Sketched Sparse Subspace Clustering For Large-Scale Hyperspectral Images
2020 IEEE INTERNATIONAL CONFERENCE ON IMAGE PROCESSING (ICIP)(2020)
摘要
Sparse subspace clustering (SSC) has achieved the state-of-the-art performance in clustering of hyperspectral images. However, the computational complexity of SSC-based methods is prohibitive for large-scale problems. We propose a large-scale SSC-based method, which processes efficiently large-scale HSIs without sacrificing the clustering accuracy. The proposed approach incorporates sketching of the self-representation dictionary reducing thereby largely the number of optimization variables. In addition, we employ a total variation (TV) regularization of the sparse matrix, resulting in a robust sparse representation. We derive a solver based on the alternating direction method of multipliers (AD-MM) for the resulting optimization problem. Experimental results on real data show improvements over the traditional SSC-based methods in terms of accuracy and running time.
更多查看译文
关键词
Sparse subspace clustering, sketching, hyperspectral image, large-scale data
AI 理解论文
溯源树
样例
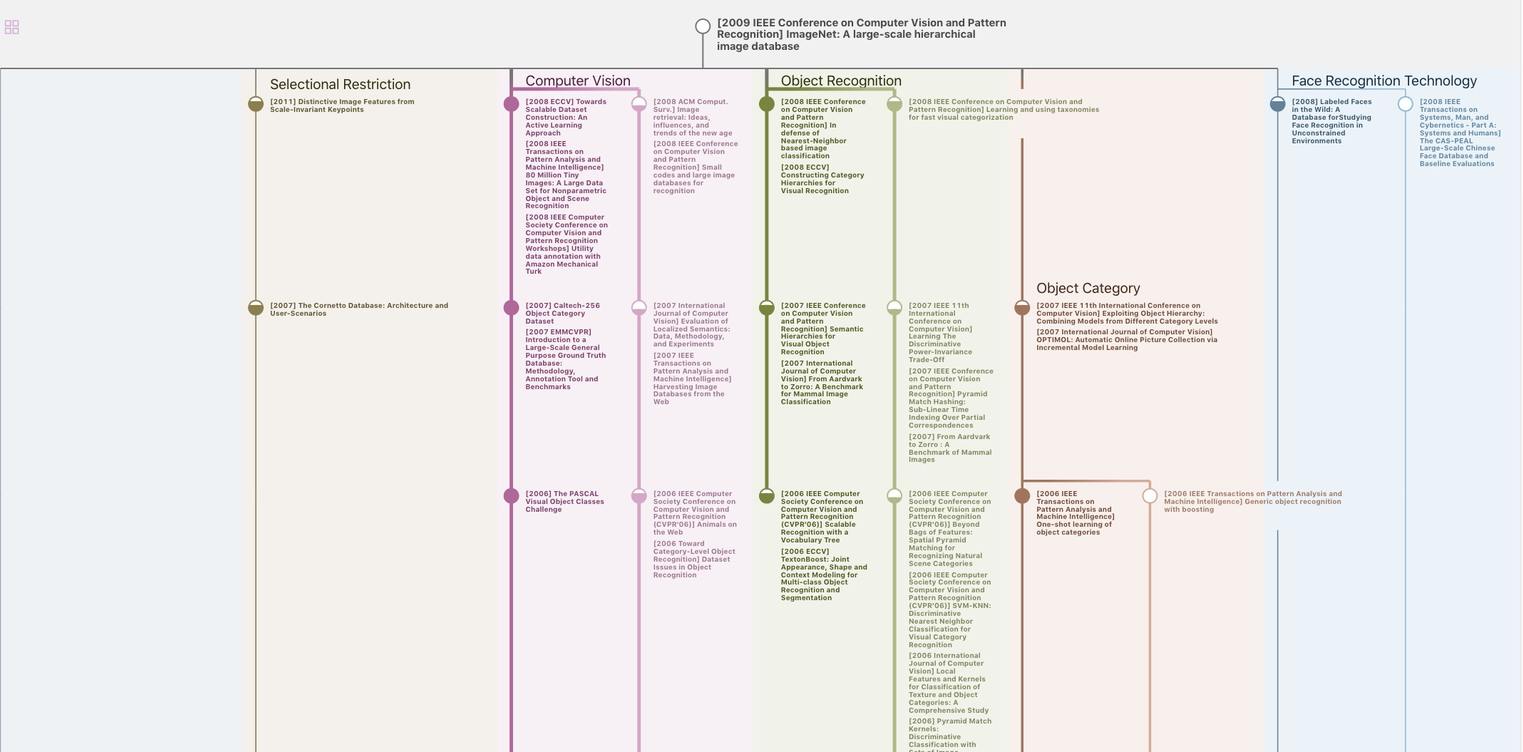
生成溯源树,研究论文发展脉络
Chat Paper
正在生成论文摘要