High temporal resolution prediction of street-level PM2.5 and NOx concentrations using machine learning approach
Journal of Cleaner Production(2020)
摘要
Accurate and high temporal resolution predictions of fine particulate matter (PM2.5) and nitrogen oxides (NOx) concentrations are crucial for pollution control, air pollutant exposure and epidemiological studies. This study aimed to develop machine learning-based models for predicting hourly street-level PM2.5 and NOx concentrations at three roadside stations in Hong Kong. We comprehensively evaluated and compared the performance of six common machine learning algorithms (MLAs) including Random Forest (RF), Boosted Regression Trees (BRT), Support Vector Machine (SVM), Extreme Gradient Boosting (XGBoost), Generalized Additive Model (GAM), and Cubist and hence applied the most suitable MLAs to apportion the contributions from emission and non-emission factors to hourly street-level PM2.5 and NOx concentrations. The results show that RF outperformed other MLAs with ten-fold cross validation (CV) R2 values higher than 0.81 and 0.62 for PM2.5 and NOx predictions, respectively. BRT, XGBoost and Cubist presented comparable predictive performances, with CV R2 of 0.79–0.83 (for PM2.5 predictions) and 0.59–0.71 (for NOx predictions). SVM and GAM had worse predictions than other MLAs. The external validation R2 values for RF and BRT models were more than 0.62 and 0.51 for PM2.5 and NOx concentration predictions, respectively. Non-emission factors contributed 84% and 65% to the predictions of street-level PM2.5 and NOx concentrations, respectively. Non-local pollution and temperature were the major non-emission factors, whereas private cars were the major emission contributor. This study highlights the capability of MLAs to produce high temporal resolution air pollution predictions, which can supplement traditional methods (e.g., land use regression) in generating accurate and high-temporal-resolution estimations of air pollution concentration.
更多查看译文
关键词
Roadside air pollution,PM2.5,NOx,Prediction model,Machine learning
AI 理解论文
溯源树
样例
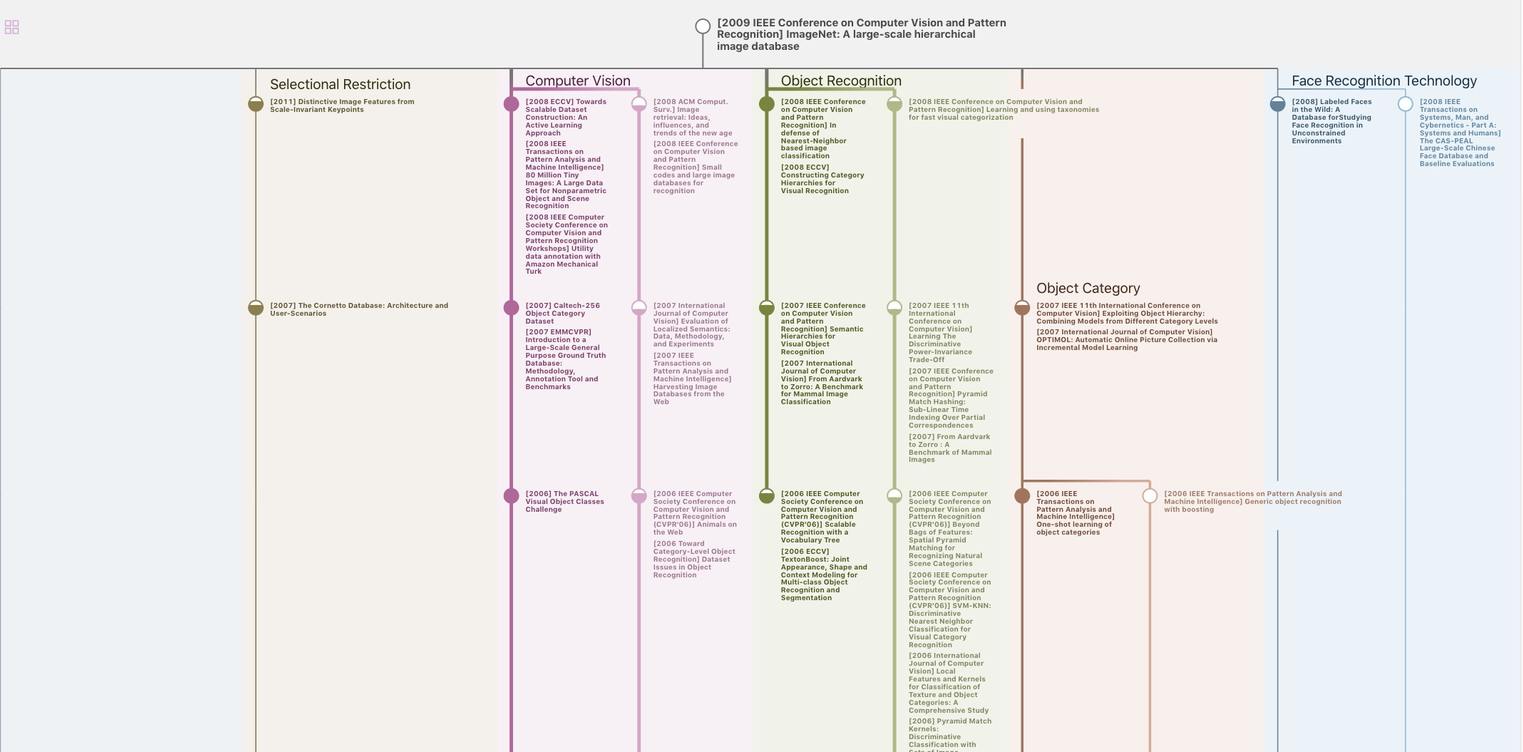
生成溯源树,研究论文发展脉络
Chat Paper
正在生成论文摘要