Tensor-Based Low-Rank and Sparse Prior Information Constraints for Hyperspectral Image Denoising
IEEE access(2020)
摘要
Hyperspectral data have been widely used in various fields due to its rich spectral and spatial information in recent years. Yet, hyperspectral images are always tainted by a variety of mixed noises. These noises seriously limit the accuracy of subsequent applications. To remove noise, this paper, based on low-rank tensor decomposition, combined with non-local self-similar prior information, proposes a tensor-based non-local low-rank denoising model, where non-local self-similarity uses mainly spatial correlation while low-rank tensor decomposition method uses mainly spectral correlation between bands. Traditional tensor-based methods are commonly NP-hard to compute and are sensitive to sparse noise. However, the method proposed in this paper can separate efficiently the low-rank clean image from Gaussian noise and sparse noise (pulses, deadlines, stripes, speckle, etc.) by using a new tensor singular value decomposition (T-SVD) and tensor nuclear norm (TNN). The NP-hard task was also achieved well by the alternating direction multiplier method. Due to the full use of spectral and spatial information of the data, Gaussian noise and sparse noise can be effectively removed. The effectiveness of our algorithm was verified through experiments using simulated and real data.
更多查看译文
关键词
Hyperspectral image denoising,non-local self-similar,low-rank tensor decomposition,sparse representation
AI 理解论文
溯源树
样例
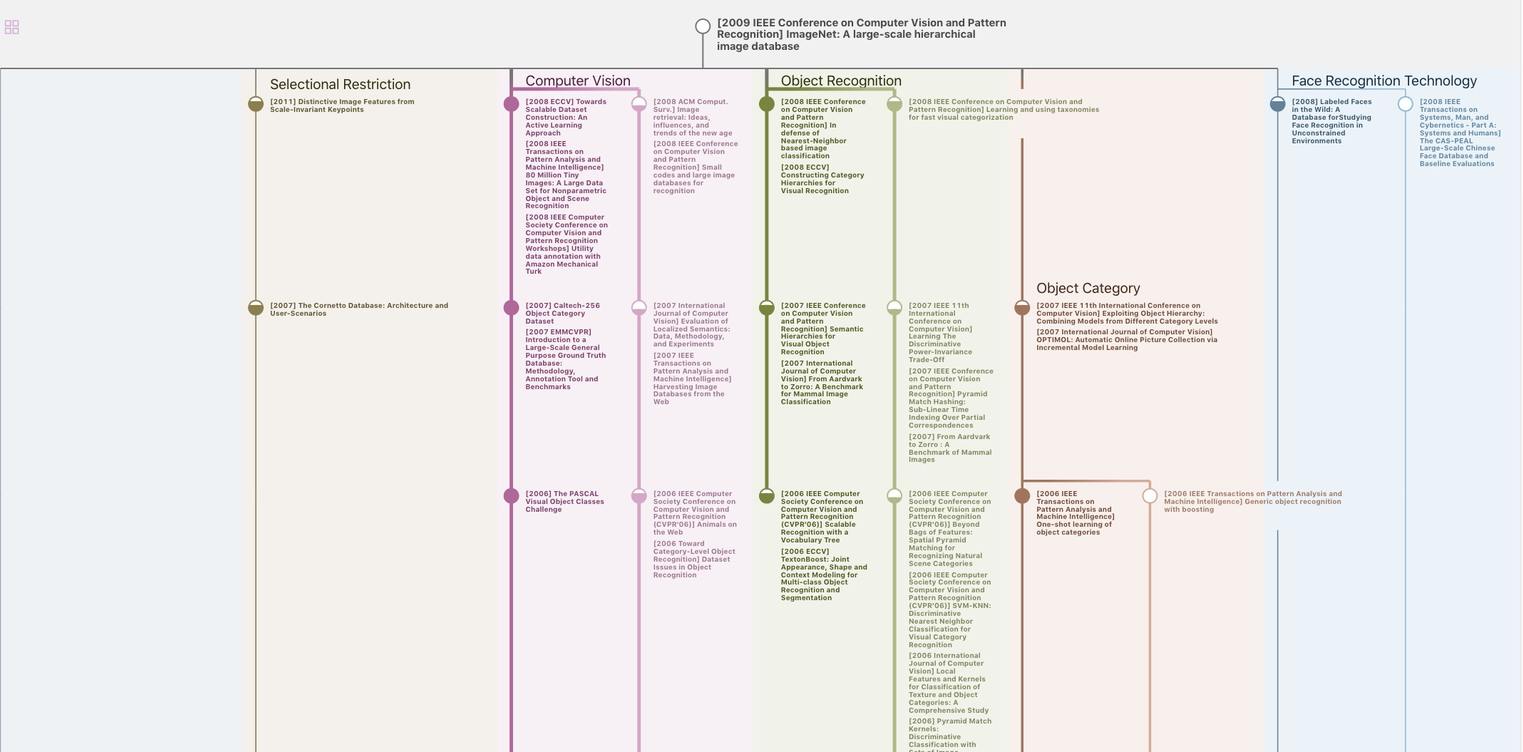
生成溯源树,研究论文发展脉络
Chat Paper
正在生成论文摘要