Oil Spill Detection Using Refined Convolutional Neural Network Based On Quad-Polarimetric Sar Images
PROCEEDINGS OF 2019 14TH IEEE INTERNATIONAL CONFERENCE ON ELECTRONIC MEASUREMENT & INSTRUMENTS (ICEMI)(2019)
Abstract
Quad-polarimetric SAR data has been proved to be useful for marine oil spill classification. Different SAR polarimetric features have been proposed to discriminate between oil spills and look-alikes which could cause false detection. In this paper we explored the ability of convolutional neural network (CNN) in automatic oil spill classification, by taking the advantage of H/A/Alpha polarimetric decomposition features and co-polarized correlation coefficients(CC). The convolutional neural network (CNN) was refined to realize the classification, in which global average pooling layer is applied instead of full connection layer. The quad-polarimetric Radarsat-2 data acquired during the Norwegian oil-on-water exercise was tested in the experiment. Sea surface was classified as clean sea, oil spill, look-alikes(biological oil spill in this case), and emulsion. The experiment results show that H/A/Alpha parameters and the combination of H/A/Alpha and co-polarized CC obtained higher accuracy, and the refined CNN has better performance than the traditional one in terms of accuracy and efficiency.
MoreTranslated text
Key words
Synthetic Aperture Radar, oil spill detection, Convolutional Neural Network, polarimetric decomposition
AI Read Science
Must-Reading Tree
Example
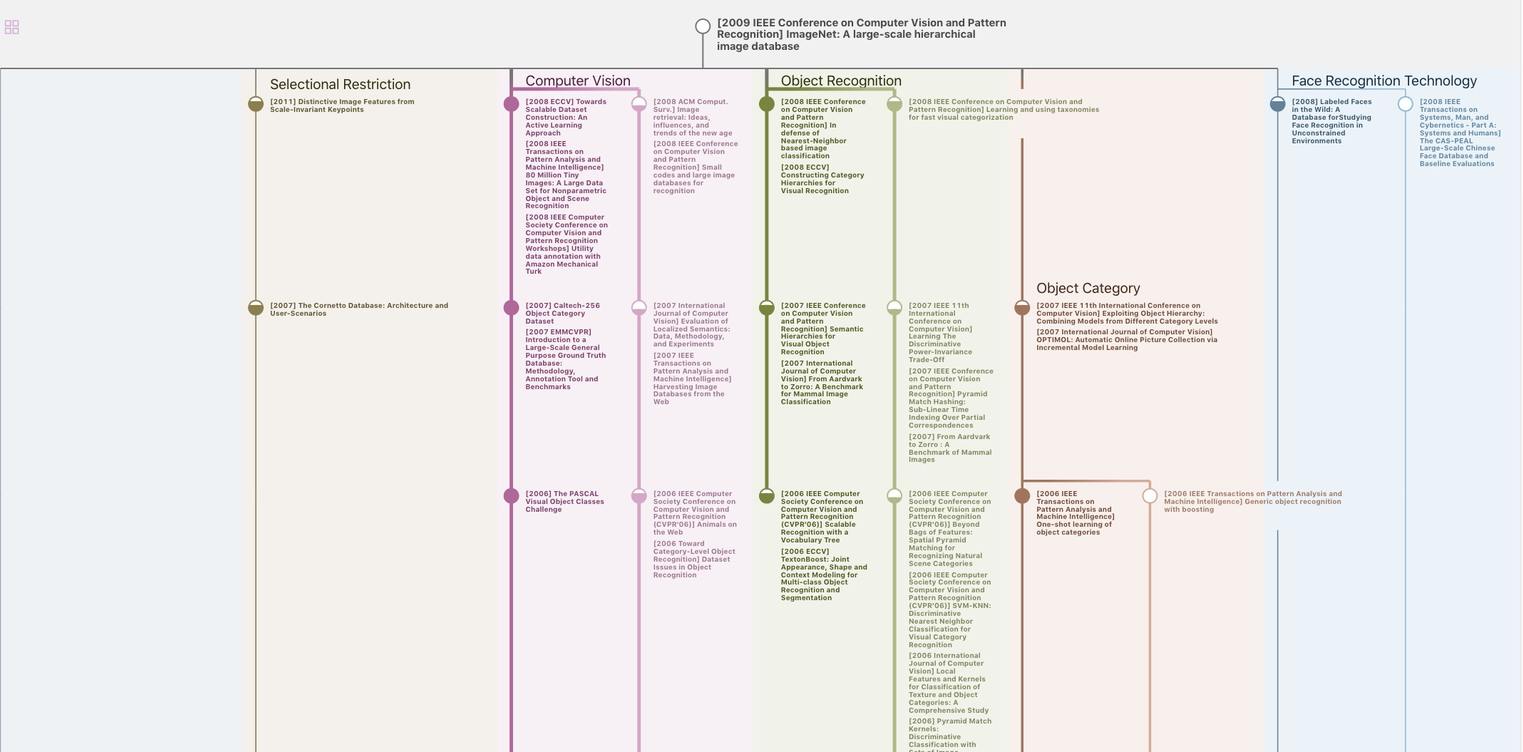
Generate MRT to find the research sequence of this paper
Chat Paper
Summary is being generated by the instructions you defined