Discriminative-shared dictionary learning for class-specific fabric texture characterization:
TEXTILE RESEARCH JOURNAL(2020)
摘要
In this paper we introduced a novel discriminative-shared dictionary learning (DSDL) model to explicitly extract a set of class-specific features as well as shared features for simultaneous fabric texture characterization. For the discriminative component, we imposed the constraints of minimizing inter-class correlation as well as maximizing intra-class correlation on them. For the shared component, we enforced a low-rank constraint and a similarity constraint on it. To demonstrate the characterization performance of the learned dictionary on multi-class fabric textures, we weaved eight fabric textures in the laboratory and then reconstructed each class of sample with each class of specific dictionary. Preliminary experiments with four-class samples demonstrate that the specific class of dictionary can only reconstruct the corresponding class of fabric texture, but has weak reconstructive ability for the rest class, and vice versa. In addition, we illustrated the contribution of shared dictionary by comparing the convergence rate of DSDL with that of Fisher Discriminative Dictionary Learning. Further, we developed a new indicator based on a similarity matrix for evaluating the discriminative of class-specific dictionaries, and we validated the effectiveness of the indicator by comparing the discriminative indicators of eight group sets. In general, the proposed DSDL model can effectively extract discriminative features and shared features simultaneously of multi-class fabric textures.
更多查看译文
关键词
dictionary learning,texture characterization,texture reconstruction,discriminative indicator
AI 理解论文
溯源树
样例
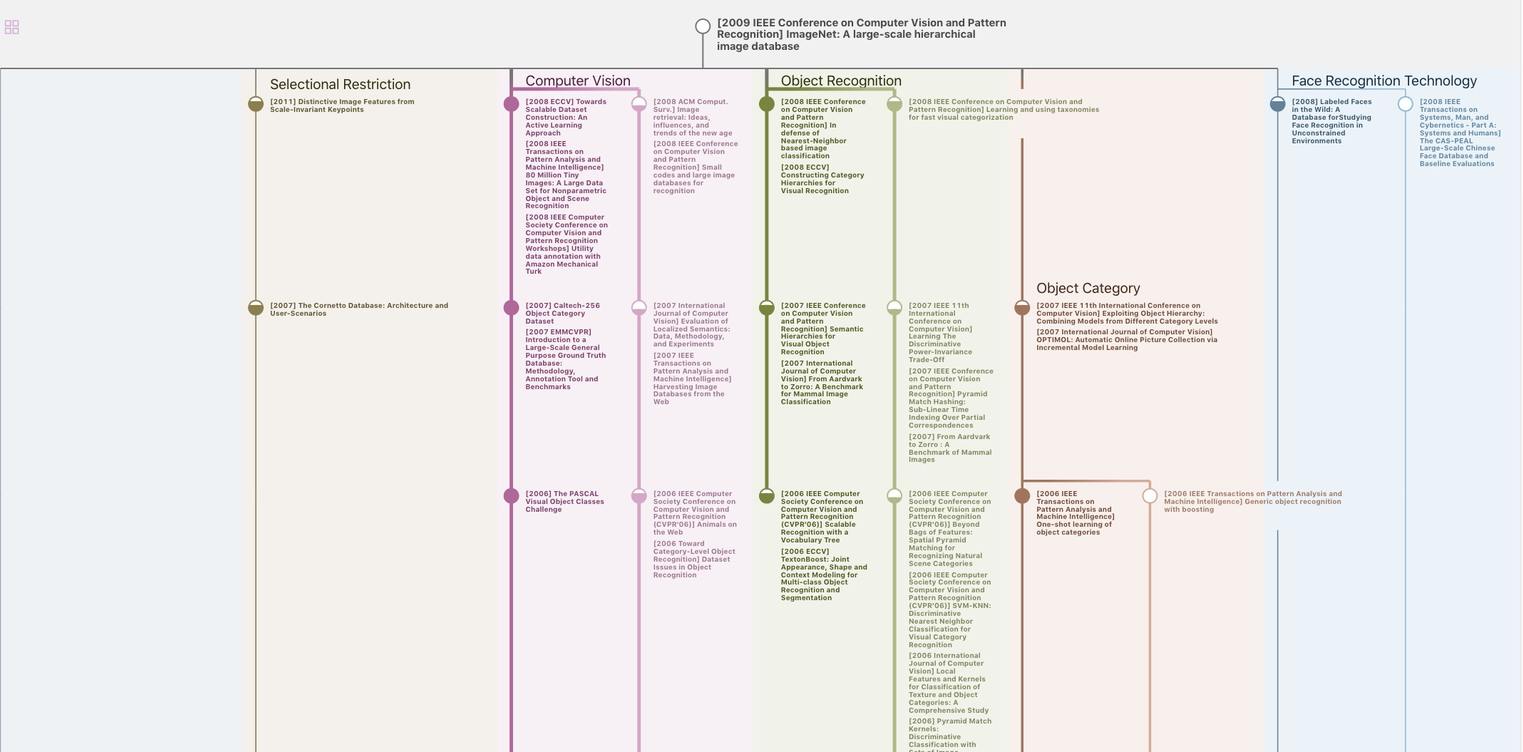
生成溯源树,研究论文发展脉络
Chat Paper
正在生成论文摘要