A New Multiple-Distribution GAN Model to Solve Complexity in End-to-End Chromosome Karyotyping
COMPLEXITY(2020)
摘要
With significant development of Internet of medical things (IoMT) and cloud-fog-edge computing, medical industry is now involving medical big data to improve quality of service in patient care. Karyotyping refers classifying human chromosomes. However, performing karyotyping task generally requires domain expertise in cytogenetics, long-period experience for high accuracy, and considerable manual efforts. An end-to-end chromosome karyotype analysis system is proposed over medical big data to automatically and accurately perform chromosome related tasks of detection, segmentation, and classification. Facing image data generated and collected by means of edge computing, we firstly utilize visual feature to generate chromosome candidates with Extremal Regions (ER) technology. Due to severe occlusion and cross overlapping, we utilize ring radius transform to cluster pixels with same property to approximate chromosome shapes. To solve the problem of unbalanced and small dataset by covering diverse data patterns, we proposed multidistributed generated advertising network (MD-GAN) to perform data enhancement by generating additional training samples. Afterwards, we fine-tune CNN for chromosome classification task by involving generated and sufficient training images. Through experiments in self-collected datasets, the proposed method achieves high accuracy in tasks of chromosome detection, segmentation, and classification. Moreover, experimental results prove that MD-GAN-based data enhancement contributes to classification results of CNN to a certain extent.
更多查看译文
AI 理解论文
溯源树
样例
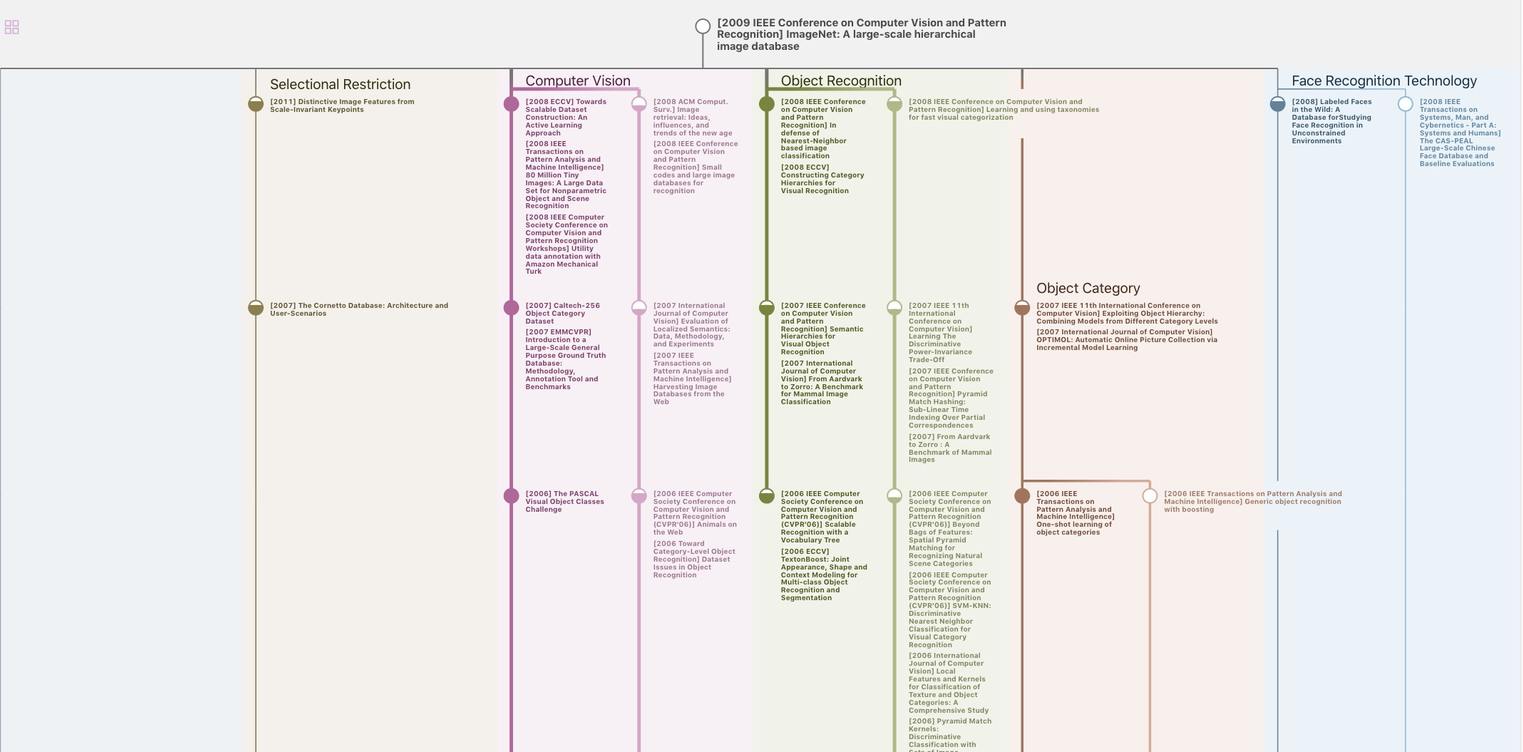
生成溯源树,研究论文发展脉络
Chat Paper
正在生成论文摘要