Adversarial open set domain adaptation via progressive selection of transferable target samples
Neurocomputing(2020)
摘要
In recent years, many Unsupervised Domain Adaptation (UDA) methods have been proposed to tackle the domain shift problem. Most existing UDA methods are derived for Close Set Domain Adaptation (CSDA) in which source and target domains are assumed to share the same label space. However, target domain may contain unknown class different from the known ones in the source domain in practice, i.e., Open Set Domain Adaptation (OSDA). Due to the presence of unknown class, aligning the whole distribution of the source and target domain for OSDA as in the previous methods will lead to negative transfer. Existing methods developed for OSDA attempt to assign smaller weights to target samples of unknown class. Despite promising performance achieved by existing methods, the samples of the unknown class are still used for distribution alignment, which makes the model suffer from the risk of negative transfer. Instead of reweighting, this paper presents a novel method namely Thresholded Domain Adversarial Network (ThDAN), which progressively selects transferable target samples for distribution alignment. Based on the fact that samples from the known classes must be more transferable than target samples of the unknown one, we derive a criterion to quantify the transferability by constructing classifiers to categorize known classes and to discriminate unknown class. In ThDAN, an adaptive threshold is calculated by averaging transferability scores of source domain samples to select target samples for training. The threshold is tweaked progressively during the training process so that more and more target samples from the known classes can be correctly selected for adversarial training. Extensive experiments show that the proposed method outperforms state-of-the-art domain adaptation and open set recognition approaches on benchmarks.
更多查看译文
关键词
Open set domain adaptation,Adversarial learning,Progressive selection
AI 理解论文
溯源树
样例
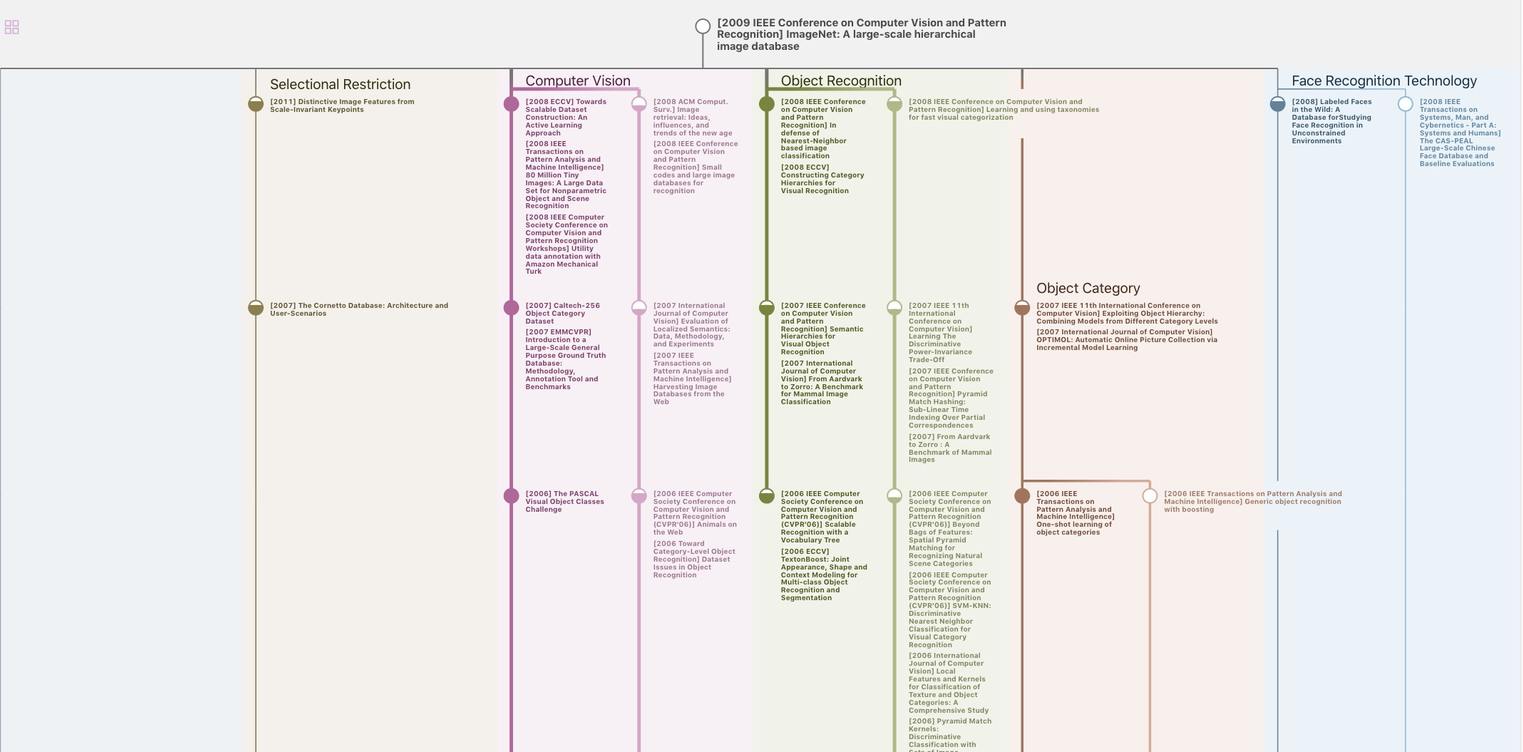
生成溯源树,研究论文发展脉络
Chat Paper
正在生成论文摘要