CrackGAN: Pavement Crack Detection Using Partially Accurate Ground Truths Based on Generative Adversarial Learning
IEEE Transactions on Intelligent Transportation Systems(2021)
摘要
Fully convolutional network is a powerful tool for per-pixel semantic segmentation/detection. However, it is problematic when coping with crack detection using partially accurate ground truths (GTs): the network may easily converge to the status that treats all the pixels as background (BG) and still achieves a very good loss, named “All Black” phenomenon, due to the unavailability of accurate GTs and the data imbalance. To tackle this problem, we propose crack-patch-only (CPO) supervised generative adversarial learning for end-to-end training, which forces the network to always produce crack-GT images while reserves both crack and BG-image translation abilities by feeding a larger-size crack image into an asymmetric U-shape generator to overcome the “All Black” issue. The proposed approach is validated using four crack datasets; and achieves state-of-the-art performance comparing with that of the recently published works in efficiency and accuracy.
更多查看译文
关键词
Pavement crack detection,fully convolutional networks,generative adversarial learning,partially accurate ground truths
AI 理解论文
溯源树
样例
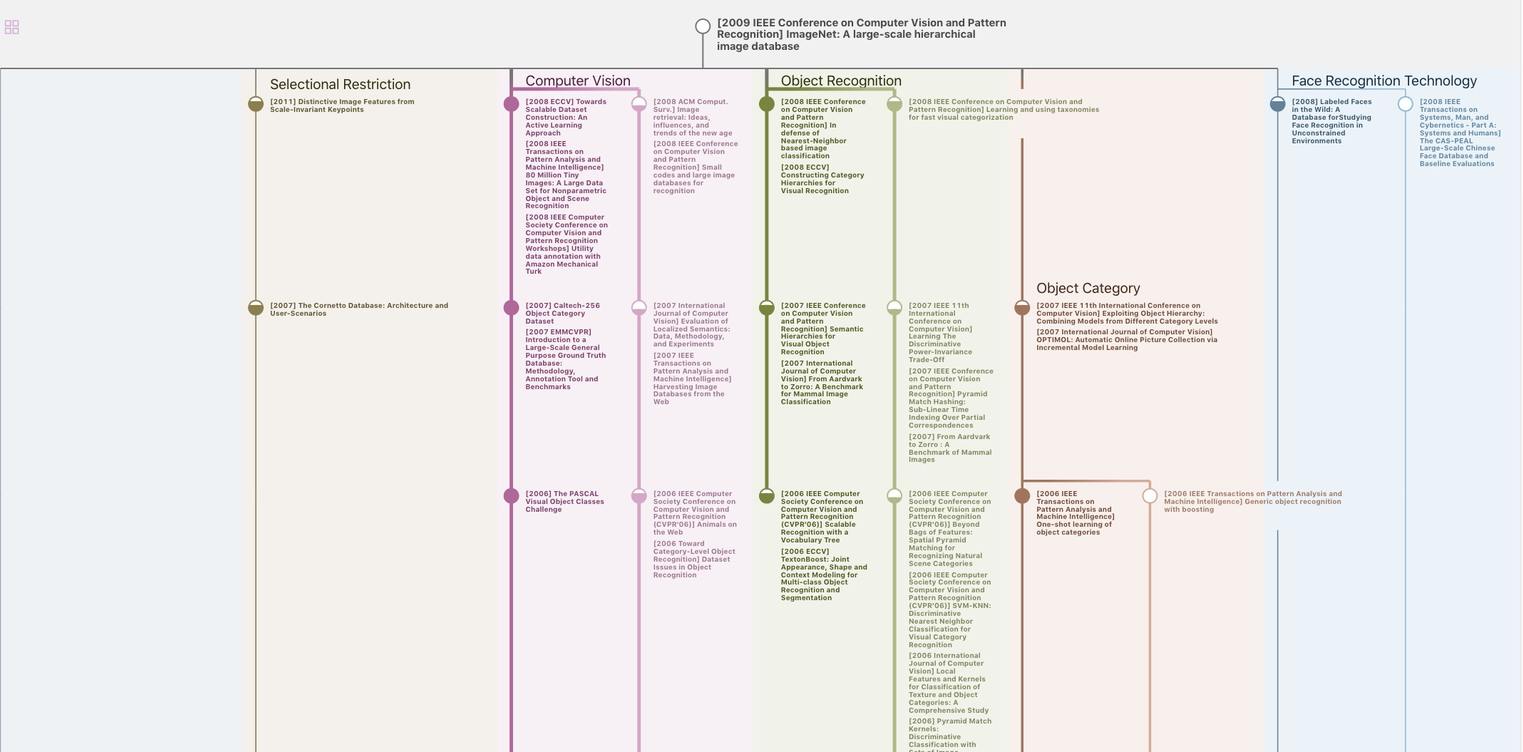
生成溯源树,研究论文发展脉络
Chat Paper
正在生成论文摘要