A Parallel-Data-Free Speech Enhancement Method Using Multi-Objective Learning Cycle-Consistent Generative Adversarial Network
IEEE-ACM TRANSACTIONS ON AUDIO SPEECH AND LANGUAGE PROCESSING(2020)
摘要
Recently, deep neural networks (DNNs) have become the mainstream strategy for speech enhancement task because it can achieve the higher speech quality and intelligibility than the traditional methods. However, these DNN-based methods always need a large number of parallel corpus consisting of clean speech and noise to produce noisy data for the training of the DNN in order to improve the generalization of the network. As a result, this implies that many noisy speech signals that are collected in real environment cannot be used to train the DNN because of the lack of corresponding clean speech and noise. Additionally, as we know, noise varies with the time and scenario, so we cannot obtain parallel speech and noise due to infinite noise data and some limited speech data. Thus, the network training with unparallel speech and noise data is essential for the generalization of the network. To address this problem, we propose a novel parallel-data-free speech enhancement method, in which the cycle-consistent generative adversarial network (CycleGAN) and multi-objective learning are employed. Our method is also able to make best use of the benefits of multi-objective learning. On the training stage, we utilize two different encoders to encode the features of clean speech and noisy speech, respectively. Then, two forward generators are immediately used to predict the ideal time-frequency (T-F) mask and log-power spectrum (LPS) of clean speech. Two inverse generators are applied to map the magnitude spectrum (MS) and LPS of noisy speech, respectively. In addition, four discriminators are used to distinguish the real speech features from the generated features. Two encoders, four generators and four discriminators are simultaneously trained by using adversarial, identity-mapping, latent similarity and cycle-consistent loss. On the test stage, we directly utilize the forward generators and encoders to acquire the enhanced speech. The experimental results indicate that the proposed approach is able to achieve the better speech enhancement performance than the reference methods. Moreover, the proposed method is also effective to improve speech quality and intelligibility when the networks are trained under the parallel data.
更多查看译文
关键词
Speech enhancement,Noise measurement,Generative adversarial networks,Generators,Task analysis,Gallium nitride,Cycle-consistent adversarial network,deep neural networks,multi-objective learning,non-parallel data,speech enhancement
AI 理解论文
溯源树
样例
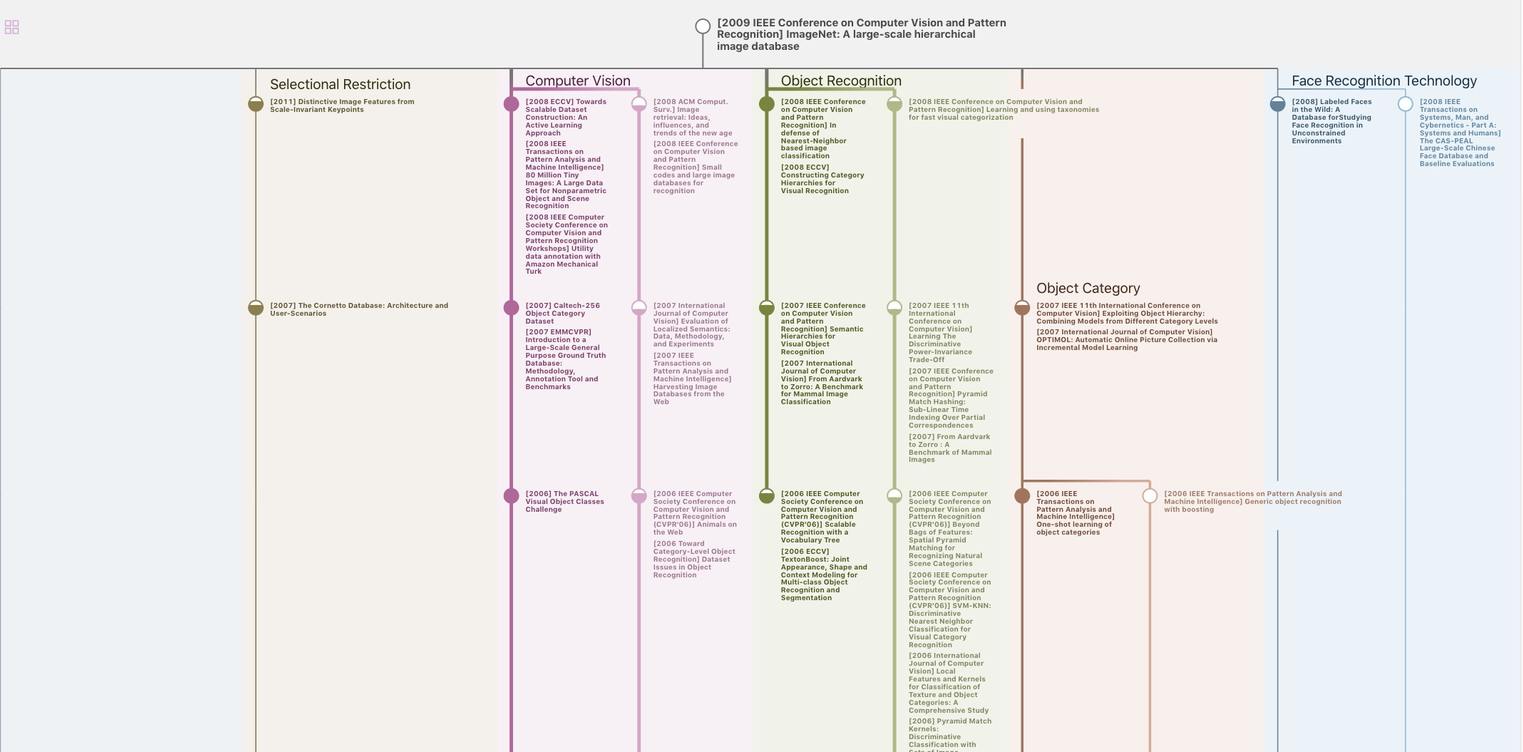
生成溯源树,研究论文发展脉络
Chat Paper
正在生成论文摘要