Improving search ranking of geospatial data based on deep learning using user behavior data
Computers & Geosciences(2020)
摘要
Finding geospatial data has been a big challenge regarding the data size and heterogeneity across various domains. Previous work has explored using machine learning to improve geospatial data search ranking, but it usually relies on training data labelled by subject matter experts, which makes it laborious and costly to apply to scenarios in which data relevancy to a query can change over time. When a user interacts with a search engine, plenteous information is recorded in the log file, which is essentially free, sustainable and up-to-the-minute. In this research, we propose a deep learning-based search ranking framework that can expeditiously update the ranking model through capturing real-time user clickstream data. The contributions of the proposed framework consist of 1) a log parser that can ingest and parse Web logs that record users’ behavior in a real-time manner; 2) a set of hypotheses of modelling the relative relevance of data; and 3) a deep learning based ranking model which can be updated dynamically with the increment of user behavior data. Quantitative comparison with a few other machine learning algorithms suggests substantial improvement.
更多查看译文
关键词
Deep learning,User behavior,Search engine,Knowledge discovery,Artificial intelligence
AI 理解论文
溯源树
样例
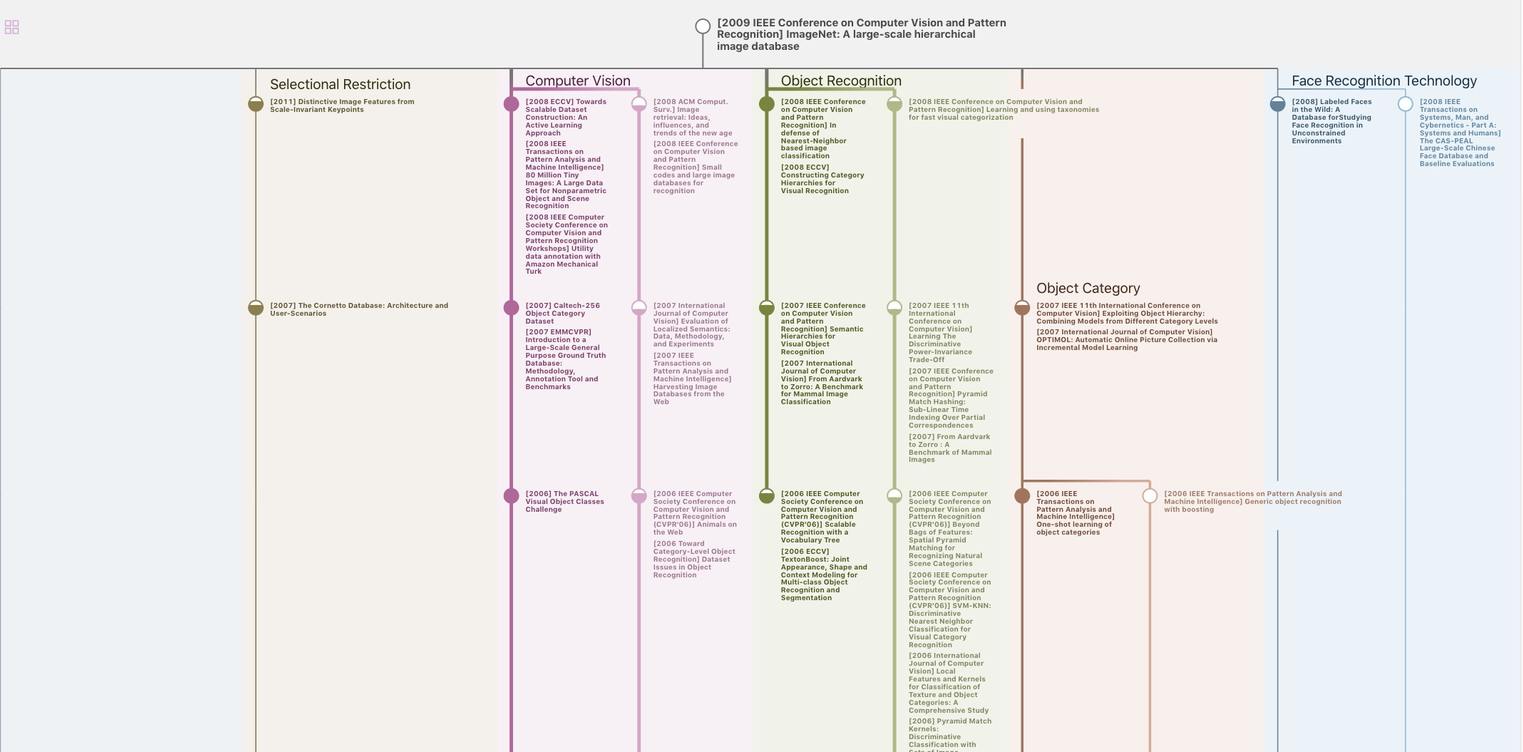
生成溯源树,研究论文发展脉络
Chat Paper
正在生成论文摘要