A big data-driven dynamic estimation model of relief supplies demand in urban flood disaster
International Journal of Disaster Risk Reduction(2020)
摘要
The dynamic relief supplies estimation for urban flood disaster still remains an important and challenging topic in emergency response. Traditional relief supplies estimation mainly depends on static census data rather than dynamic spatio-temporal population distribution, which may easily lead to a serious imbalance between supply and demand for relief resources. The emergence of big data originating from web mapping service, social media, crowdsourcing system and other methods provides alternative data sources to understand the dynamic distribution of urban population, which can help accurately estimating the relief supplies demand when urban flood occurs. This paper proposes a big data-driven dynamic estimation model of relief supplies demand, which merges the dynamic population distribution from Baidu big data and Multilayer Perceptron (MLP) neural network so as to improve the accuracy and timeliness. Taking Wuhan as an example, a specific day in summer flooding period was chosen to dynamically predict three kinds of relief materials. The results indicate that the proposed model is more feasible to estimate the relief supplies because it is integrated with MLP neural network trained by national-wide historical flood disaster cases and Baidu big data. The utility of Baidu big data for flood-affected population statistics has been shown to be more comprehensive than that of the traditional demographic methods. Thorough analysis exhibits the superiority of our proposed model with respect to accuracy and dynamics. This allows our proposed model to be more widely applied in pre- and post-disaster operations activities carried out by government and humanitarian aid organizations.
更多查看译文
关键词
Flood disaster,Relief supplies estimation,Big data,Multilayer perceptron neural network,Dynamic population distribution
AI 理解论文
溯源树
样例
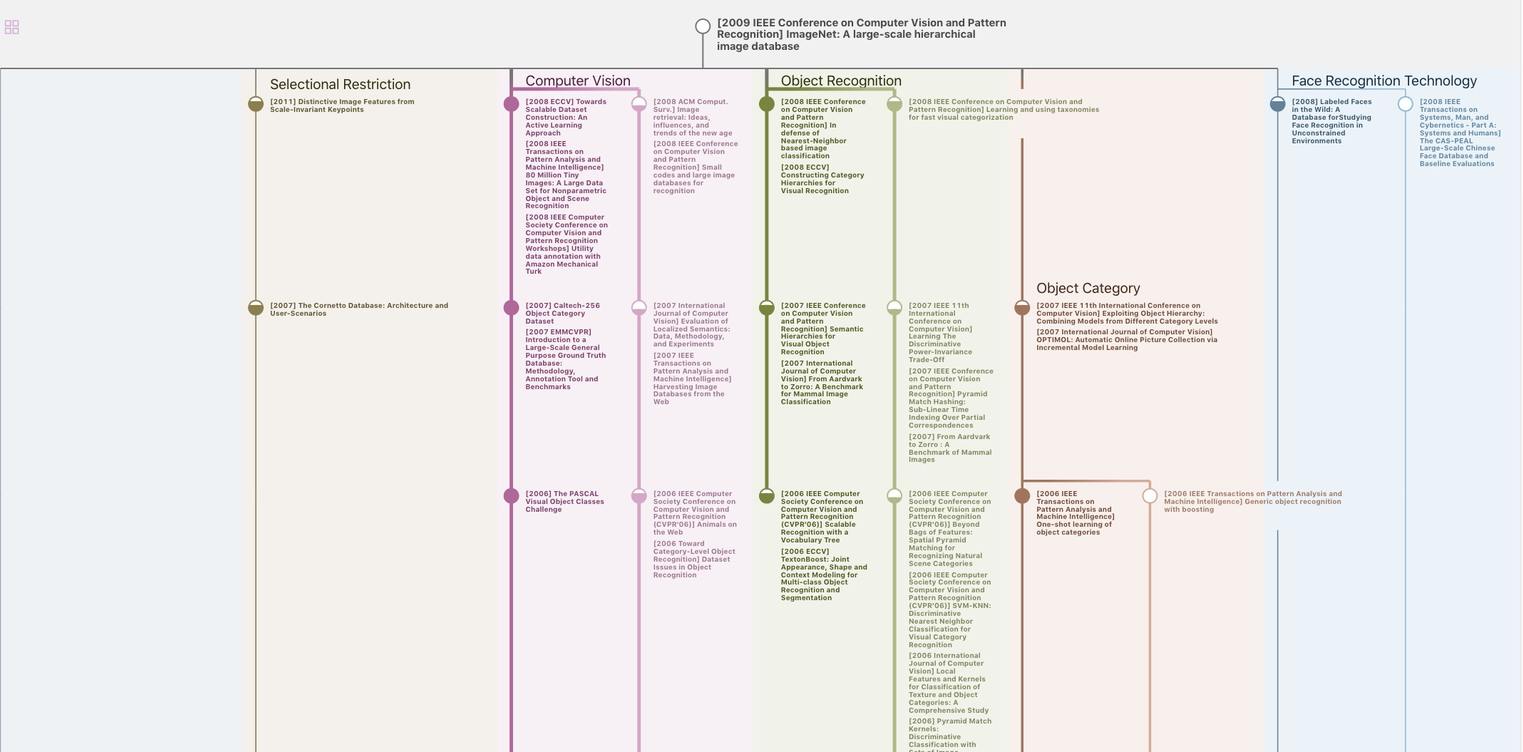
生成溯源树,研究论文发展脉络
Chat Paper
正在生成论文摘要