Healthcare and anomaly detection: using machine learning to predict anomalies in heart rate data
AI & SOCIETY(2020)
摘要
The application of machine learning algorithms to healthcare data can enhance patient care while also reducing healthcare worker cognitive load. These algorithms can be used to detect anomalous physiological readings, potentially leading to expedited emergency response or new knowledge about the development of a health condition. However, while there has been much research conducted in assessing the performance of anomaly detection algorithms on well-known public datasets, there is less conceptual comparison across unsupervised and supervised performance on physiological data. Moreover, while heart rate data are both ubiquitous and noninvasive, there has been little research specifically for anomaly detection of this type of data. Considering that heart rate data are indicative of both potential health complications and an individual’s physical activity, this is a rich source of largely overlooked data. To this end, we employed and evaluated five machine learning algorithms, two of which are unsupervised and the remaining three supervised, in their ability to detect anomalies in heart rate data. These algorithms were then evaluated on real heart rate data. Findings supported the effectiveness of local outlier factor and random forests algorithms in the task of heart rate anomaly detection, as each model generalized well from their training on simulated heart rate data to real world heart rate data. Furthermore, results support that simulated data can help configure algorithms to a degree of performance when real labeled data are not available and that this type of learning might be especially helpful in initial deployment of a system without prior data.
更多查看译文
关键词
Anomaly detection,Healthcare,Heart rate,Machine learning
AI 理解论文
溯源树
样例
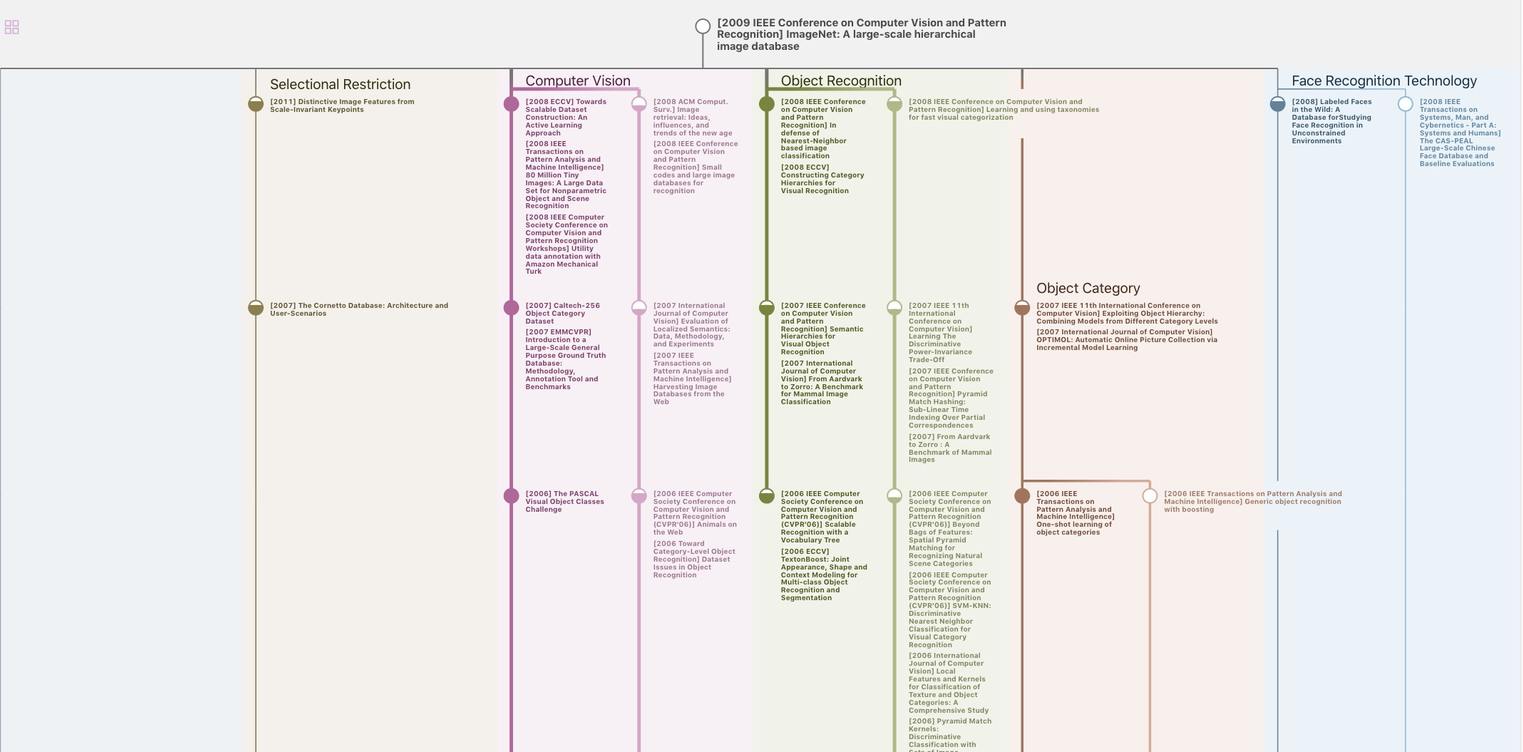
生成溯源树,研究论文发展脉络
Chat Paper
正在生成论文摘要