Machine-Learning Algorithms For Predicting Hospital Re-Admissions In Sickle Cell Disease
BRITISH JOURNAL OF HAEMATOLOGY(2021)
摘要
Reducing preventable hospital re-admissions in Sickle Cell Disease (SCD) could potentially improve outcomes and decrease healthcare costs. In a retrospective study of electronic health records, we hypothesized Machine-Learning (ML) algorithms may outperform standard re-admission scoring systems (LACE and HOSPITAL indices). Participants (n = 446) included patients with SCD with at least one unplanned inpatient encounter between January 1, 2013, and November 1, 2018. Patients were randomly partitioned into training and testing groups. Unplanned hospital admissions (n = 3299) were stratified to training and testing samples. Potential predictors (n = 486), measured from the last unplanned inpatient discharge to the current unplanned inpatient visit, were obtained via both data-driven methods and clinical knowledge. Three standard ML algorithms, Logistic Regression (LR), Support-Vector Machine (SVM), and Random Forest (RF) were applied. Prediction performance was assessed using the C-statistic, sensitivity, and specificity. In addition, we reported the most important predictors in our best models. In this dataset, ML algorithms outperformed LACE [C-statistic 0 center dot 6, 95% Confidence Interval (CI) 0 center dot 57-0 center dot 64] and HOSPITAL (C-statistic 0 center dot 69, 95% CI 0 center dot 66-0 center dot 72), with the RF (C-statistic 0 center dot 77, 95% CI 0 center dot 73-0 center dot 79) and LR (C-statistic 0 center dot 77, 95% CI 0 center dot 73-0 center dot 8) performing the best. ML algorithms can be powerful tools in predicting re-admission in high-risk patient groups.
更多查看译文
关键词
30‐, day unplanned hospital readmission, machine learning, prediction, retrospective study, sickle cell disease
AI 理解论文
溯源树
样例
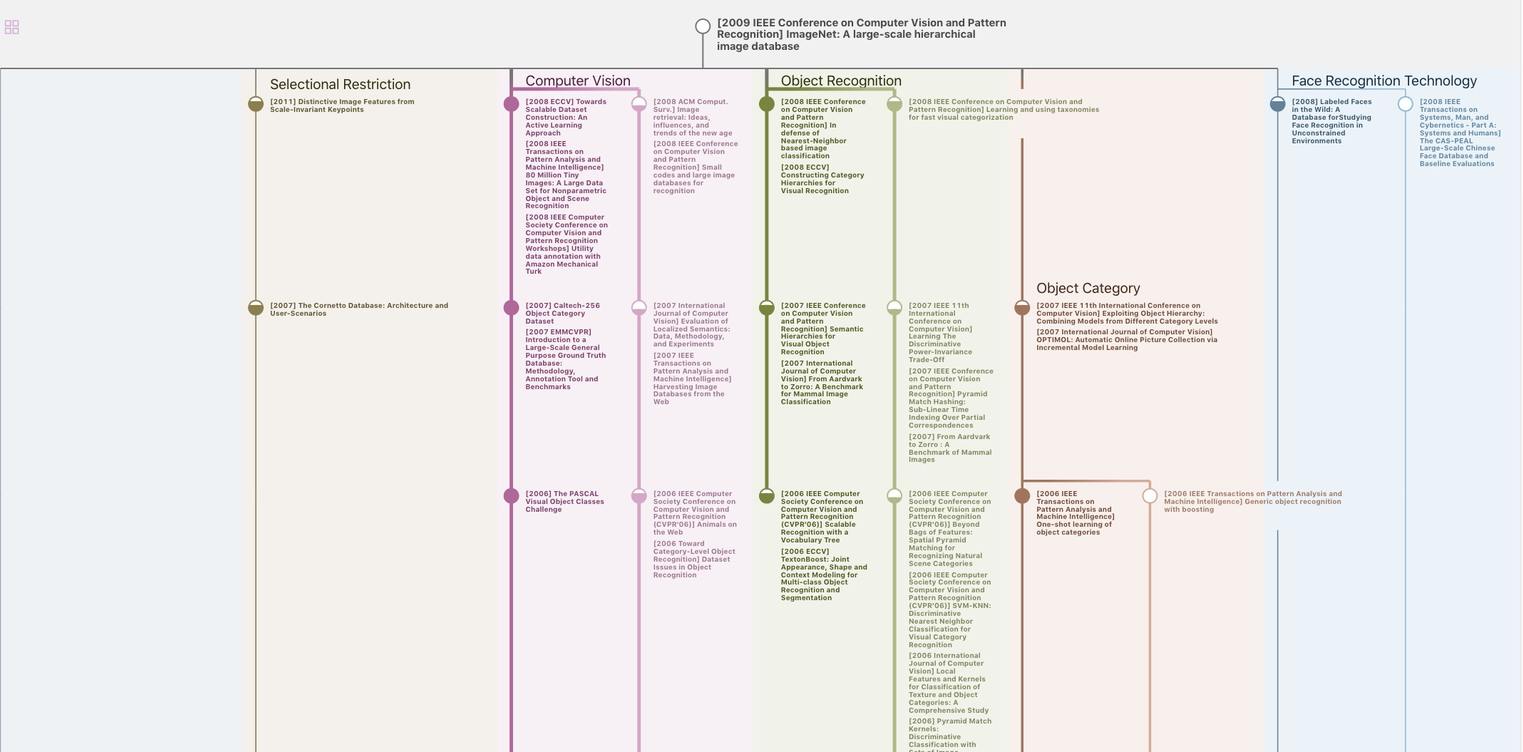
生成溯源树,研究论文发展脉络
Chat Paper
正在生成论文摘要