DIPN: Deep Interaction Prediction Network with Application to Clutter Removal
2021 IEEE INTERNATIONAL CONFERENCE ON ROBOTICS AND AUTOMATION (ICRA 2021)(2021)
摘要
We propose a Deep Interaction Prediction Network (DIPN) for learning to predict complex interactions that ensue as a robot end-effector pushes multiple objects, whose physical properties, including size, shape, mass, and friction coefficients may be unknown a priori. DIPN "imagines" the effect of a push action and generates an accurate synthetic image of the predicted outcome. DIPN is shown to be sample efficient when trained in simulation or with a real robotic system. The high accuracy of DIPN allows direct integration with a grasp network, yielding a robotic manipulation system capable of executing challenging clutter removal tasks while being trained in a fully self-supervised manner. The overall network demonstrates intelligent behavior in selecting proper actions between push and grasp for completing clutter removal tasks and significantly outperforms the previous state-of-the-art. Remarkably, DIPN achieves even better performance on the real robotic hardware system than in simulation.
更多查看译文
关键词
robotic manipulation system,robotic hardware system,complex interactions,robot end-effector pushes multiple objects,DIPN imagines,push action,predicted outcome,grasp network,deep interaction prediction network,clutter removal tasks,physical properties,friction coefficients,synthetic image,self-supervised manner
AI 理解论文
溯源树
样例
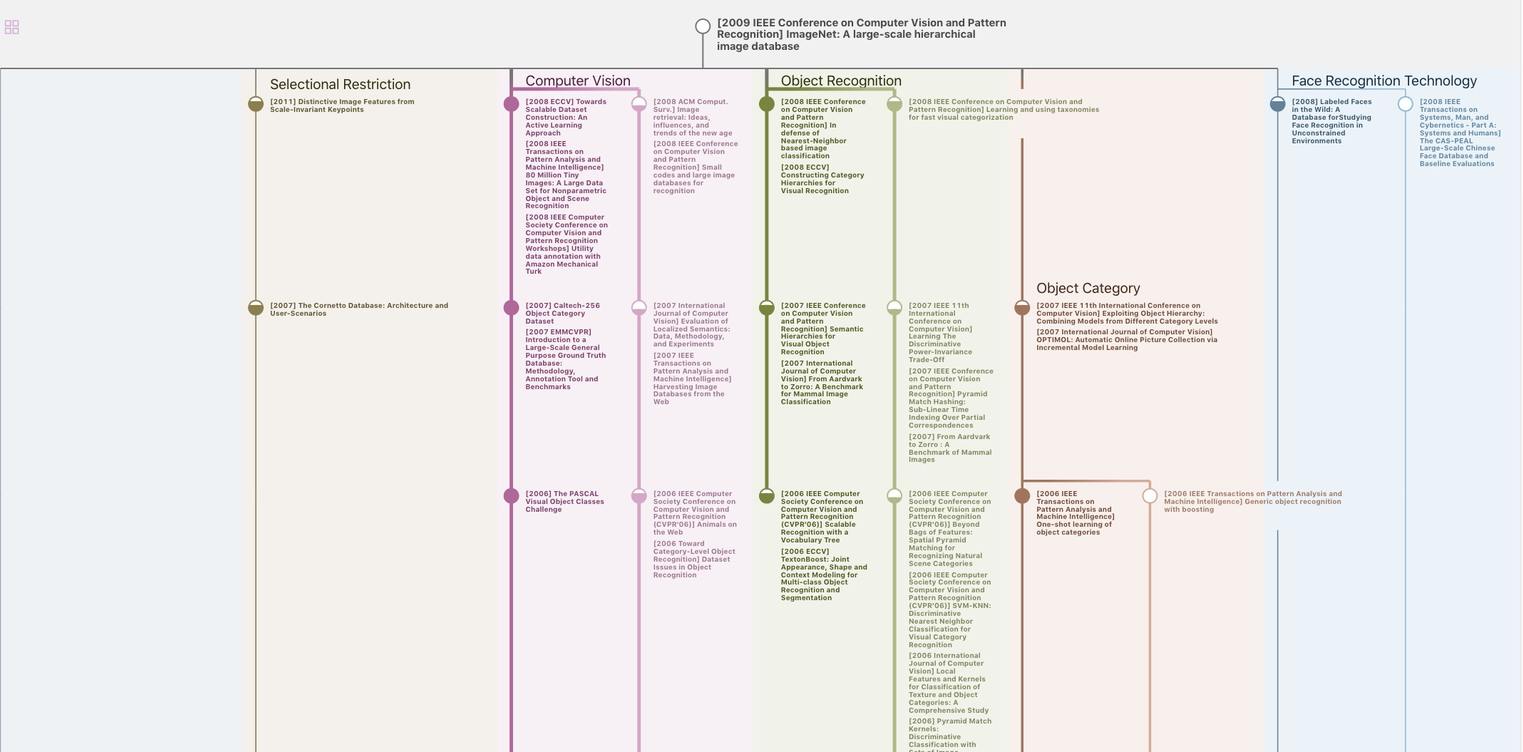
生成溯源树,研究论文发展脉络
Chat Paper
正在生成论文摘要