High-Performance Machine Learning for Large-Scale Data Classification considering Class Imbalance
Periodicals(2020)
摘要
AbstractCurrently, data classification is one of the most important ways to analysis data. However, along with the development of data collection, transmission, and storage technologies, the scale of the data has been sharply increased. Additionally, due to multiple classes and imbalanced data distribution in the dataset, the class imbalance issue is also gradually highlighted. The traditional machine learning algorithms lack of abilities for handling the aforementioned issues so that the classification efficiency and precision may be significantly impacted. Therefore, this paper presents an improved artificial neural network in enabling the high-performance classification for the imbalanced large volume data. Firstly, the Borderline-SMOTE (synthetic minority oversampling technique) algorithm is employed to balance the training dataset, which potentially aims at improving the training of the back propagation neural network (BPNN), and then, zero-mean, batch-normalization, and rectified linear unit (ReLU) are further employed to optimize the input layer and hidden layers of BPNN. At last, the ensemble learning-based parallelization of the improved BPNN is implemented using the Hadoop framework. Positive conclusions can be summarized according to the experimental results. Benefitting from Borderline-SMOTE, the imbalanced training dataset can be balanced, which improves the training performance and the classification accuracy. The improvements for the input layer and hidden layer also enhance the training performances in terms of convergence. The parallelization and the ensemble learning techniques enable BPNN to implement the high-performance large-scale data classification. The experimental results show the effectiveness of the presented classification algorithm.
更多查看译文
关键词
classification,machine learning,large-scale large-scale,high-performance
AI 理解论文
溯源树
样例
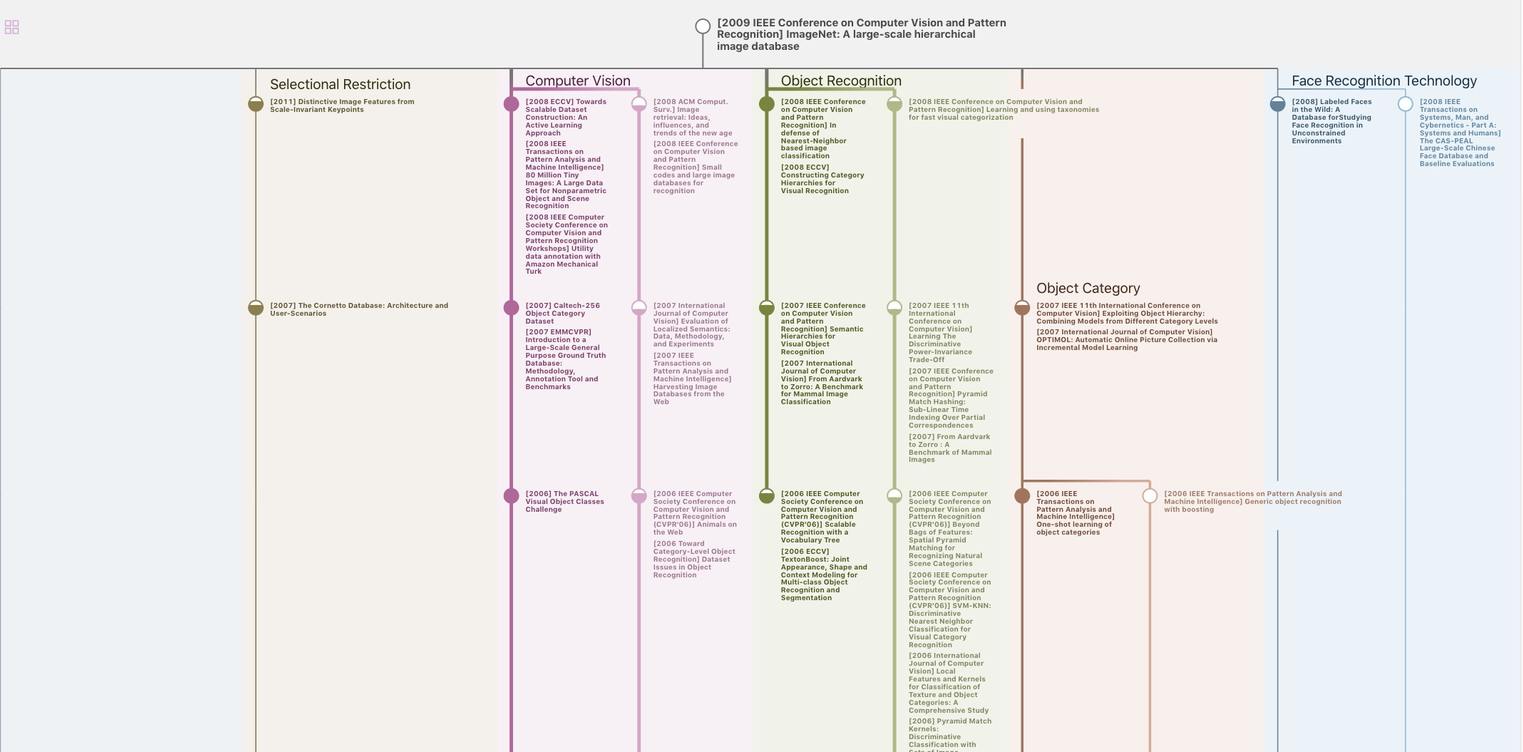
生成溯源树,研究论文发展脉络
Chat Paper
正在生成论文摘要