Contour-Aware Polyp Segmentation In Colonoscopy Images Using Detailed Upsamling Encoder-Decoder Networks
IEEE ACCESS(2020)
摘要
Colorectal cancer has become one of the most common cause of cancer mortality worldwide, with a five-year survival rate of over 50%. Additionally, the potential of some common polyp types to progress to colorectal cancer is considered high. Colonoscopy is the most common method for finding and removing polyps. However, during colonoscopy, a significant number of polyps is missed as a result of human error mistakes. Thus, this study was primarily motivated by the need to obtain an early and accurate diagnosis of polyps detected in colonoscopy images. In this paper, we propose a new polyp segmentation method based on an architecture of multi-model deep encoder-decoder networks called MED-Net. Not only does this architecture obtain multi-level contextual information by extracting discriminative features at different effective fields-of-view and multiple image scales, it also can substantially do upsample more correctly to produce better prediction. It is also able to capture more accurate polyp boundaries by using multi-scale effective decoders. Moreover, we also present a complementary strategy for improving the method's segmentation performance based on a combination of a boundary-aware data augmentation method and an effective weighted loss function. The purpose of this strategy is to allow our deep learning network to sequentially focus on poorly defined polyp boundaries, which are caused by the non-specular transition zone between the polyp and non-polyp regions. To provide a general view of the proposed method, our network was trained and evaluated on four well-known dataset CVC-ColonDB, CVC-ClinicDB, ASU-Mayo Clinic Colonoscopy Video Database, and ETIS-LaribPolypDB. Our results show that our MED-Net significantly outperforms state-of-the-art methods.
更多查看译文
关键词
Cancer, Image segmentation, Colonoscopy, Feature extraction, Training, Decoding, Colon, Polyp segmentation, hierarchical collaborative representation-based classification, local ternary patterns, deep convolutional neural network, colorectal segmentation
AI 理解论文
溯源树
样例
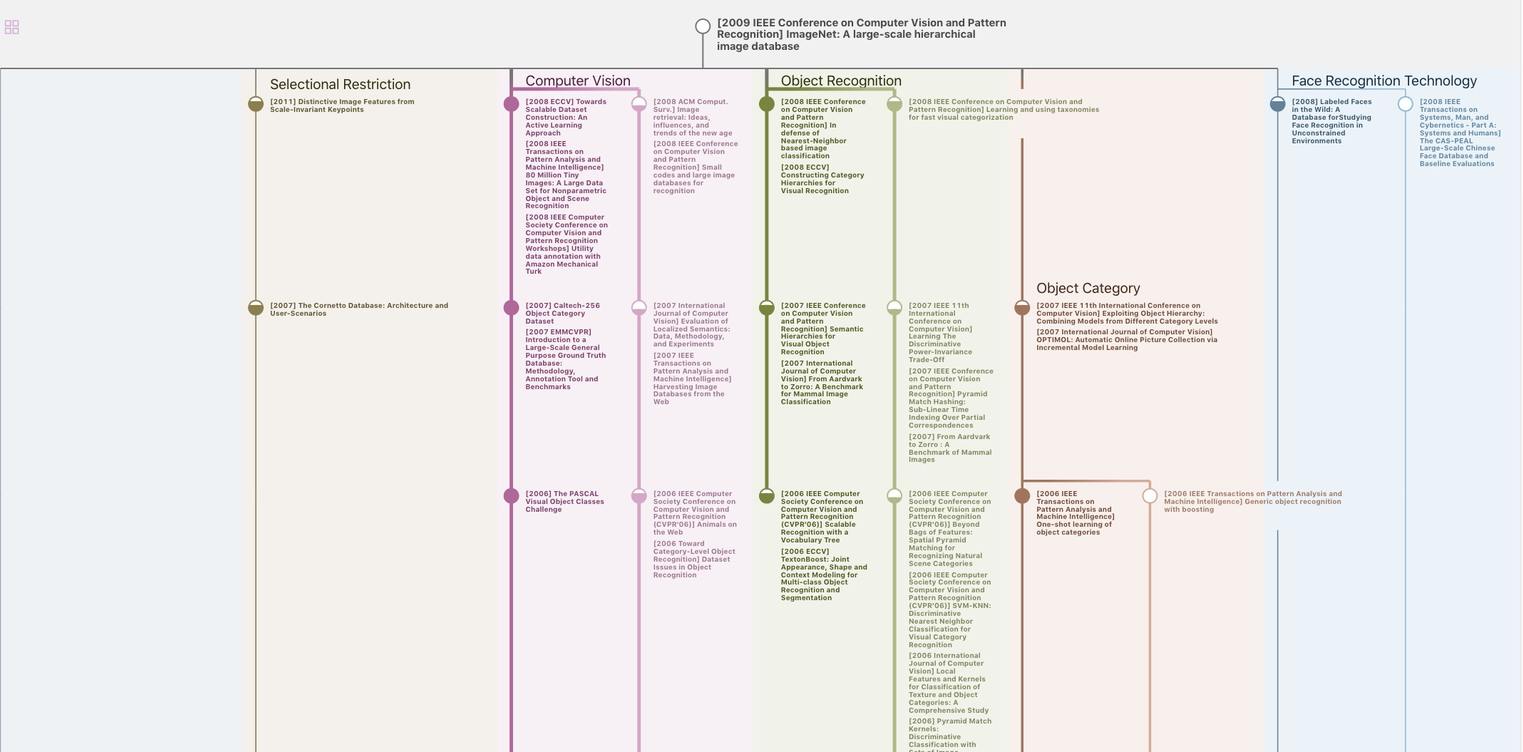
生成溯源树,研究论文发展脉络
Chat Paper
正在生成论文摘要