Rediscovering alignment relations with Graph Convolutional Networks
arxiv(2021)
摘要
Knowledge graphs are concurrently published and edited in the Web of data. Hence they may overlap, which makes key the task that consists in matching their content. This task encompasses the identification, within and across knowledge graphs, of nodes that are equivalent, more specific, or weakly related. In this article, we propose to match nodes of a knowledge graph by (i) learning node embeddings with Graph Convolutional Networks such that similar nodes have low distances in the embedding space, and (ii) clustering nodes based on their embeddings. We experimented this approach on a biomedical knowledge graph and particularly investigated the interplay between formal semantics and GCN models with the two following main focuses. Firstly, we applied various inference rules associated with domain knowledge, independently or combined, before learning node embeddings, and we measured the improvements in matching results. Secondly, while our GCN model is agnostic to the exact alignment relations (e.g., equivalence, weak similarity), we observed that distances in the embedding space are coherent with the "strength" of these different relations (e.g., smaller distances for equivalences), somehow corresponding to their rediscovery by the model.
更多查看译文
关键词
Knowledge graph,matching,embedding,Graph Convolutional Network,ontology,clustering
AI 理解论文
溯源树
样例
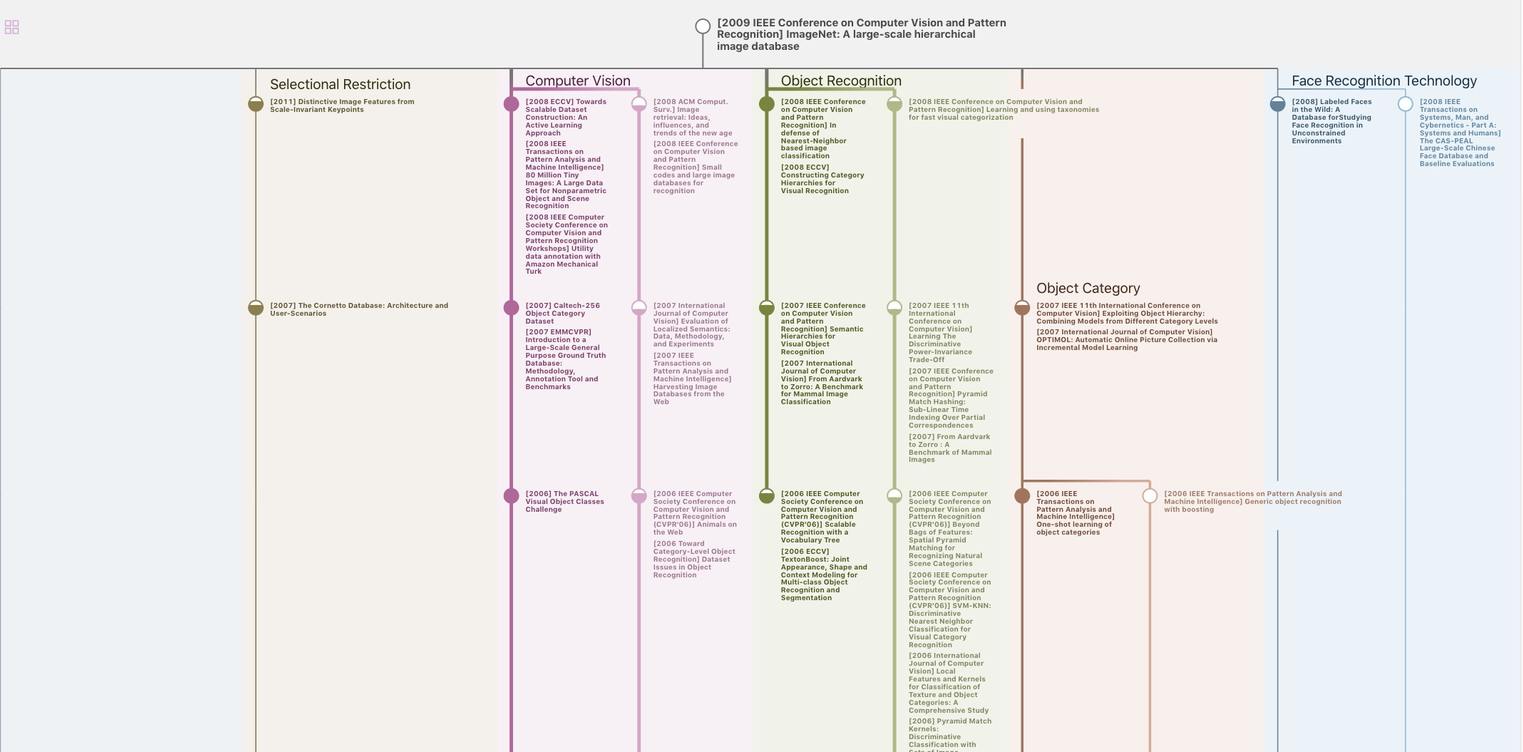
生成溯源树,研究论文发展脉络
Chat Paper
正在生成论文摘要