Predicting Lncrna-Disease Association Based On Extreme Gradient Boosting
PROCEEDINGS OF 2020 10TH INTERNATIONAL CONFERENCE ON BIOSCIENCE, BIOCHEMISTRY AND BIOINFORMATICS (ICBBB 2020)(2020)
摘要
There is increasing evidence that long non-coding RNAs (lncRNAs) play an important role in many significant biological processes. Associations' detection between lncRNAs and human diseases by computational models is beneficial to the identification of biomarkers and the discovery of drugs for the diagnosis, treatment, and prognosis of human diseases. In this study, we propose a method called PrLDA (Predicting LncRNA-Disease Association based on extreme gradient boosting) for predicting potential lncRNA-disease associations based on eXtreme Gradient Boosting (XGBoost). Firstly, we compute semantic similarity of diseases and lncRNA sequence similarity. Then, we extracte feature vectors by concatenating these similarities horizontally. At last, the feature matrix after dimension reduction is used as the input for XGBoost and we get the score about the lncRNA association with a specific disease. Computational results indicate that our method can predict lncRNA-disease associations with higher accuracy compared with previous methods. Furthermore, case study shows that our method can effectively predict candidate lncRNAs for breast cancer, with 80% of the top 10 predictions are confirmed by experiments. Therefore, PrLDA is a useful computational method for lncRNA-disease association prediction.
更多查看译文
关键词
lncRNA-disease associations, Prediction model, Feature matrix, Principal component analysis, Extreme gradient boosting
AI 理解论文
溯源树
样例
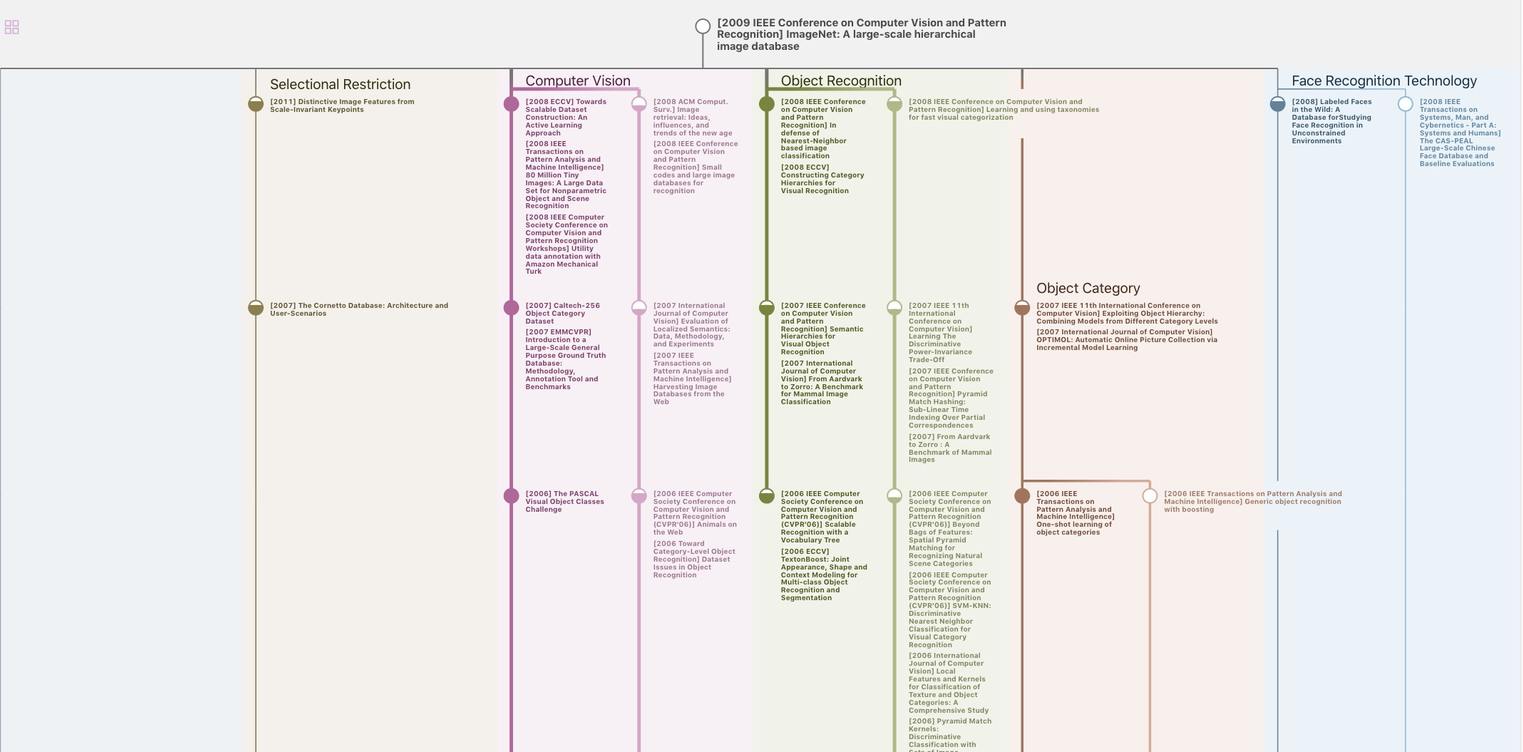
生成溯源树,研究论文发展脉络
Chat Paper
正在生成论文摘要