Amadeus: Scalable, Privacy-Preserving Live Video Analytics
arxiv(2020)
摘要
Smart-city applications ranging from traffic management to public-safety alerts rely on live analytics of video from surveillance cameras in public spaces. However, a growing number of government regulations stipulate how data collected from these cameras must be handled in order to protect citizens' privacy. This paper describes Amadeus, which balances privacy and utility by redacting video in near realtime for smart-city applications. Our main insight is that whitelisting objects, or blocking by default, is crucial for scalable, privacy-preserving video analytics. In the context of modern object detectors, we prove that whitelisting reduces the risk of an object-detection error leading to a privacy violation, and helps Amadeus scale to a large and diverse set of applications. In particular, Amadeus utilizes whitelisting to generate composable encrypted object-specific live streams, which simultaneously meet the requirements of multiple applications in a privacy-preserving fashion, while reducing the compute and streaming-bandwidth requirements at the edge. Experiments with our Amadeus prototype show that compared to blacklisting objects, whitelisting yields significantly better privacy (up to ~28x) and bandwidth savings (up to ~5.5x). Additionally, our experiments also indicate that the composable live streams generated by Amadeus are usable by real-world applications with minimum utility loss.
更多查看译文
关键词
analytics,scalable,video,privacy-preserving
AI 理解论文
溯源树
样例
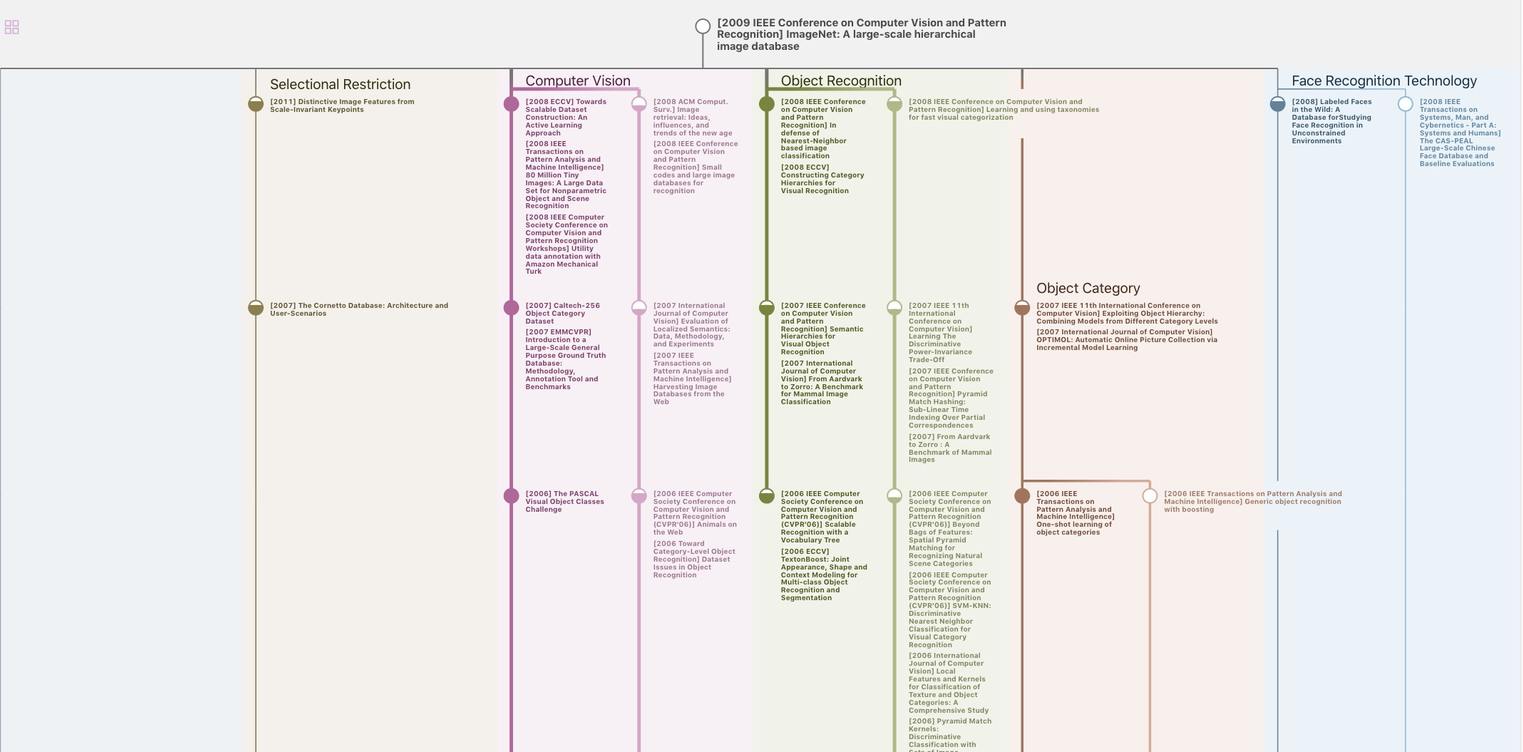
生成溯源树,研究论文发展脉络
Chat Paper
正在生成论文摘要