Sketch and Scale: Geo-distributed tSNE and UMAP
2020 IEEE INTERNATIONAL CONFERENCE ON BIG DATA (BIG DATA)(2020)
摘要
Running machine learning analytics over geographically distributed datasets is a rapidly arising problem in the world of data management policies ensuring privacy and data security. Visualizing high dimensional data using tools such as t-distributed Stochastic Neighbor Embedding (tSNE) and Uniform Manifold Approximation and Projection (UMAP) became common practice for data scientists. Both tools scale poorly in time and memory. While recent optimizations showed successful handling of 10,000 data points, scaling beyond million points is still challenging. We introduce a novel framework: Sketch and Scale (SnS). It leverages a Count Sketch data structure to compress the data on the edge nodes, aggregates the reduced size sketches on the master node, and runs vanilla tSNE or UMAP on the summary, representing the densest areas, extracted from the aggregated sketch. We show this technique to be fully parallel, scale linearly in time, logarithmically in memory, and communication, making it possible to analyze datasets with many millions, potentially billions of data points, spread across several data centers around the globe. We demonstrate the power of our method on two mid-size datasets: cancer data with 52 million 35-band pixels from multiple images of tumor biopsies; and astrophysics data of 100 million stars with multi-color photometry from the Sloan Digital Sky Survey (SDSS).
更多查看译文
关键词
count sketch, heavy hitter, scalable, umap, tsne, geo-distributed
AI 理解论文
溯源树
样例
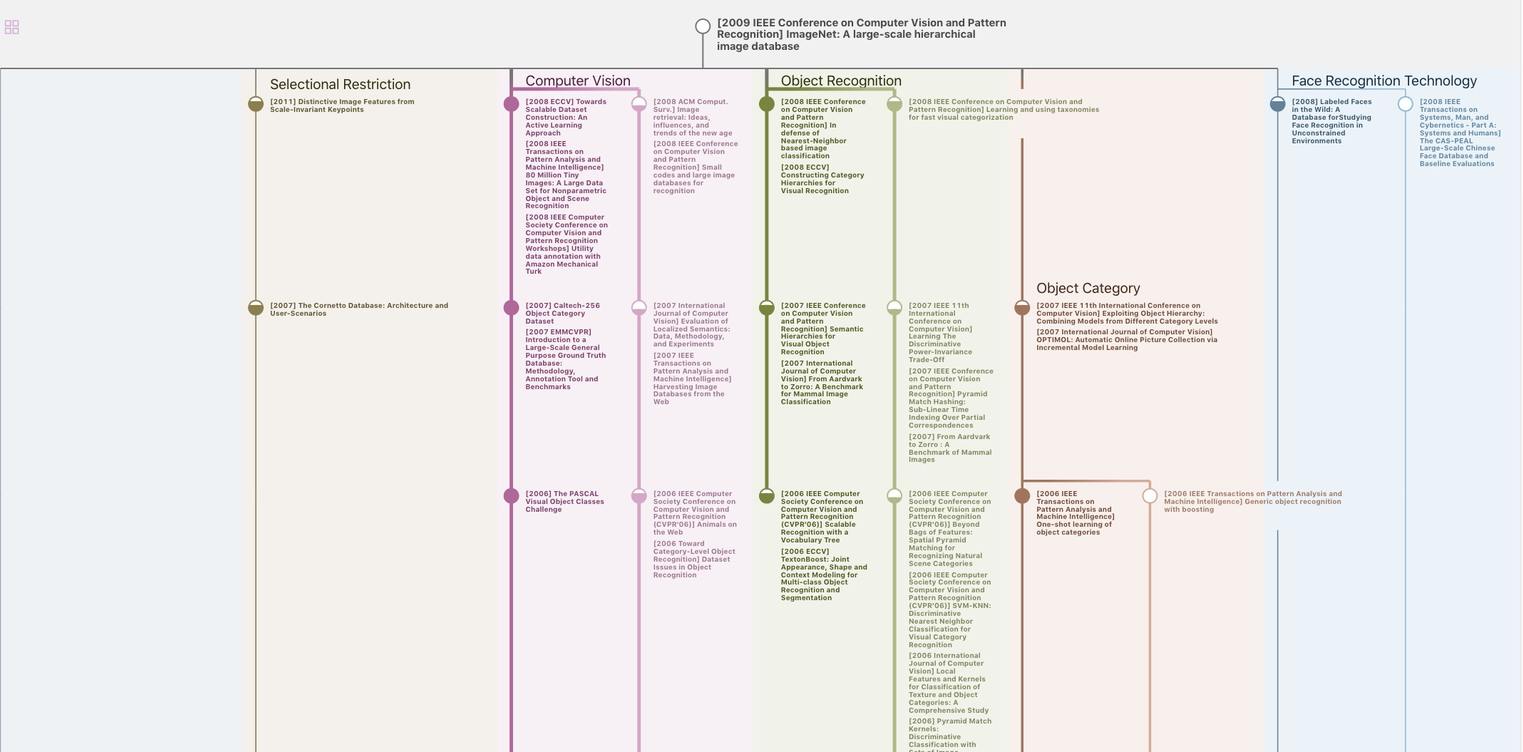
生成溯源树,研究论文发展脉络
Chat Paper
正在生成论文摘要