Neural Architecture Search for a Highly Efficient Network with Random Skip Connections
Applied sciences(2020)
摘要
Regarding the sequence learning of neural networks, there exists a problem of how to capture long-term dependencies and alleviate the gradient vanishing phenomenon. To manage this problem, we proposed a neural network with random connections via a scheme of a neural architecture search. First, a dense network was designed and trained to construct a search space, and then another network was generated by random sampling in the space, whose skip connections could transmit information directly over multiple periods and capture long-term dependencies more efficiently. Moreover, we devised a novel cell structure that required less memory and computational power than the structures of long short-term memories (LSTMs), and finally, we performed a special initialization scheme on the cell parameters, which could permit unhindered gradient propagation on the time axis at the beginning of training. In the experiments, we evaluated four sequential tasks: adding, copying, frequency discrimination, and image classification; we also adopted several state-of-the-art methods for comparison. The experimental results demonstrated that our proposed model achieved the best performance.
更多查看译文
关键词
neural architecture search,recurrent neural networks,temporal dependency,vanishing gradient
AI 理解论文
溯源树
样例
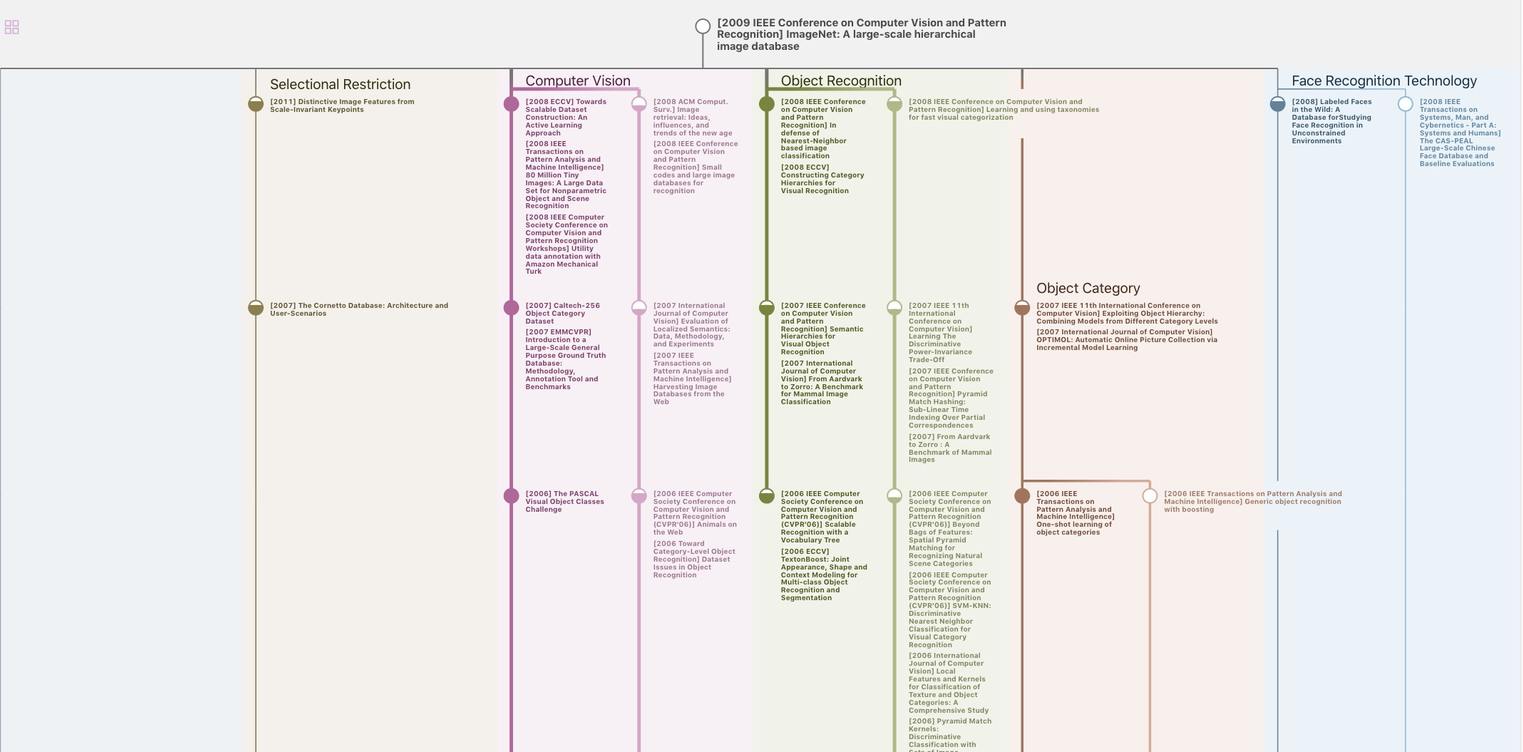
生成溯源树,研究论文发展脉络
Chat Paper
正在生成论文摘要