On the Interpretability of Machine Learning Models and Experimental Feature Selection in Case of Multicollinear Data
Electronics(2020)
摘要
In the field of machine learning, a considerable amount of research is involved in the interpretability of models and their decisions. The interpretability contradicts the model quality. Random Forests are among the best quality technologies of machine learning, but their operation is of “black box” character. Among the quantifiable approaches to the model interpretation, there are measures of association of predictors and response. In case of the Random Forests, this approach usually consists of calculating the model’s feature importances. Known methods, including the built-in one, are less suitable in settings with strong multicollinearity of features. Therefore, we propose an experimental approach to the feature selection task, a greedy forward feature selection method with least-trees-used criterion. It yields a set of most informative features that can be used in a machine learning (ML) training process with similar prediction quality as the original feature set. We verify the results of the proposed method on two known datasets, one with small feature multicollinearity and another with large feature multicollinearity. The proposed method also allows for a domain expert help with selecting among equally important features, which is known as the human-in-the-loop approach.
更多查看译文
关键词
interpretable machine learning,feature multicollinearity,random forests,feature selection,feature importance,greedy feature selection
AI 理解论文
溯源树
样例
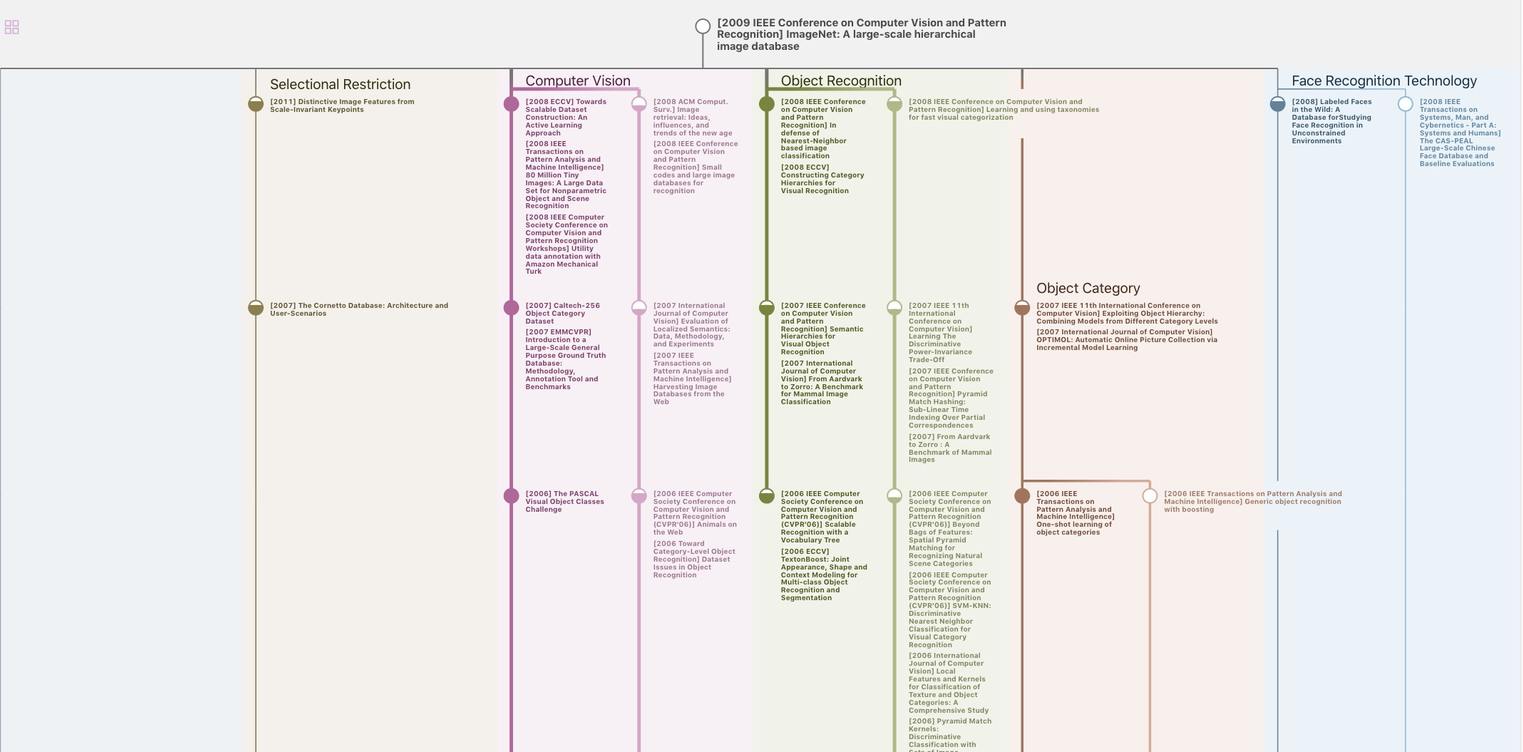
生成溯源树,研究论文发展脉络
Chat Paper
正在生成论文摘要