Dynamic Mode Decomposition Analysis of Spatially Agglomerated Flow Databases
ENERGIES(2020)
摘要
Dynamic Mode Decomposition (DMD) techniques have risen as prominent feature identification methods in the field of fluid dynamics. Any of the multiple variables of the DMD method allows to identify meaningful features from either experimental or numerical flow data on a data-driven manner. Performing a DMD analysis requires handling matrices V is an element of RnpxN , where np and N are indicative of the spatial and temporal resolutions. The DMD analysis of a complex flow field requires long temporal sequences of well resolved data, and thus the memory footprint may become prohibitively large. In this contribution, the effect that principled spatial agglomeration (i.e., reduction in np via clustering) has on the results derived from the DMD analysis is investigated. We compare twelve different clustering algorithms on three testcases, encompassing different flow regimes: a synthetic flow field, a ReD=60 flow around a cylinder cross section, and a Re tau approximate to 200 turbulent channel flow. The performance of the clustering techniques is thoroughly assessed concerning both the accuracy of the results retrieved and the computational performance. From this assessment, we identify DBSCAN/HDBSCAN as the methods to be used if only relatively high agglomeration levels are affordable. On the contrary, Mini-batch K-means arises as the method of choice whenever high agglomeration np/np<< 1 is possible.
更多查看译文
关键词
modal decompositions,turbulent flows,flow reconstruction,machine learning,clustering algorithms,spatial agglomeration,dynamic mode decomposition,feature detection
AI 理解论文
溯源树
样例
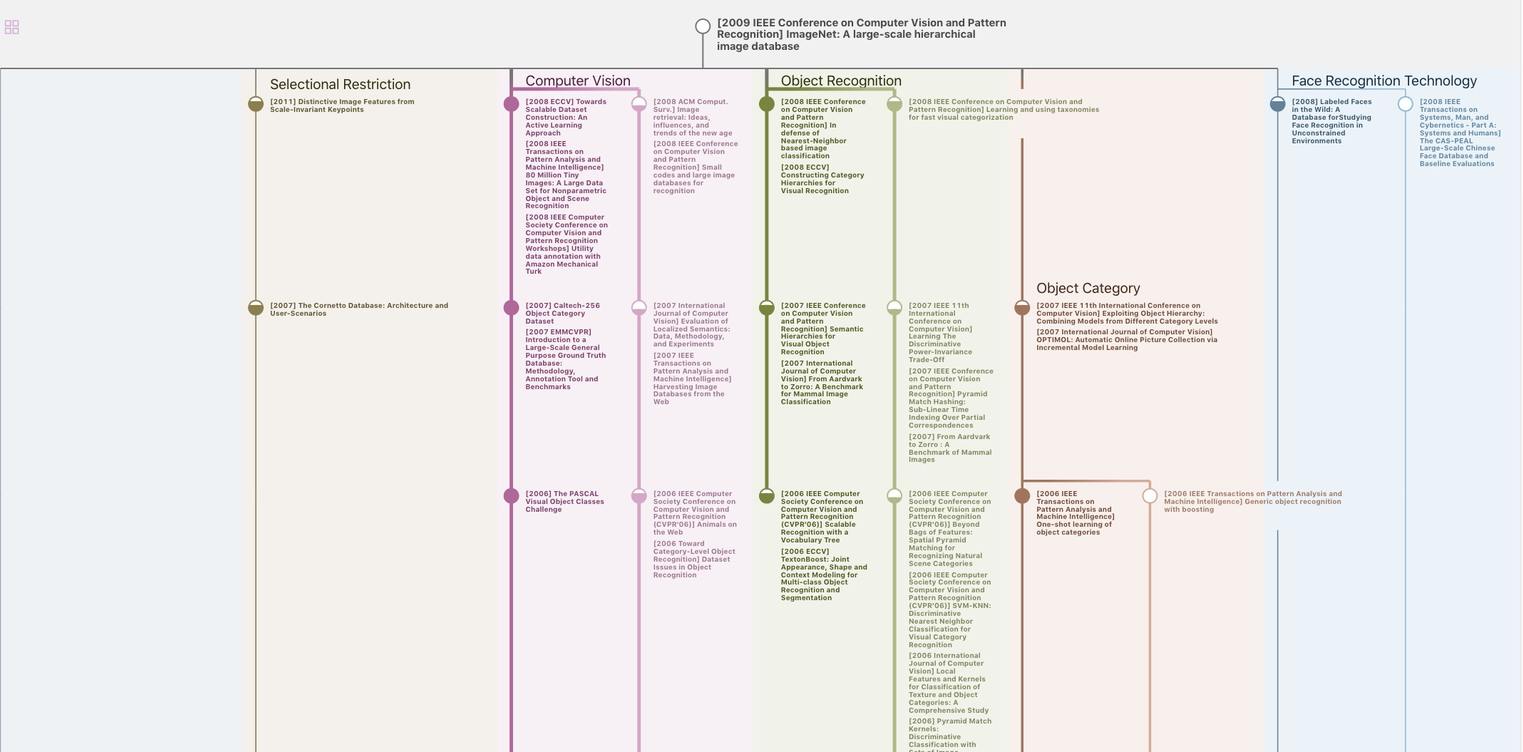
生成溯源树,研究论文发展脉络
Chat Paper
正在生成论文摘要