Classification of 12-Lead ECGs: the PhysioNet/Computing in Cardiology Challenge 2020
PHYSIOLOGICAL MEASUREMENT(2020)
摘要
Objective: Vast 12-lead ECGs repositories provide opportunities to develop new machine learning approaches for creating accurate and automatic diagnostic systems for cardiac abnormalities. However, most 12-lead ECG classification studies are trained, tested, or developed in single, small, or relatively homogeneous datasets. In addition, most algorithms focus on identifying small numbers of cardiac arrhythmias that do not represent the complexity and difficulty of ECG interpretation. This work addresses these issues by providing a standard, multi-institutional database and a novel scoring metric through a public competition: the PhysioNet/Computing in Cardiology Challenge 2020. Approach: A total of 66 361 12-lead ECG recordings were sourced from six hospital systems from four countries across three continents; 43 101 recordings were posted publicly with a focus on 27 diagnoses. For the first time in a public competition, we required teams to publish open-source code for both training and testing their algorithms, ensuring full scientific reproducibility. Main results: A total of 217 teams submitted 1395 algorithms during the Challenge, representing a diversity of approaches for identifying cardiac abnormalities from both academia and industry. As with previous Challenges, high-performing algorithms exhibited significant drops (less than or similar to更多
查看译文
关键词
electrocardiogram,signal processing,generalizability,reproducibility,competition,PhysioNet
AI 理解论文
溯源树
样例
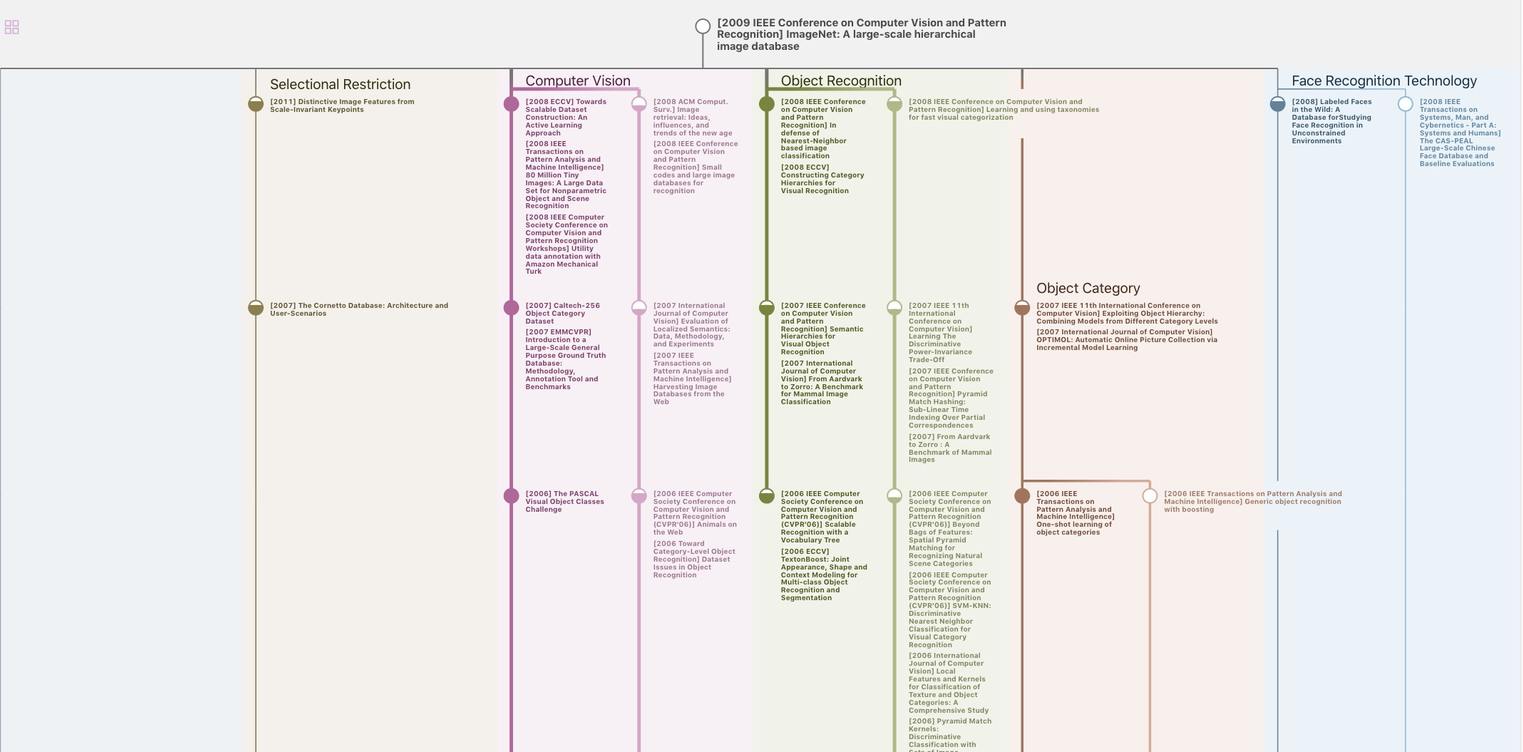
生成溯源树,研究论文发展脉络
Chat Paper
正在生成论文摘要