Deep Learning Techniques for Improving Estimations of Key Parameters for Efficient Flight Planning
2019 IEEE/AIAA 38th Digital Avionics Systems Conference (DASC)(2019)
摘要
This paper applies machine learning techniques to improve flight efficiency. Specifically, we focus on two distinct problems: uncertainties in aircraft performance models and uncertainties in wind. In this sense, this paper proposed methodologies to improve baseline models for fuel flow and wind estimations are via operational data. We utilize Base of Aircraft Data (BADA) 4 as baseline for aircraft performance model. Historical Global Forecast System (GFS) predictions are utilized as baseline estimations for u and v components of wind. As for the operational data, Quick Access Recorder (QAR) trajectory footprints of a narrow body and a wide body aircraft, which include actual recorded fuel flow from engines and measured wind speed and direction, are used. State-of-the-art deep learning algorithms are deployed to map baseline estimations for fuel flow and wind to their ground truths. Proper input parameters to have the best estimation results and be compatible with the ground-based flight planning systems are derived through extensive feature engineering. Comparison of the aircraft performance models with real flight data shows that precise estimation of fuel flow with mean absolute errors on a range of %0.1 - %0.7 can be achieved across all the flight modes. Results also show that we can achieve considerable reduction in wind uncertainty both from a mean error and variance sense. For short haul flights, the standard deviations of forecast errors in u and v components are reduced from 6.25 and 8.38 knots to 1.37 and 1.81 knots, respectively. The same reduction is from 11.02 and 10.89 knots to 4.88 and 4.76 knots in the long haul flights.
更多查看译文
关键词
deep learning techniques,flight efficiency,aircraft performance model,wind estimations,Aircraft Data 4,wide body aircraft,measured wind speed,ground-based flight planning systems,flight data,flight modes,wind uncertainty,short haul flights,long haul flights,quick access recorder trajectory footprints,historical global forecast system predictions
AI 理解论文
溯源树
样例
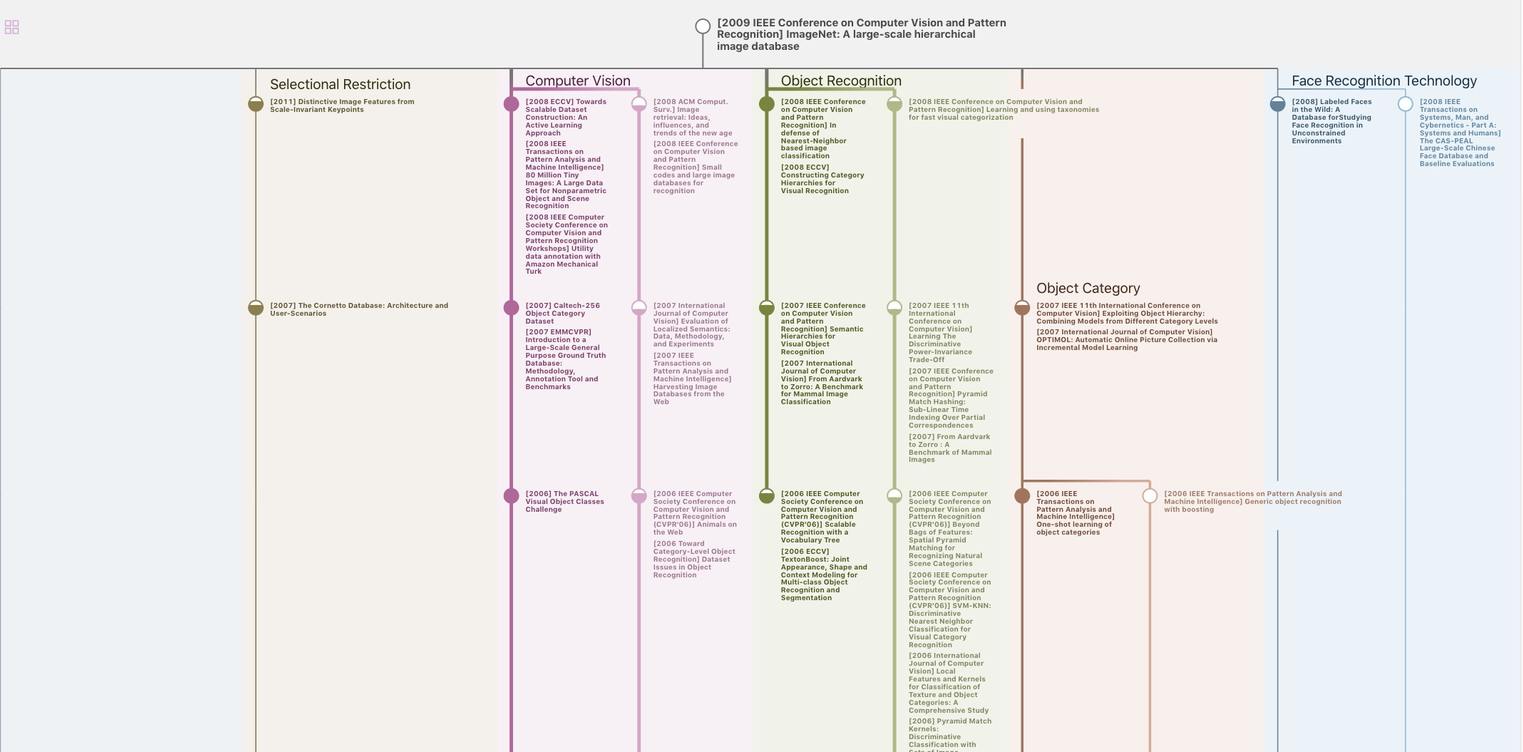
生成溯源树,研究论文发展脉络
Chat Paper
正在生成论文摘要