AQA: An Adaptive Quality Assessment Framework for Online Review Systems
IEEE Transactions on Services Computing(2022)
摘要
Computing robust and accurate quality scores for users and items in online review systems is critical, since scores directly reflect the community-wide belief about their quality. A broad range of methods have been proposed to compute rating scores, including simple aggregation, weighted aggregation, and iterative techniques, where the latter provides relatively accurate results. However, there are still serious challenges to address, especially in terms of time complexity, accuracy, and robustness against manipulation. In this article, we propose an adaptive quality assessment framework that computes dependable and accurate quality scores for users and items. The proposed method is a semi-iterative weighted aggregation technique in which, a novel approach is used to assign weights to received reviews. The weight depends on two parameters: similarity of reviews, and review prediction. In review prediction, we utilize a combination of online machine learning and collaborative filtering to predict the review expected from the user. The intuition behind using online learning is its ability to obtain lower time complexity in comparison with batch learning. We evaluate our proposed model using a real-word dataset, and compare it with two related approaches. Results show the superiority of our proposed approach, in terms of accuracy and robustness against manipulation.
更多查看译文
关键词
Online review systems,review prediction,quality assessment
AI 理解论文
溯源树
样例
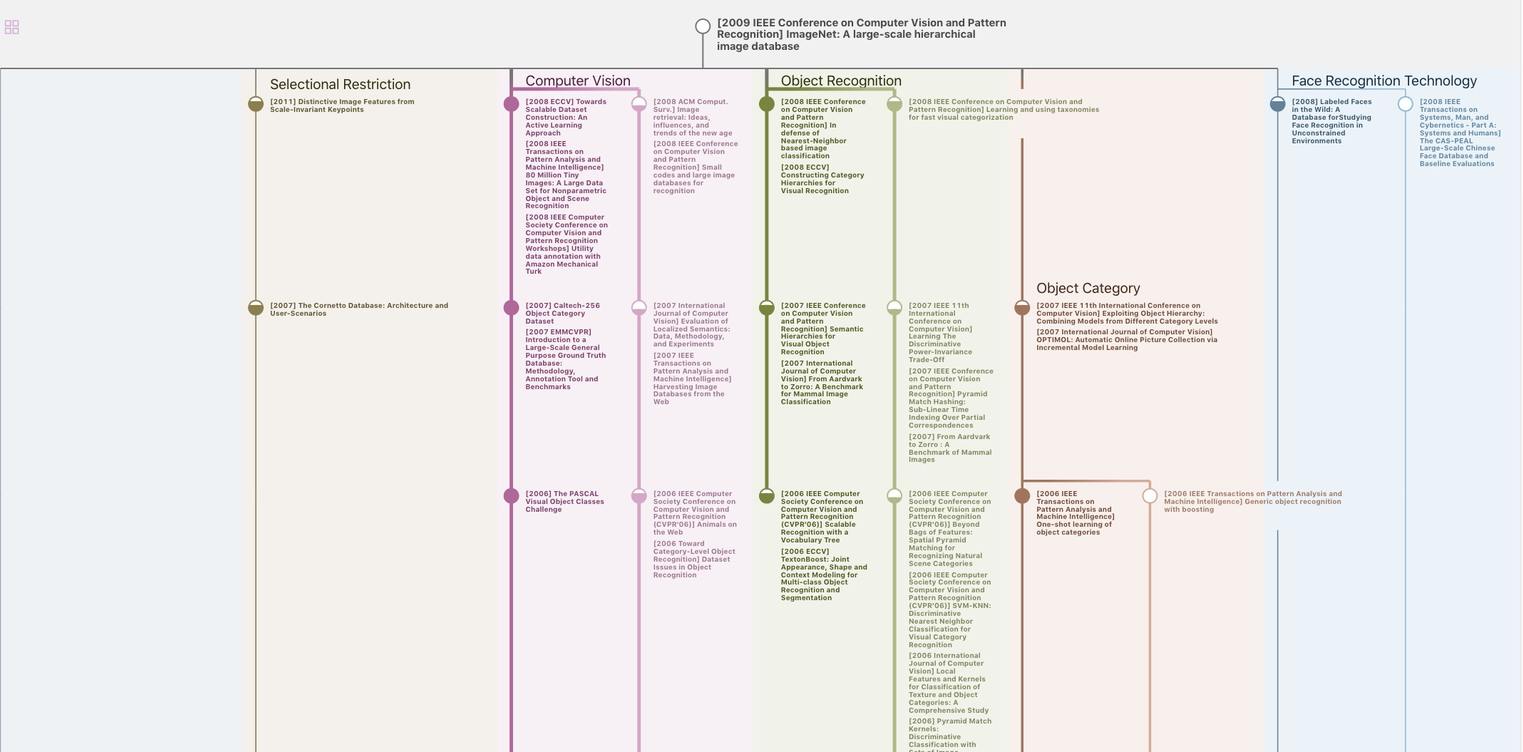
生成溯源树,研究论文发展脉络
Chat Paper
正在生成论文摘要