PDAWL: Profile-based Iterative Dynamic Adaptive WorkLoad Balance on Heterogeneous Architectures
JSSPP(2020)
摘要
While High Performance Computing systems are increasingly based on heterogeneous cores, their effectiveness depends on how well the scheduler can allocate workloads onto appropriate computing devices and how communication and computation can be overlapped. With different types of resources integrated into one system, the complexity of the scheduler correspondingly increases. Moreover, for applications with varying problem sizes on different heterogeneous resources, the optimal scheduling approach may vary accordingly. We thus present PDAWL, an event-driven profile-based Iterative Dynamic Adaptive Work-Load balance scheduling approach to dynamically and adaptively adjust workload to efficiently utilize heterogeneous resources. It combines online scheduling (DAWL), which can adaptively adjust workload based on available real time heterogeneous resources, with an offline machine learning (profile-based estimation model) which can build a device-specific communication computation estimation model. Our scheduling approach is tested on control-regular applications, Stencil kernel (based on a Jacobi Algorithm) and Sparse Matrix-Vector Multiplication (SpMV) in an event-driven runtime system. Experimental results show that PDAWL is either on-par or far outperforms whichever yields the best results (CPU or GPU).
更多查看译文
关键词
Heterogeneous many-core computing, Workload balance, Adaptive modeling, Ml assisted scheduling
AI 理解论文
溯源树
样例
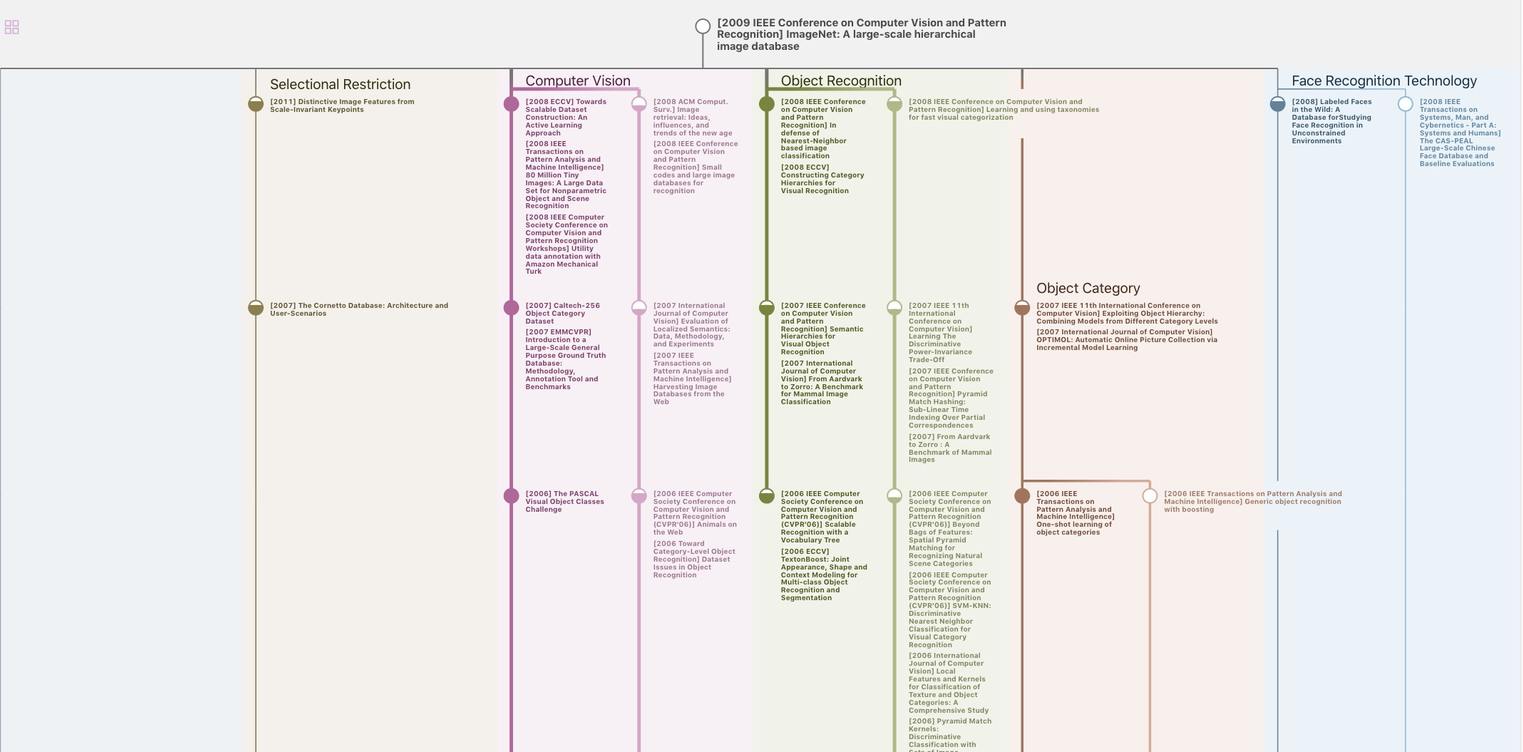
生成溯源树,研究论文发展脉络
Chat Paper
正在生成论文摘要