Optimizing Extreme Learning Machines Using Chains Of Salps For Efficient Android Ransomware Detection
APPLIED SCIENCES-BASEL(2020)
摘要
Nowadays, smartphones are an essential part of people's lives and a sign of a contemporary world. Even that smartphones bring numerous facilities, but they form a wide gate into personal and financial information. In recent years, a substantial increasing rate of malicious efforts to attack smartphone vulnerabilities has been noticed. A serious common threat is the ransomware attack, which locks the system or users' data and demands a ransom for the purpose of decrypting or unlocking them. In this article, a framework based on metaheuristic and machine learning is proposed for the detection of Android ransomware. Raw sequences of the applications API calls and permissions were extracted to capture the ransomware pattern of behaviors and build the detection framework. Then, a hybrid of the Salp Swarm Algorithm (SSA) and Kernel Extreme Learning Machine (KELM) is modeled, where the SSA is used to search for the best subset of features and optimize the KELM hyperparameters. Meanwhile, the KELM algorithm is utilized for the identification and classification of the apps into benign or ransomware. The performance of the proposed (SSA-KELM) exhibits noteworthy advantages based on several evaluation measures, including accuracy, recall, true negative rate, precision, g-mean, and area under the curve of a value of 98%, and a ratio of 2% of false positive rate. In addition, it has a competitive convergence ability. Hence, the proposed SSA-KELM algorithm represents a promising approach for efficient ransomware detection.
更多查看译文
关键词
Kernel Extreme Learning Machine,Salp Swarm,swarm intelligence,Android,ransomware,machine classification
AI 理解论文
溯源树
样例
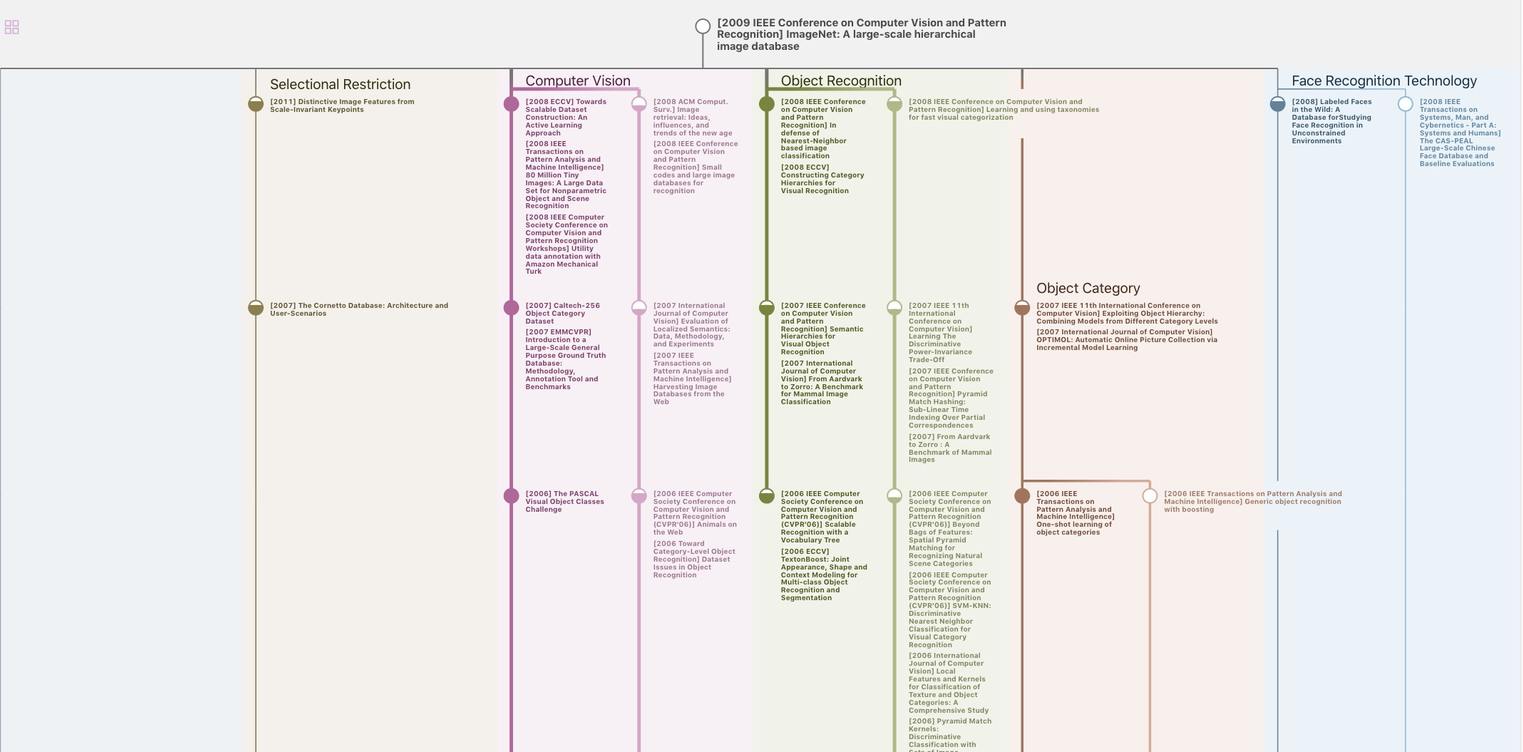
生成溯源树,研究论文发展脉络
Chat Paper
正在生成论文摘要