MUSE: Illustrating Textual Attributes by Portrait Generation
arxiv(2021)
摘要
We propose a novel approach, MUSE, to illustrate textual attributes visually via portrait generation. MUSE takes a set of attributes written in text, in addition to facial features extracted from a photo of the subject as input. We propose 11 attribute types to represent inspirations from a subject's profile, emotion, story, and environment. We propose a novel stacked neural network architecture by extending an image-to-image generative model to accept textual attributes. Experiments show that our approach significantly outperforms several state-of-the-art methods without using textual attributes, with Inception Score score increased by 6% and Fr\'echet Inception Distance (FID) score decreased by 11%, respectively. We also propose a new attribute reconstruction metric to evaluate whether the generated portraits preserve the subject's attributes. Experiments show that our approach can accurately illustrate 78% textual attributes, which also help MUSE capture the subject in a more creative and expressive way.
更多查看译文
关键词
textual attributes,portrait generation,attribute,generative model,MUSE
AI 理解论文
溯源树
样例
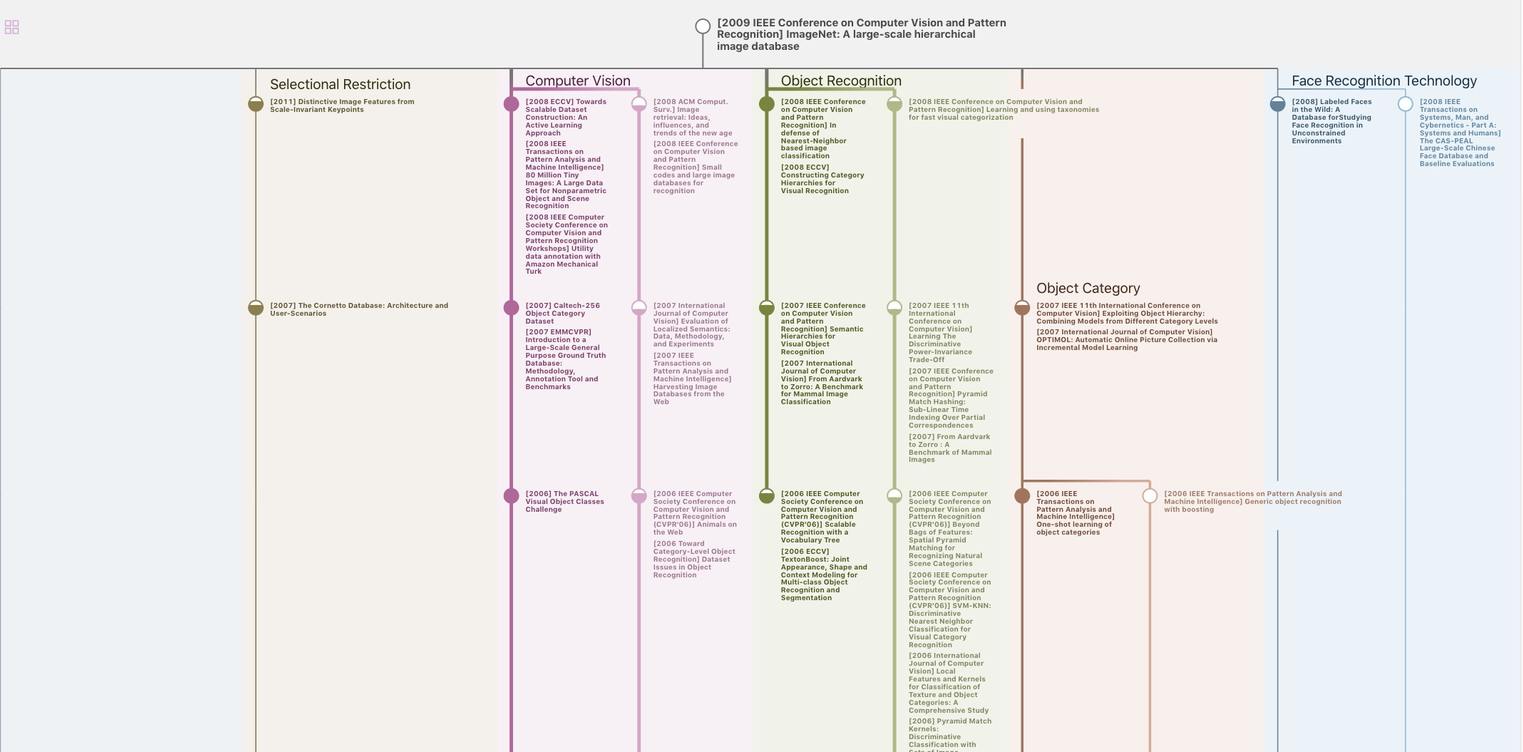
生成溯源树,研究论文发展脉络
Chat Paper
正在生成论文摘要