High-Throughput Screening of Hydrogen Evolution Reaction Catalysts in MXene Materials
JOURNAL OF PHYSICAL CHEMISTRY C(2020)
摘要
In this study, machine learning (ML) models combined with density functional theory (DFT) calculations and Gibbs free energy of hydrogen adsorption (Delta G(H*)) were employed to facilitate the high-throughput screening of hydrogen evolution reaction (HER) catalysts in various MXene materials. The predicted Delta G(H*) values show a high-level accuracy via the random forest algorithm by using only simple elemental features. A total of 299 MXene materials were screened by DFT calculations and four ML models (Elman Artificial Neural Networks, kernel ridge regression, support vector regression, and random forest regression algorithms). Using the simple elemental information, the random forest algorithm shows a high-level predicted accuracy with a low testing root-mean-square error of 0.27 eV. Os2B- and S-terminated Scn+1Nn (n = 1, 2, 3) were discovered to be the active catalysts as Delta G(H*) approaches zero with wide hydrogen coverages (theta from 1/9 to 4/9). S functional groups play a crucial role in regulating the HER performance due to the antibonding states which are full of electrons. Consequently, it weakens the adsorption of H* which is the key step of HER. In summary, the present work suggests that ML models are competitive tools in accelerating the screening of efficient HER catalysts.
更多查看译文
关键词
hydrogen evolution reaction catalysts,high-throughput
AI 理解论文
溯源树
样例
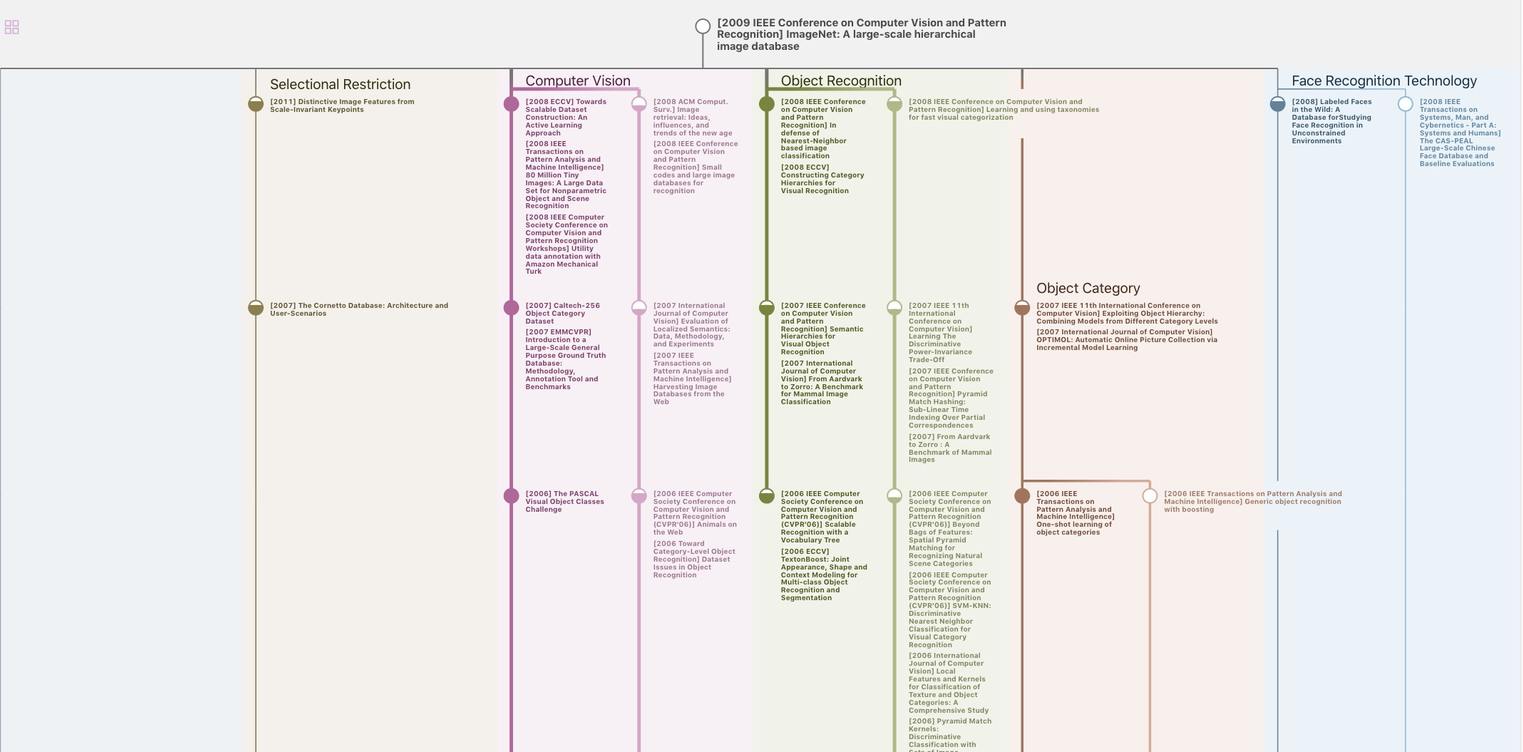
生成溯源树,研究论文发展脉络
Chat Paper
正在生成论文摘要