithm Validation Study
JMIR MEDICAL INFORMATICS(2020)
摘要
Background: Current online prognostic prediction models for breast cancer, such as Adjuvant! Online and PREDICT, are based on specific populations. They have been well validated and widely used in the United States and Western Europe; however, several validation attempts in non-European countries have revealed suboptimal predictions.Objective: We aimed to develop an advanced breast cancer prognosis model for disease progression, cancer-specific mortality, and all-cause mortality by integrating tumor, demographic, and treatment characteristics from a large breast cancer cohort in China.Methods: This study was approved by the Clinical Test and Biomedical Ethics Committee of West China Hospital, Sichuan University on May 17, 2012. Data collection for this project was started in May 2017 and ended in March 2019. Data on 5293 women diagnosed with stage I to III invasive breast cancer between 2000 and 2013 were collected. Disease progression, cancer-specific mortality, all-cause mortality, and the likelihood of disease progression or death within a 5-year period were predicted. Extreme gradient boosting was used to develop the prediction model. Model performance was assessed by calculating the area under the receiver operating characteristic curve (AUROC), and the model was calibrated and compared with PREDICT.Results: The training, test, and validation sets comprised 3276 (499 progressions, 202 breast cancer-specific deaths, and 261 all-cause deaths within 5-year follow-up), 1405 (211 progressions, 94 breast cancer-specific deaths, and 129 all-cause deaths), and 612 (109 progressions, 33 breast cancer-specific deaths, and 37 all-cause deaths) women, respectively. The AUROC values for disease progression, cancer-specific mortality, and all-cause mortality were 0.76, 0.88, and 0.82 for training set; 0.79, 0.80, and 0.83 for the test set; and 0.79, 0.84, and 0.88 for the validation set, respectively. Calibration analysis demonstrated good agreement between predicted and observed events within 5 years. Comparable AUROC and calibration results were confirmed in different age, residence status, and receptor status subgroups. Compared with PREDICT, our model showed similar AUROC and improved calibration values.Conclusions: Our prognostic model exhibits high discrimination and good calibration. It may facilitate prognosis prediction and clinical decision making for patients with breast cancer in China.
更多查看译文
关键词
breast cancer,prognosis,machine learning,prediction model
AI 理解论文
溯源树
样例
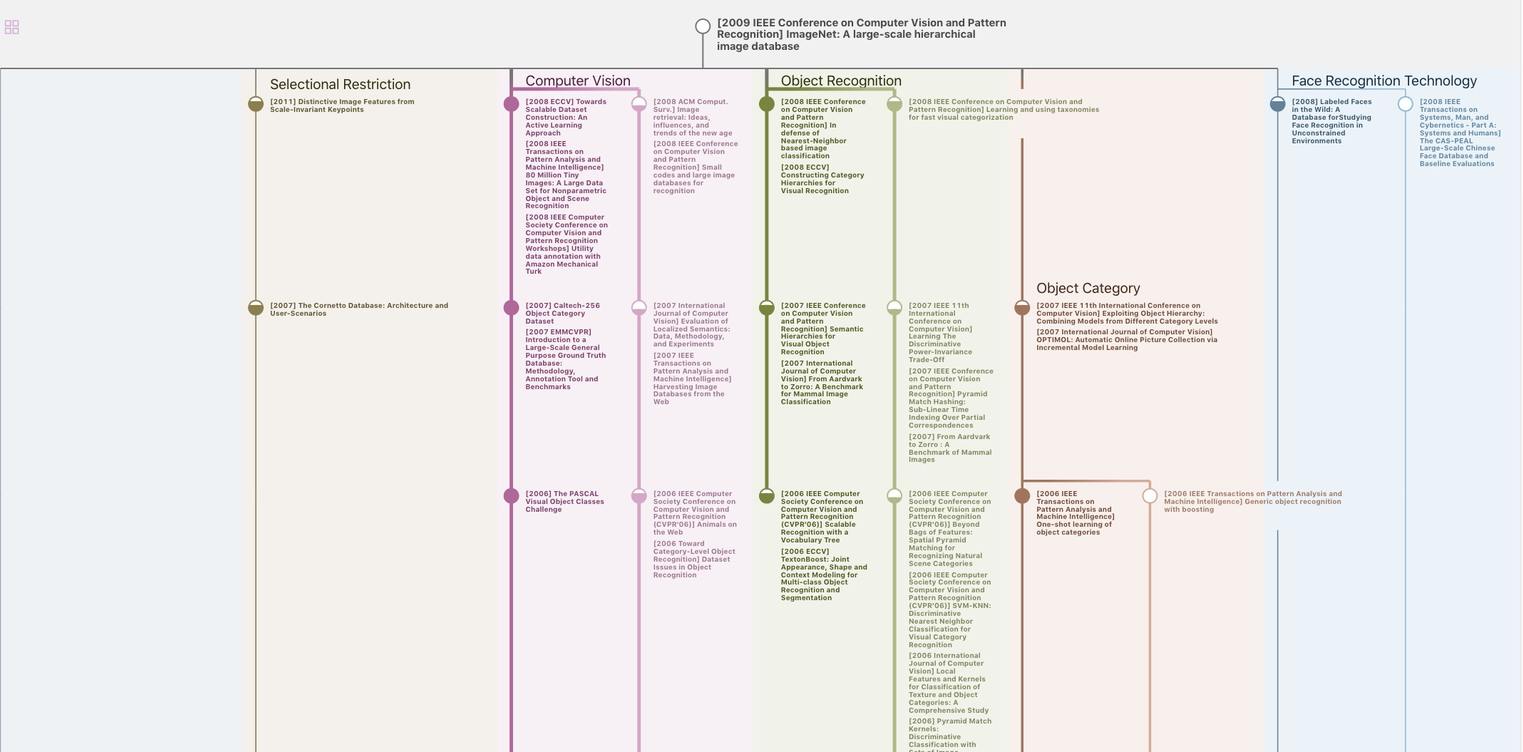
生成溯源树,研究论文发展脉络
Chat Paper
正在生成论文摘要