Modeling and Optimizing Resource Allocation Decisions through Multi-model Markov Decision Processes with Capacity Constraints
arxiv(2020)
摘要
This paper proposes a new formulation for the dynamic resource allocation problem, which converts the traditional MDP model with known parameters and no capacity constraints to a new model with uncertain parameters and a resource capacity constraint. Our motivating example comes from a medical resource allocation problem: patients with multiple chronic diseases can be provided either normal or special care, where the capacity of special care is limited due to financial or human resources. In such systems, it is difficult, if not impossible, to generate good estimates for the evolution of health for each patient. We formulate the problem as a two-stage stochastic integer program. However, it becomes easily intractable in larger instances of the problem for which we propose and test a parallel approximate dynamic programming algorithm. We show that commercial solvers are not capable of solving the problem instances with a large number of scenarios. Nevertheless, the proposed algorithm provides a solution in seconds even for very large problem instances. In our computational experiments, it finds the optimal solution for $42.86\%$ of the instances. On aggregate, it achieves $0.073\%$ mean gap value. Finally, we estimate the value of our contribution for different realizations of the parameters. Our findings show that there is a significant amount of additional utility contributed by our model.
更多查看译文
关键词
optimizing resource allocation decisions,capacity constraints,processes,multi-model
AI 理解论文
溯源树
样例
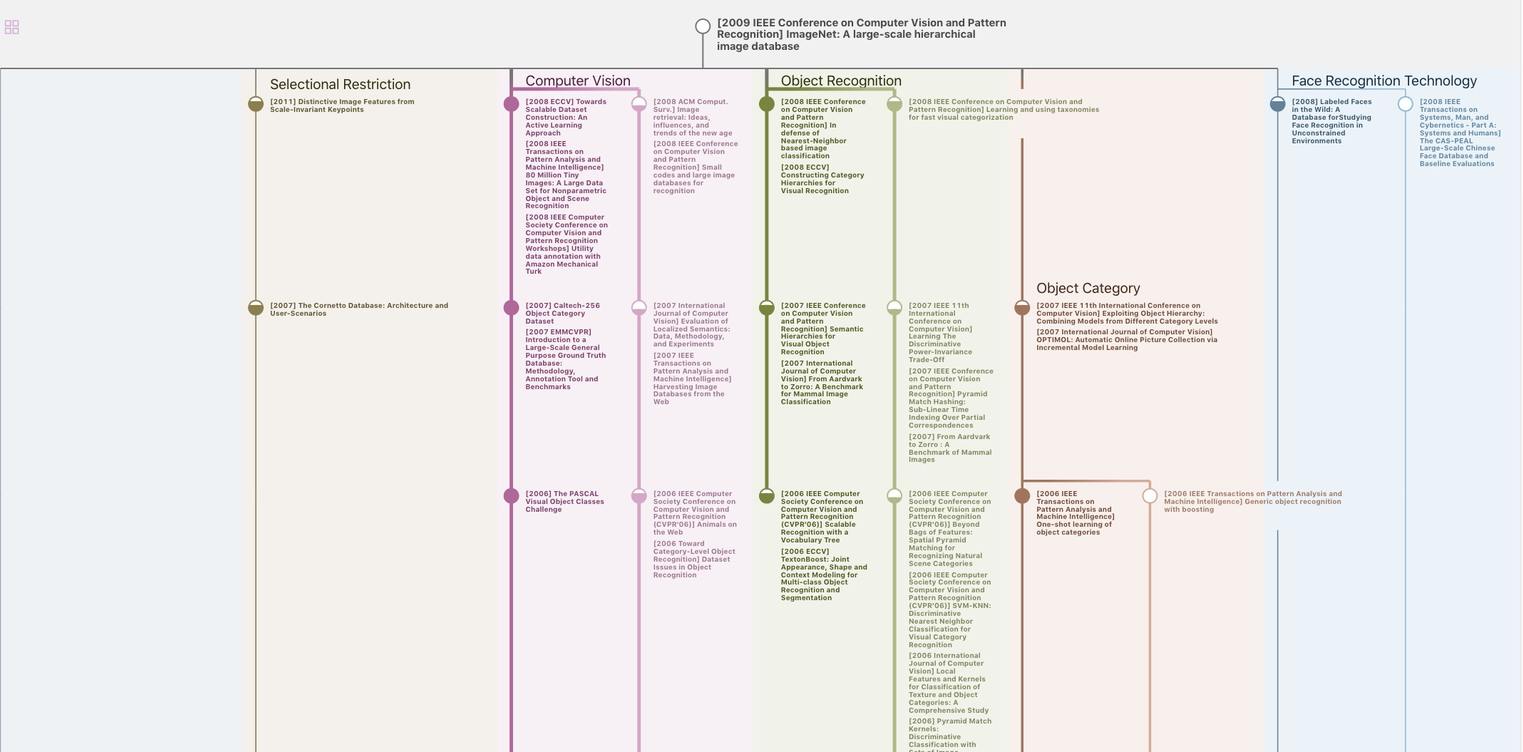
生成溯源树,研究论文发展脉络
Chat Paper
正在生成论文摘要