A Soft Affiliation Graph Model for Scalable Overlapping Community Detection
european conference on principles of data mining and knowledge discovery(2020)
摘要
We propose an overlapping community model based on the Affiliation Graph Model (AGM), that exhibits the pluralistic homophily property that the probability of a link between nodes increases with increasing number of shared communities. We take inspiration from the Mixed Membership Stochastic Blockmodel (MMSB), in proposing an edgewise community affiliation. This allows decoupling of community affiliations between nodes, opening the way to scalable inference. We show that our model corresponds to an AGM with soft community affiliations and develop a scalable algorithm based on a Stochastic Gradient Riemannian Langevin Dynamics (SGRLD) sampler. Empirical results show that the model can scale to network sizes that are beyond the capabilities of MCMC samplers of the standard AGM. We achieve comparable performance in terms of accuracy and run-time efficiency to scalable MMSB samplers.
更多查看译文
关键词
scalable overlapping community detection,soft affiliation graph model
AI 理解论文
溯源树
样例
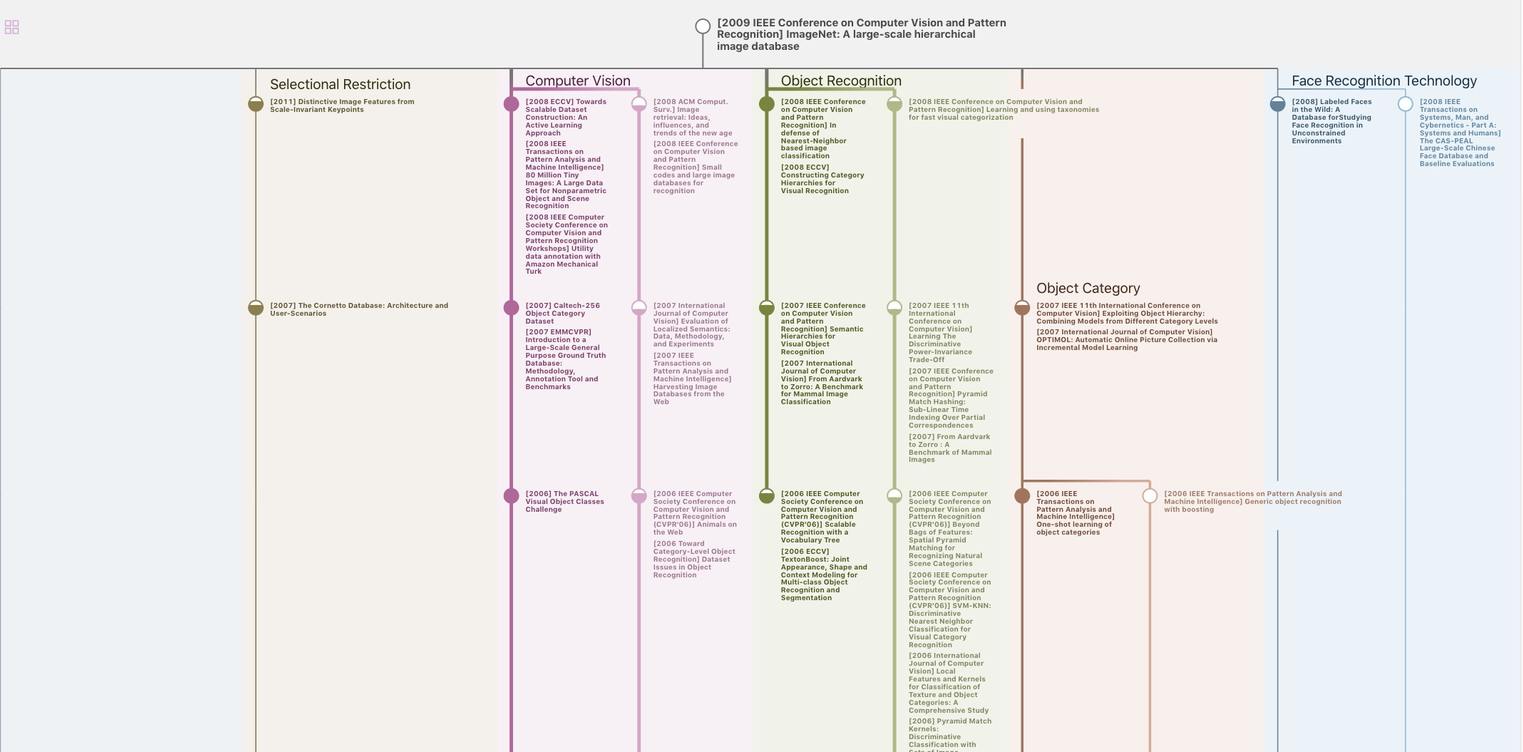
生成溯源树,研究论文发展脉络
Chat Paper
正在生成论文摘要