Development of a speed invariant deep learning model with application to condition monitoring of rotating machinery
JOURNAL OF INTELLIGENT MANUFACTURING(2020)
摘要
The application of cutting-edge technologies such as AI, smart sensors, and IoT in factories is revolutionizing the manufacturing industry. This emerging trend, so called smart manufacturing, is a collection of various technologies that support decision-making in real-time in the presence of changing conditions in manufacturing activities; this may advance manufacturing competitiveness and sustainability. As a factory becomes highly automated, physical asset management comes to be a critical part of an operational life-cycle. Maintenance is one area where the collection of technologies may be applied to enhance operational reliability using a machine condition monitoring system. Data-driven models have been extensively applied to machine condition data to build a fault detection system. Most existing studies on fault detection were developed under a fixed set of operating conditions and tested with data obtained from that set of conditions. Therefore, variability in a model’s performance from data obtained from different operating settings is not well reported. There have been limited studies considering changing operational conditions in a data-driven model. For practical applications, a model must identify a targeted fault under variable operational conditions. With this in mind, the goal of this paper is to study invariance of model to changing speed via a deep learning method, which can detect a mechanical imbalance, i.e., targeted fault, under varying speed settings. To study the speed invariance, experimental data obtained from a motor test-bed are processed, and time-series data and time–frequency data are applied to long short-term memory and convolutional neural network, respectively, to evaluate their performance.
更多查看译文
关键词
Maintenance, Long short-term memory, Convolutional neural network, Machine condition monitoring, Mechanical imbalance
AI 理解论文
溯源树
样例
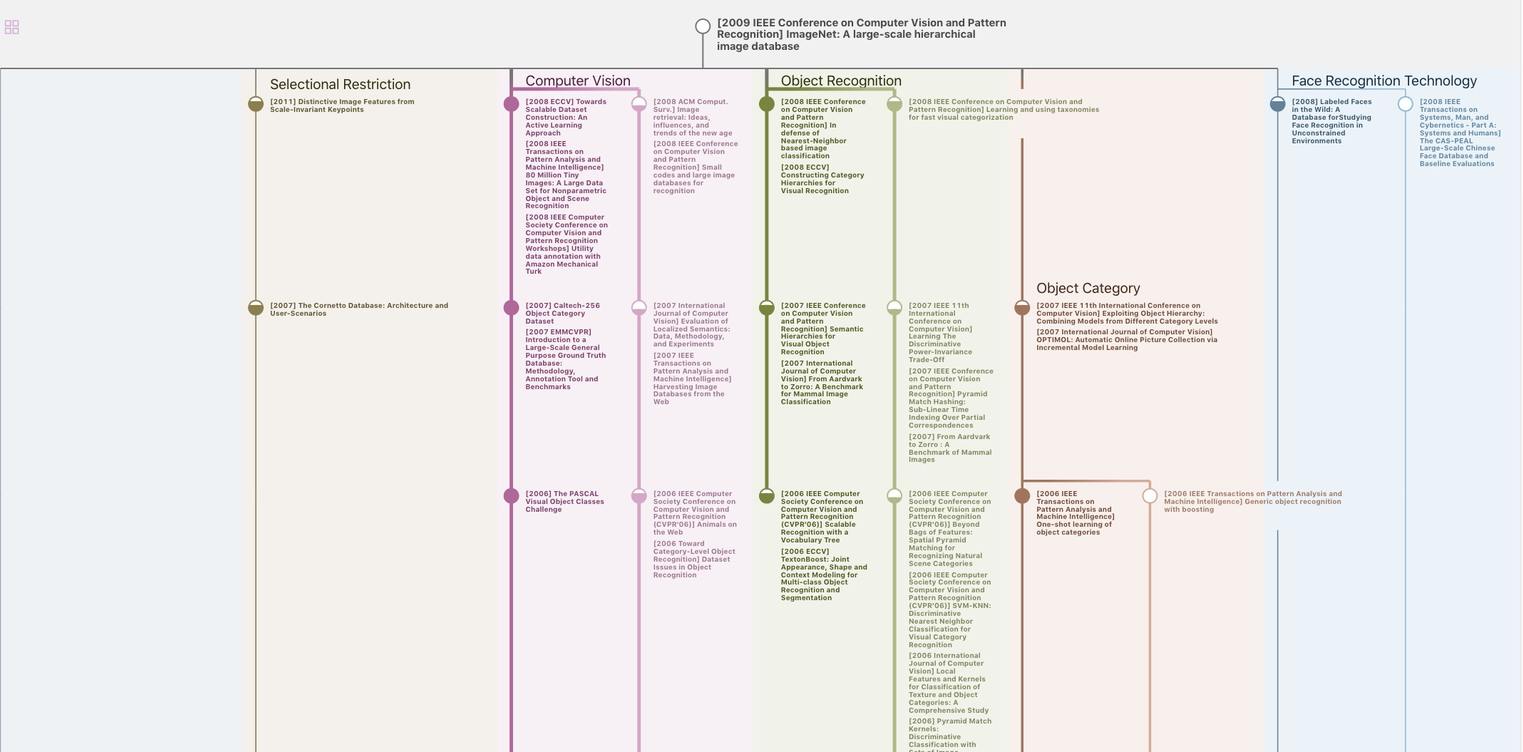
生成溯源树,研究论文发展脉络
Chat Paper
正在生成论文摘要