Adaptive Linear Span Network For Object Skeleton Detection
IEEE TRANSACTIONS ON IMAGE PROCESSING(2021)
摘要
Conventional networks for object skeleton detection are usually hand-crafted. Despite the effectiveness, hand-crafted network architectures lack the theoretical basis and require intensive prior knowledge to implement representation complementarity for objects/parts in different granularity. In this paper, we propose an adaptive linear span network (AdaLSN), driven by neural architecture search (NAS), to automatically configure and integrate scale-aware features for object skeleton detection. AdaLSN is formulated with the theory of linear span, which provides one of the earliest explanations for multi-scale deep feature fusion. AdaLSN is materialized by defining a mixed unit-pyramid search space, which goes beyond many existing search spaces using unit-level or pyramid-level features. Within the mixed space, we apply genetic architecture search to jointly optimize unit-level operations and pyramid-level connections for adaptive feature space expansion. AdaLSN substantiates its versatility by achieving significantly higher accuracy and latency trade-off compared with the state-of-the-arts. It also demonstrates general applicability to image-to-mask tasks such as edge detection and road extraction. Code is available at https://github.com/sunsmarterjie/SDL-Skeletongithub.com/sunsmarterjie/SDL-Skeleton.
更多查看译文
关键词
Feature extraction, Skeleton, Search problems, Computer architecture, Semantics, Network architecture, Transforms, Skeleton detection, linear span network, neural architecture search, genetic algorithm
AI 理解论文
溯源树
样例
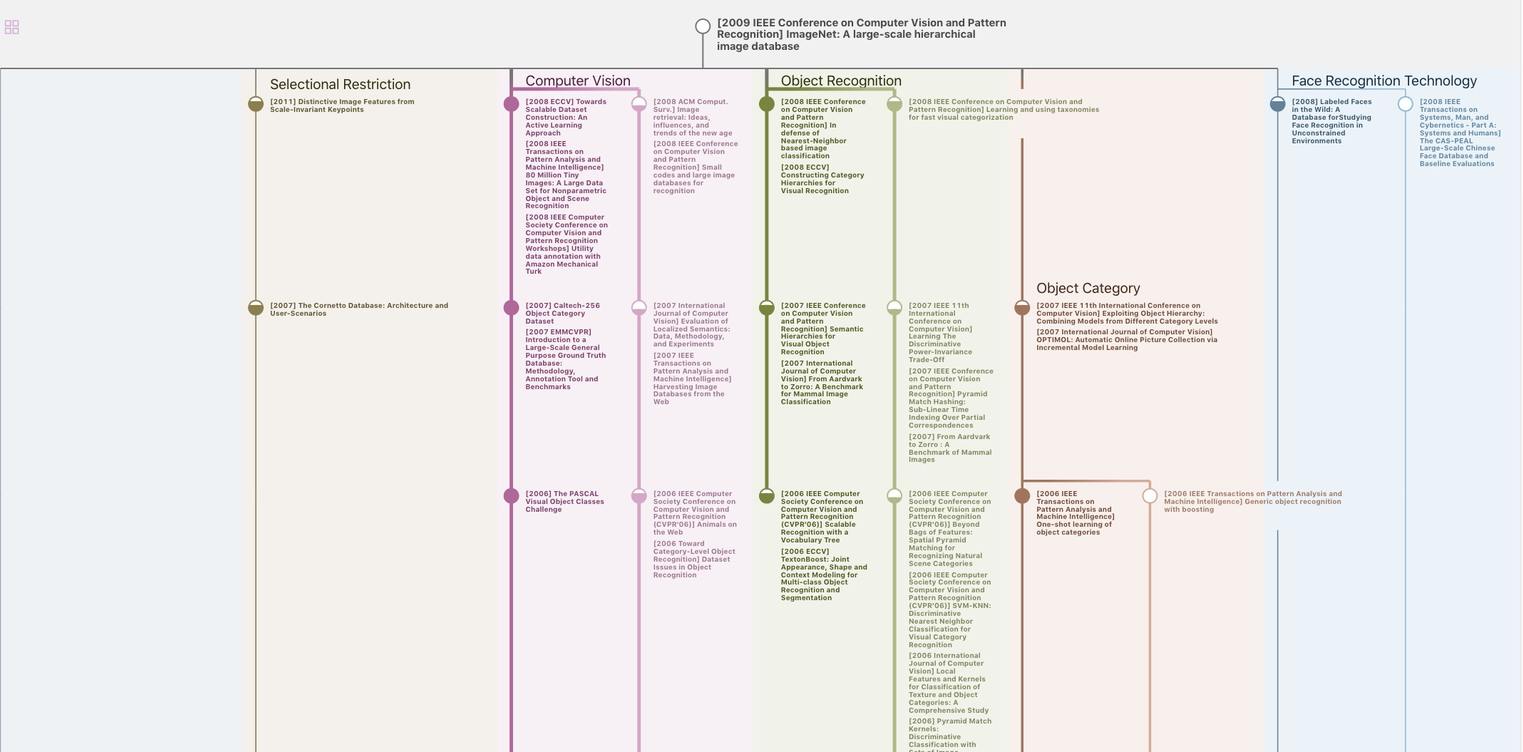
生成溯源树,研究论文发展脉络
Chat Paper
正在生成论文摘要