Stochastic Attention Head Removal: A Simple and Effective Method for Improving Automatic Speech Recognition with Transformers
arxiv(2020)
摘要
Recently, Transformers have shown competitive automatic speech recognition (ASR) results. One key factor to the success of these models is the multi-head attention mechanism. However, we observed in trained models, the diagonal attention matrices indicating the redundancy of the corresponding attention heads. Furthermore, we found some architectures with reduced numbers of attention heads have better performance. Since the search for the best structure is time prohibitive, we propose to randomly remove attention heads during training and keep all attention heads at test time, thus the final model can be viewed as an average of models with different architectures. This method gives consistent performance gains on the Wall Street Journal, AISHELL, Switchboard and AMI ASR tasks. On the AISHELL dev/test sets, the proposed method achieves state-of-the-art Transformer results with 5.8%/6.3% word error rates.
更多查看译文
关键词
Transformer (machine learning model),Redundancy (engineering),Machine learning,Effective method,Computer science,Key (cryptography),Diagonal,Structure (mathematical logic),Simple (abstract algebra),Head (linguistics),Artificial intelligence
AI 理解论文
溯源树
样例
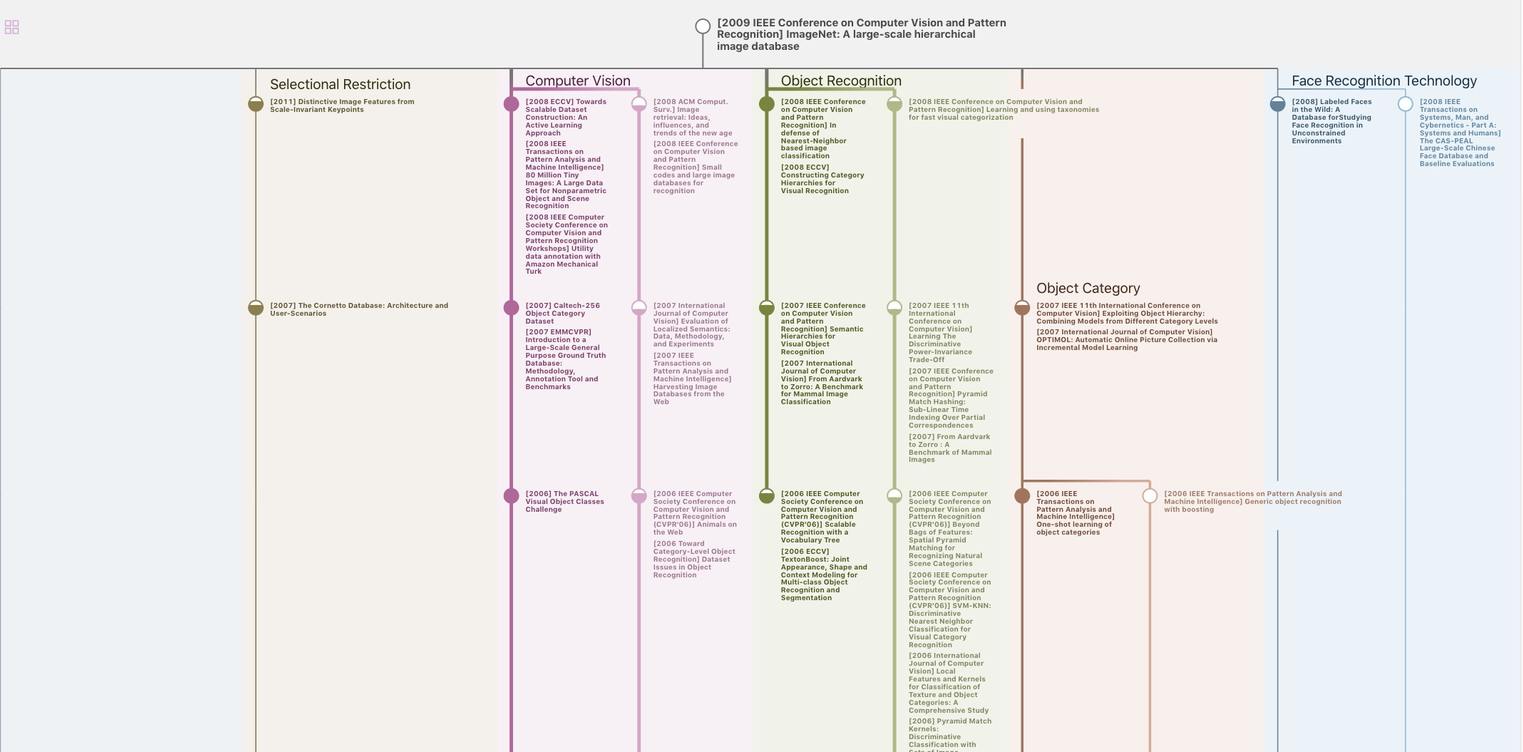
生成溯源树,研究论文发展脉络
Chat Paper
正在生成论文摘要