Reinforcement learning for dynamic condition-based maintenance of a system with individually repairable components
QUALITY ENGINEERING(2020)
摘要
In this article, a reinforcement learning approach is used to develop a new dynamic maintenance policy for multi-component systems with individually repairable components, where each component experiences two competing failure processes of degradation and random shocks. The gamma process is used to model the degradation path of each component in the system. It is assumed that each incoming shock causes damage to the degradation path of all the components. A combination of component degradation is then used to define the system states. The optimal maintenance action for each component at each specific state is found by modeling the problem as Markov decision process and solving it by using a Q-learning algorithm. Using a reinforcement learning approach provides a more time-efficient and cost-effective method compared to the traditional maintenance optimization solutions, and it can also provide a dynamic maintenance policy for each specific degradation state of the system which is more useful and beneficial compared to the fixed or stationary maintenance plan which is often proposed by previous studies. A numerical example shows how the reinforcement learning can be used to find the optimal maintenance action for systems with different system configurations.
更多查看译文
关键词
reinforcement learning,Markov decision process,Q-learning,condition-based maintenance,gamma process,competing failure processes
AI 理解论文
溯源树
样例
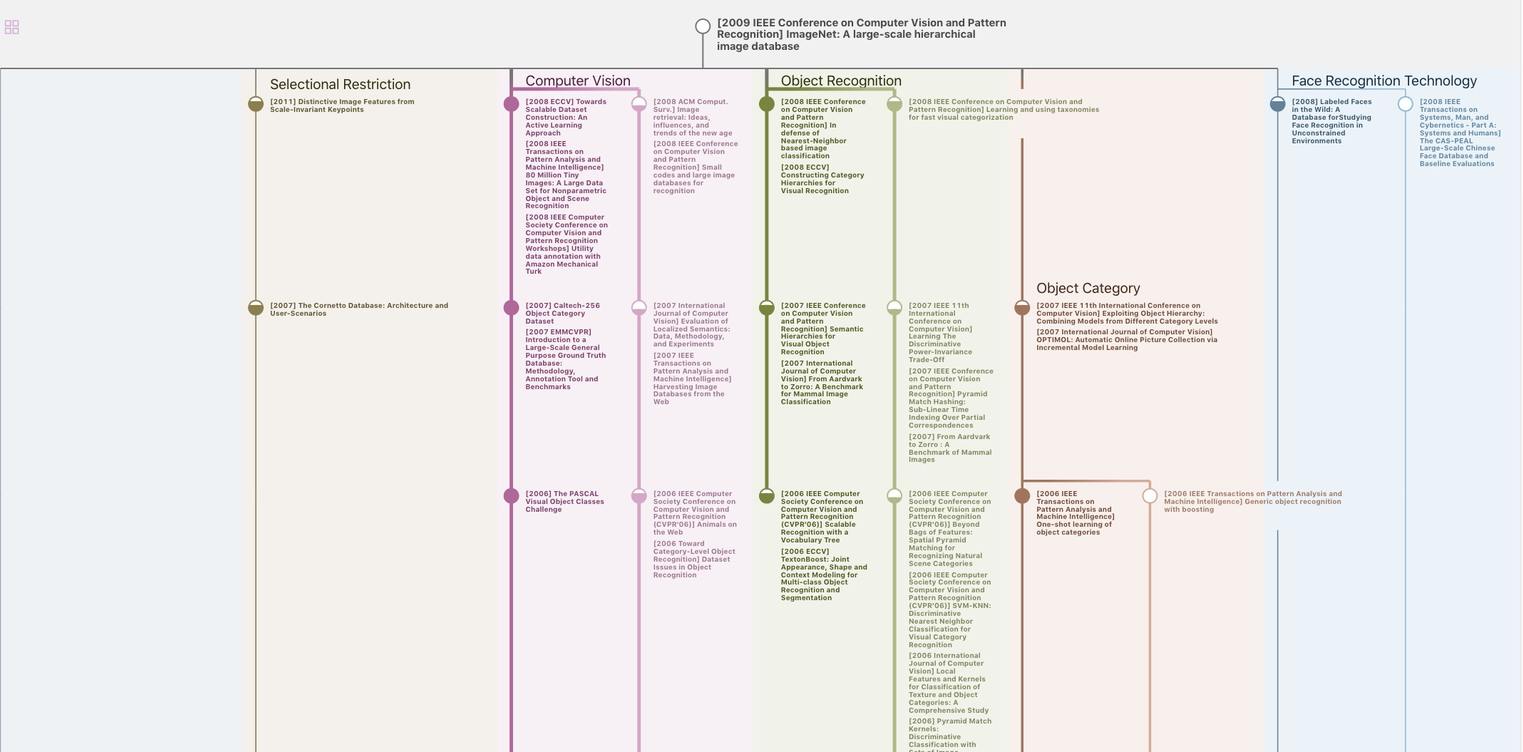
生成溯源树,研究论文发展脉络
Chat Paper
正在生成论文摘要