Application of Machine Learning Algorithm and Remote-sensed Data to Estimate Forest Gross Primary Production at Multi-sites Level
Journal of remote sensing(2019)
摘要
Forest covers 30% of the Earth's land area and plays an important role in global carbon flux through its ability to store much greater amounts of carbon than other terrestrial ecosystems. The Gross Primary Production (GPP) represents the productivity of forest ecosystems according to climate change and its effect on the phenology, health, and carbon cycle. In this study, we estimated the daily GPP for a forest ecosystem using remote-sensed data from Moderate Resolution Imaging Spectroradiometer (MODIS) and machine learning algorithms Support Vector Machine (SVM). MODIS products were employed to train the SVM model from 75% to 80% data of the total study period and validated using eddy covariance measurement (EC) data at the six flux tower sites. We also compare the GPP derived from EC and MODIS (MYD17). The MODIS products made use of two data sets: one for Processed MODIS that included calculated by combined products (e.g., Vapor Pressure Deficit), another one for Unprocessed MODIS that used MODIS products without any combined calculation. Statistical analyses, including Pearson correlation coefficient (R), mean squared error (MSE), and root mean square error (RMSE) were used to evaluate the outcomes of the model. In general, the SVM model trained by the Unprocessed MODIS (R = 0.77 - 0.94, p < 0.001) derived from the multi-sites outperformed those trained at a single-site (R =0.75 -0.95, p <0.001). These results show better performance trained by the data including various events and suggest the possibility of using remote-sensed data without complex processes to estimate GPP such as non-stationary ecological processes.
更多查看译文
关键词
Gross Primary Production, Machine learning, MODIS, Remote-sensed data
AI 理解论文
溯源树
样例
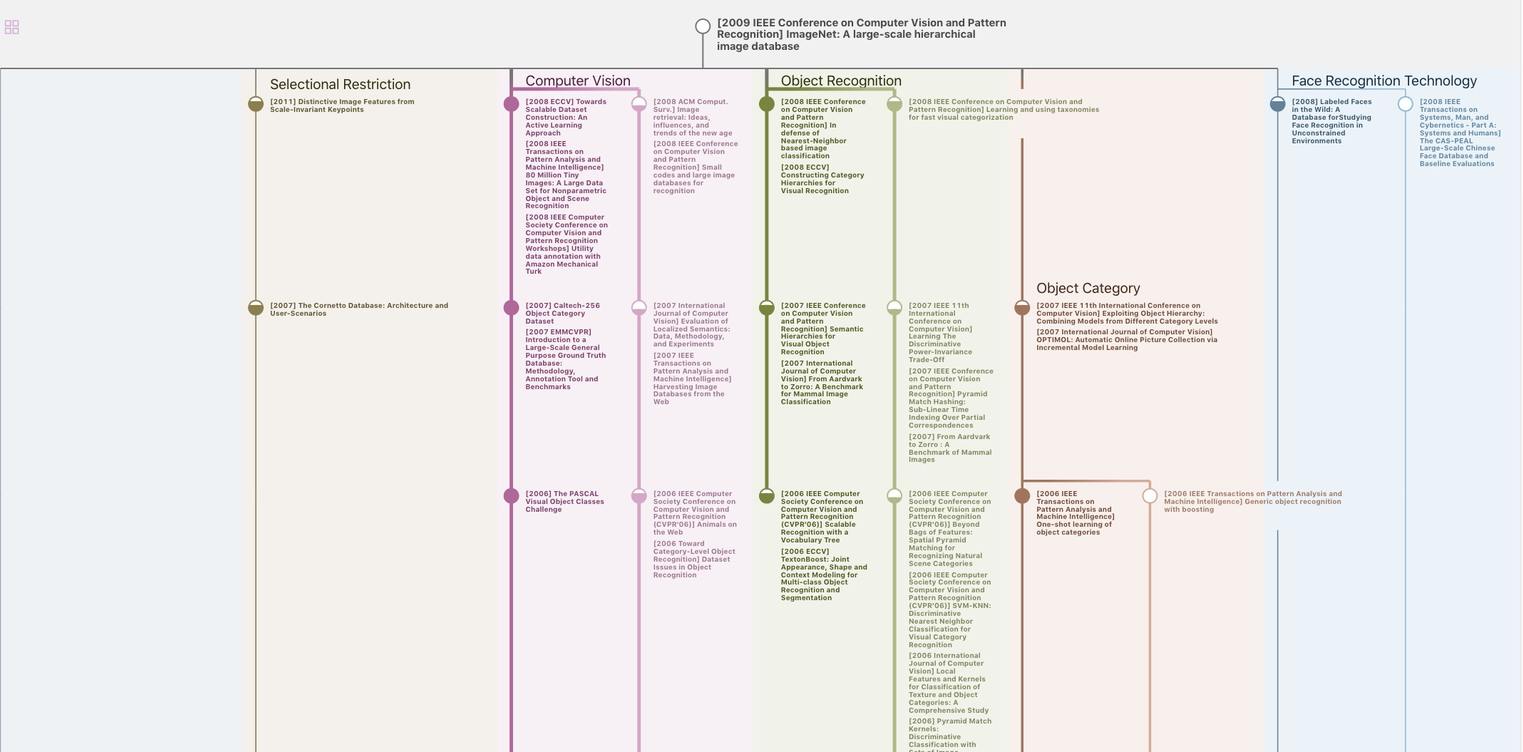
生成溯源树,研究论文发展脉络
Chat Paper
正在生成论文摘要