Ul-Cnn: An Ultra-Lightweight Convolutional Neural Network Aiming At Flash-Based Computing-In-Memory Architecture For Pedestrian Recognition
JOURNAL OF CIRCUITS SYSTEMS AND COMPUTERS(2021)
摘要
Pedestrian recognition has achieved the state-of-the-art performance due to the progress of recent convolutional neural network (CNN). However, mainstream CNN models are too complicated to emerging Computing-In-Memory (CIM) architectures for hardware implementation, because enormous parameters and massive intermediate processing results may incur severe "memory bottleneck". This paper proposed a design methodology of Parameter Substitution with Nodes Compensation (PSNC) to significantly reduce parameters of CNN model without inference accuracy degradation. Based on the PSNC methodology, an ultra-lightweight convolutional neural network (UL-CNN) was designed. The UL-CNN model is a specially optimized convolutional neural network aiming at a flash-based CIM architecture (Cony-Flash) and to apply for recognizing person. The implementation result of running UL-CNN on Cony-Flash shows that the inference accuracy is up to 94.7%. Compared to LeNet-5, on the premise of the similar operations and accuracy, the amounts of UL-CNN's parameters are less than 37% of LeNet-5 at the same dataset benchmark. Such parameter reduction can dramatically speed up the training process and economize on-chip storage overhead, as well as save the power consumption of the memory access. With the aid of UL-CNN, the Cony-Flash architecture can provide the best energy efficiency compared to other platforms (CPU, GPU, FPGA, etc.), which consumes only 2.2x 105J to complete pedestrian recognition for one frame.
更多查看译文
关键词
Ultra-lightweight convolutional neural network, parameters substitution with nodes compensation, flash-based computing-in-memory, pedestrian recognition
AI 理解论文
溯源树
样例
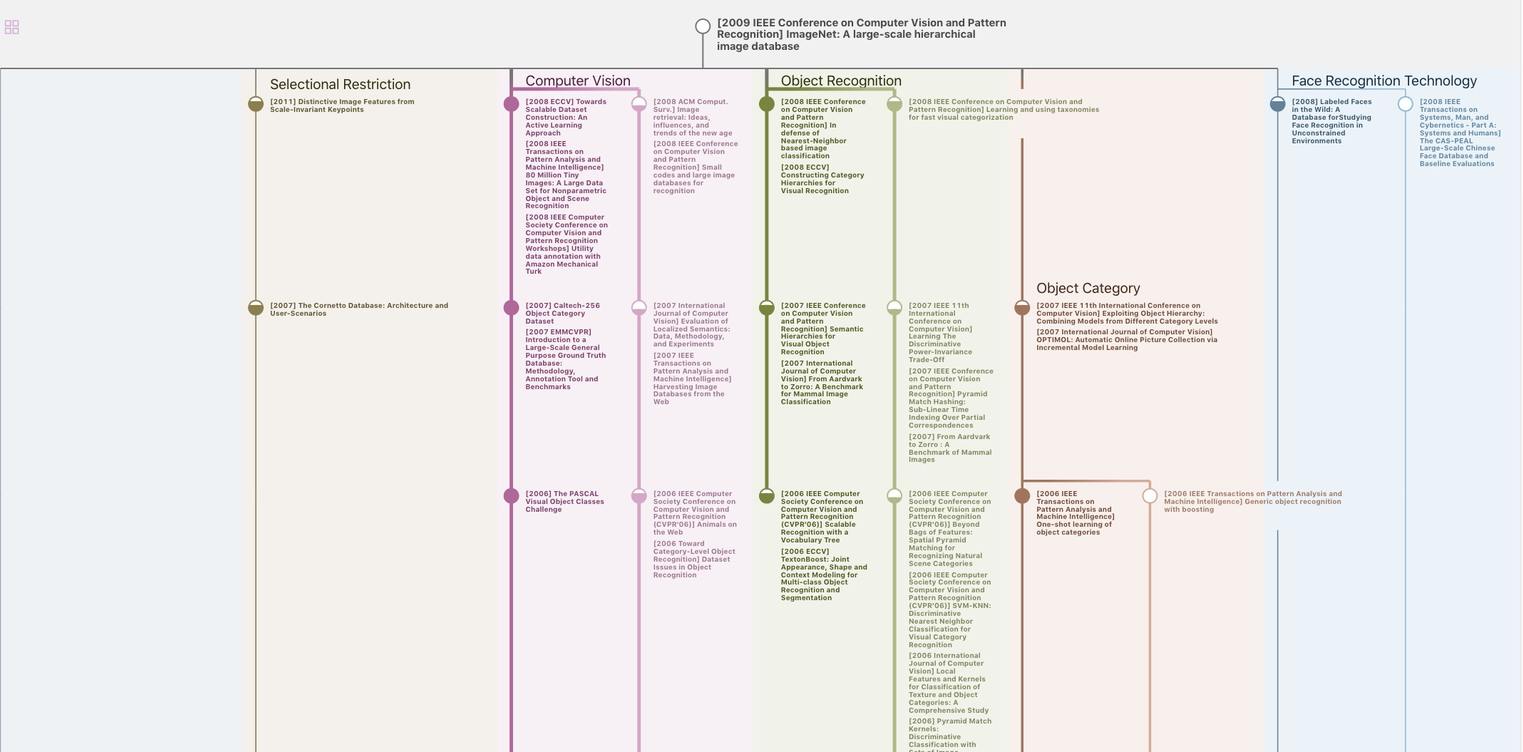
生成溯源树,研究论文发展脉络
Chat Paper
正在生成论文摘要