Lattice LSTM for Chinese Sentence Representation
IEEE-ACM TRANSACTIONS ON AUDIO SPEECH AND LANGUAGE PROCESSING(2020)
摘要
Words provide a useful source of information for Chinese NLP, and word segmentation has been taken as a pre-processing step for most downstream tasks. For many NLP tasks, however, word segmentation can introduce noise and lead to error propagation. The rise of neural representation learning models allows sentence-level semantic information to be collected from characters directly. As a result, it is an empirical question whether a fully character-based model should be used instead of first performing word segmentation. We investigate a neural representation that simultaneously encodes character and word information without the need for segmentation. In particular, candidate words are found in a sentence by matching with a pre-defined lexicon. A lattice structured LSTM is used to encode the resulting word-character lattice, where gate vectors are used to control information flow through words, so that the more useful words can be automatically identified by end-to-end training. We compare the performance of the resulting lattice LSTM and baseline sequence LSTM structures over both character sequences and automatically segmented word sequences. Results on NER show that the character-word lattice model can significantly improve the performance. In addition, as a general sentence representation architecture, character-word lattice LSTM can also be used for learning contextualized representations. To this end, we compare lattice LSTM structure with its sequential LSTM counterpart, namely ELMo. Results show that our lattice version of ELMo gives better language modeling performances. On Chinese POS-tagging, chunking and syntactic parsing tasks, the resulting contextualized Chinese embeddings also give better performance than ELMo trained on the same data.
更多查看译文
关键词
Lattices,Task analysis,Bridges,Urban areas,Rivers,Training,Labeling,Lattice LSTM,NER,language modeling,contextualize representation
AI 理解论文
溯源树
样例
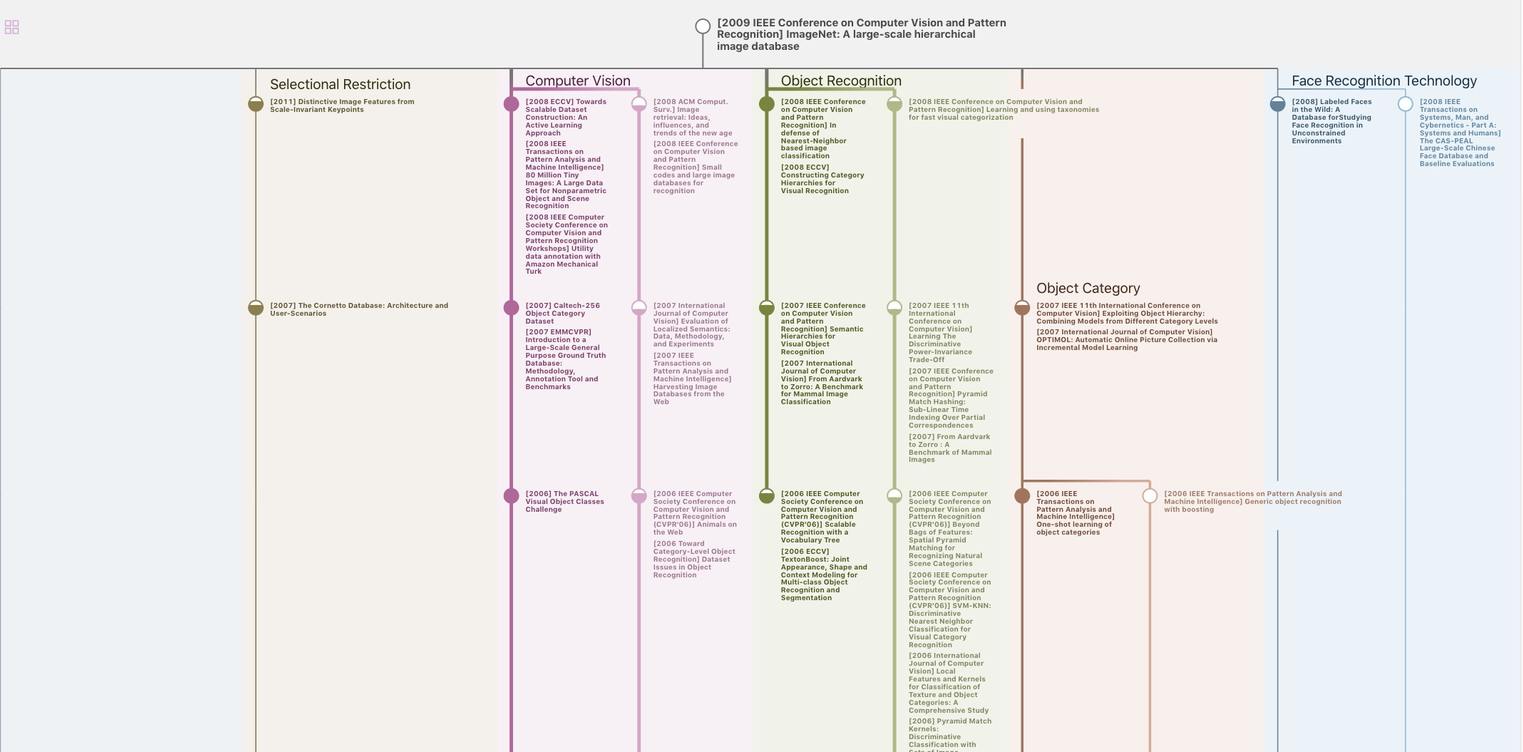
生成溯源树,研究论文发展脉络
Chat Paper
正在生成论文摘要