Predicting leaf nitrogen content in olive trees using hyperspectral data for precision agriculture
PRECISION AGRICULTURE(2020)
摘要
Olive orchard is one of the main crops in the Mediterranean basin and, particularly, in Spain, with 56% of European production. In semi-arid regions, nitrogen (N) is the main limiting factor of olive trees after water and its quantification is essential to carry out accurate fertilization planning. In the present study, N status of an olive orchard located in Carmonita (southwest Spain) was analysed using hyperspectral data. Reflectance data were recorded with a high precision spectro-radiometer through the full spectrum (350–2500 nm). Different vegetation indices (VI), combining two or three wavelengths, and partial least squares regression (PLSR) models were developed, and the prediction capabilities were compared. Different pre-processing (smoothing, SM; standard normal variate, SNV; first and second derivative) were applied to analyse the influence of the noise generated by the spectro-radiometer measurements when computing the determination coefficient between leaf N content (LNC) and spectra data. Results showed that second derivative combined with SNV pre-processing produced the best determination coefficients. The wavelengths most sensitive to N variation used to perform VI were selected from the visible and the short-wave infrared spectrum regions, which relate to chlorophyll a + b and N absorption features. DCNI and TCARI showed the best fittings for the LNC prediction (R 2 = 0.72, R 2 cv = 0.71; and R 2 = 0.64, R 2 cv = 0.63, respectively). PLSR models yielded higher accuracy than the models based on VI (R 2 = 0.98, R 2 cv = 0.56), although the large difference between calibration and cross-validation showed more uncertainty in the PLSR models.
更多查看译文
关键词
Leaf nutritional status, Linear regression, Nitrogen indices, Olive orchards, Partial least squares regression, SWIR spectral region
AI 理解论文
溯源树
样例
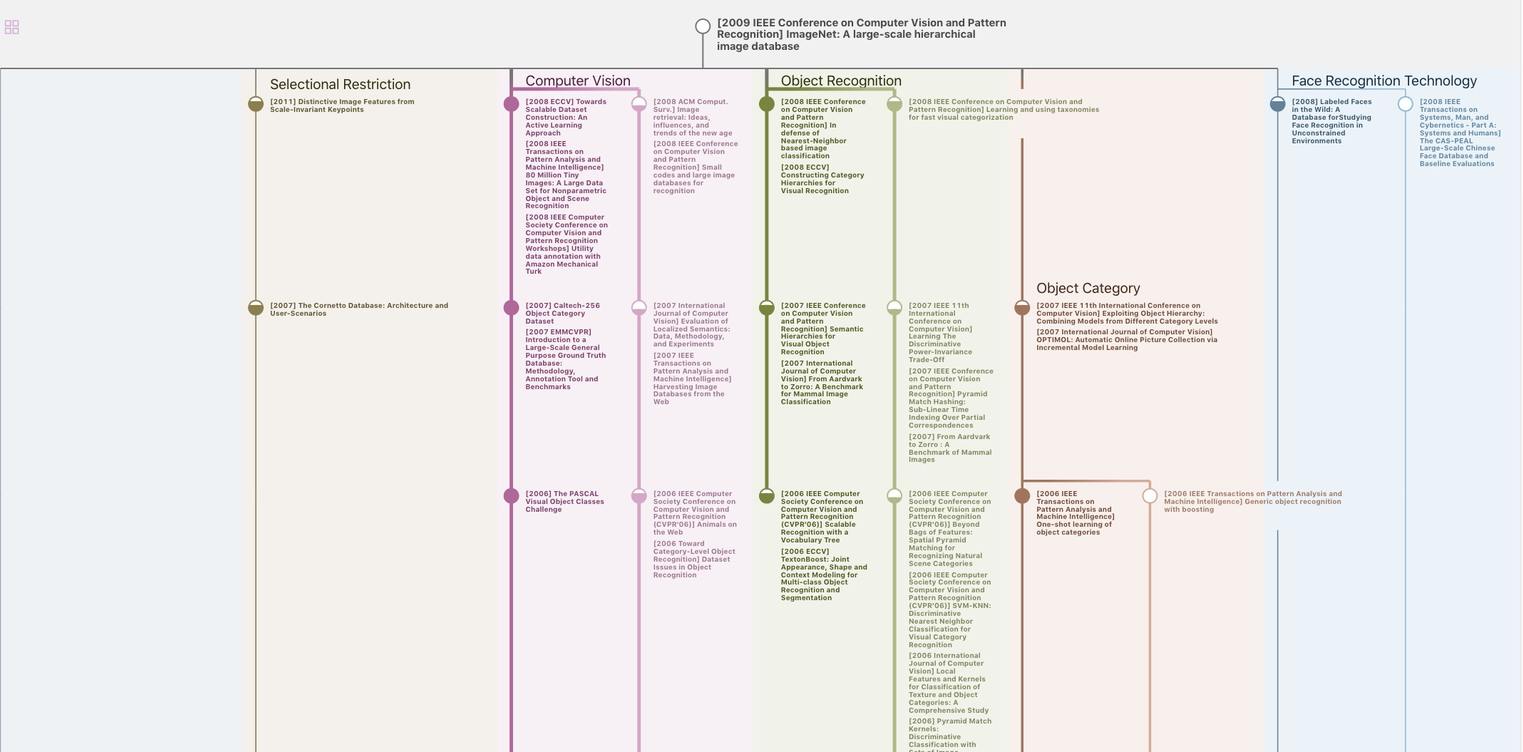
生成溯源树,研究论文发展脉络
Chat Paper
正在生成论文摘要