Connecting the Dots: Hypotheses Generation by Leveraging Semantic Shifts.
PAKDD (2)(2020)
摘要
Literature-based Discovery (LBD) (a.k.a. Hypotheses Generation) is a systematic knowledge discovery process that elicit novel inferences about previously unknown scientific knowledge by rationally connecting complementary and non-interactive literature. Prompt identification of such novel knowledge is beneficial not only for researchers but also for various other stakeholders such as universities, funding bodies and academic publishers. Almost all the prior LBD research suffer from two major limitations. Firstly, the over-reliance of domain-dependent resources which restrict the models’ applicability to certain domains/problems. In this regard, we propose a generalisable LBD model that supports both cross-domain and cross-lingual knowledge discovery. The second persistent research deficiency is the mere focus of static snapshot of the corpus (i.e. ignoring the temporal evolution of topics) to detect the new knowledge. However, the knowledge in scientific literature changes dynamically and thus relying merely on static snapshot limits the model’s ability in capturing semantically meaningful connections. As a result, we propose a novel temporal model that captures semantic change of topics using diachronic word embeddings to unravel more accurate connections. The model was evaluated using the largest available literature repository to demonstrate the efficiency of the proposed cues towards recommending novel knowledge.
更多查看译文
关键词
hypotheses generation
AI 理解论文
溯源树
样例
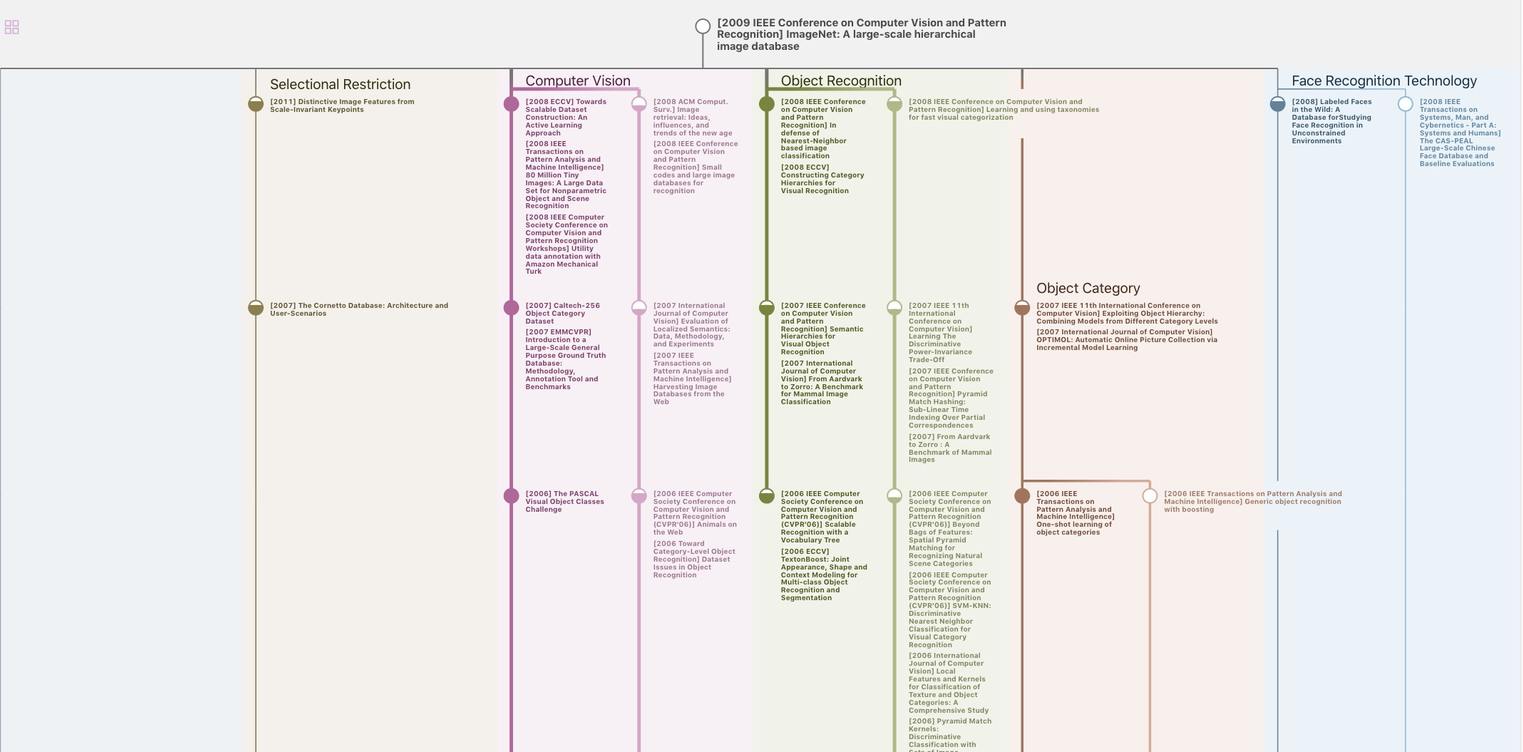
生成溯源树,研究论文发展脉络
Chat Paper
正在生成论文摘要