Robust Principal Component Analysis with Projection Learning for Image Classification
Journal of modern optics(2020)
摘要
In this paper, we propose a robust subspace learning method, based on RPCA, named Robust Principal Component Analysis with Projection Learning (RPCAPL), which further improves the performance of feature extraction by projecting data samples into a suitable subspace. For Subspace Learning (SL) methods in clustering and classification tasks, it is also critical to construct an appropriate graph for discovering the intrinsic structure of the data. For this reason, we add a graph Laplacian matrix to the RPCAPL model for preserving the local geometric relationships between data samples and name the improved model as RPCAGPL, which takes all samples as nodes in the graph and treats affinity between pairs of connected samples as weighted edges. The RPCAGPL can not only globally capture the low-rank subspace structure of the data in the original space, but also locally preserve the neighbor relationship between the data samples.
更多查看译文
关键词
Subspace learning,low-rank representation,projection learning,graph embedding,sparse constraint
AI 理解论文
溯源树
样例
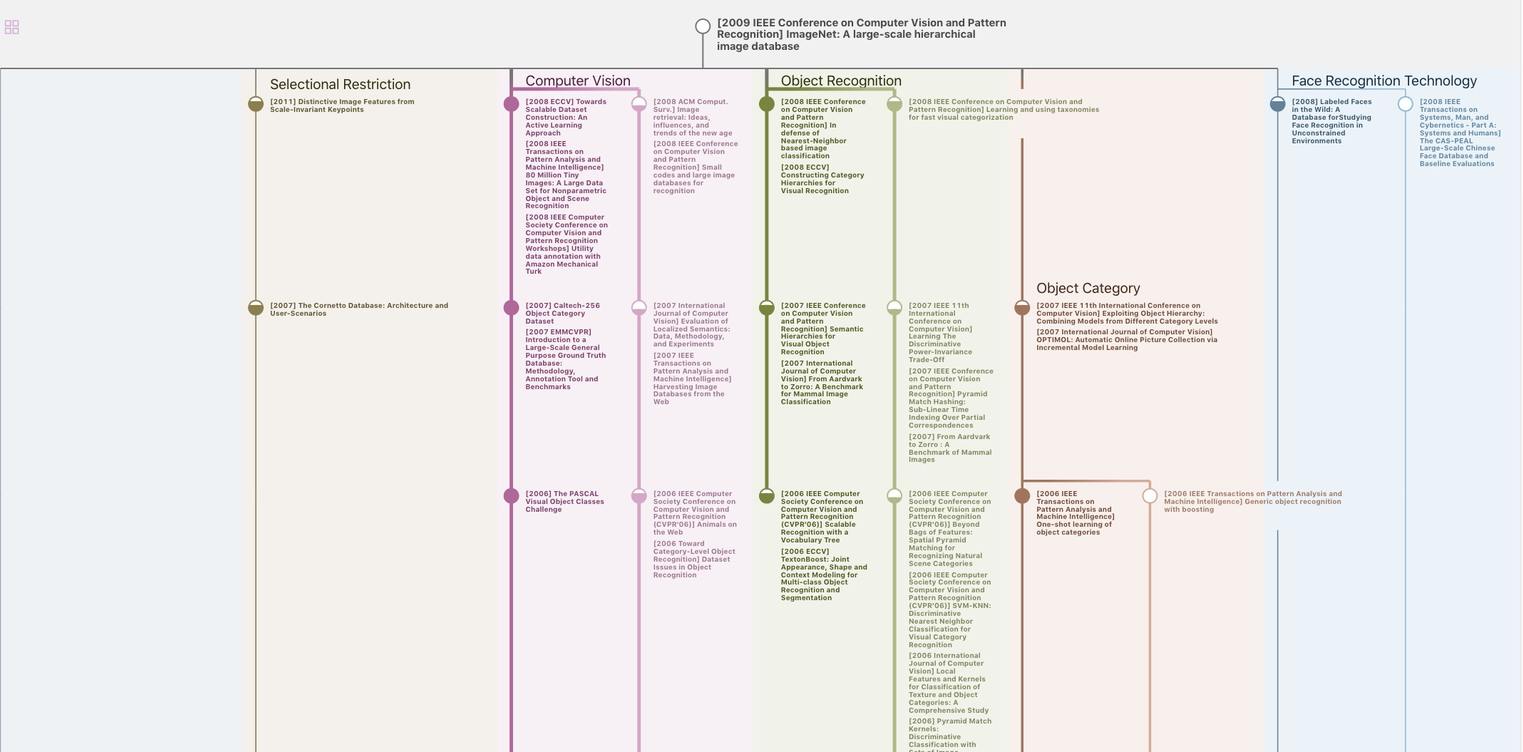
生成溯源树,研究论文发展脉络
Chat Paper
正在生成论文摘要