Face Spoofing Detection Using Relativity Representation On Riemannian Manifold
IEEE TRANSACTIONS ON INFORMATION FORENSICS AND SECURITY(2020)
摘要
Face recognition and verification systems are susceptible to spoofing attacks using photographs, videos or masks. Most existing methods focus on spoofing detection in Euclidean space, and ignore the features' manifold structure and interrelationships, thus limiting their capabilities of discrimination and generalization. In this paper, we propose a relativity representation on Riemannian manifold for face spoofing detection. The relativity representation improves generalization capability while ensuring discriminability, at both levels of feature description and classification score. The feature-level relativity representation generalizes information by modeling interrelationships among basic features, and would not depend too much on characteristics of a particular dataset. The score-level relativity representation makes decisions relatively, not absolutely, according to interrelationships (via Riemannian metric) and competitions (via example reweighting) among data samples on Riemannian manifold. The discriminability is ensured by the high-order nature of the feature-level relativity representation as well as Riemannian reweighted discriminative learning of the score-level relativity representation. Moreover, we integrate an attack-sensitive SVM classifier in Euclidean space to improve spoofing detection. Experiments demonstrate the effectiveness of our method on both intra-dataset and cross-dataset testing.
更多查看译文
关键词
Feature extraction, Face, Measurement, Manifolds, Videos, Support vector machines, Kernel, Face spoofing detection, relativity representation, Riemannian manifold, Riemannian reweighted discriminative learning
AI 理解论文
溯源树
样例
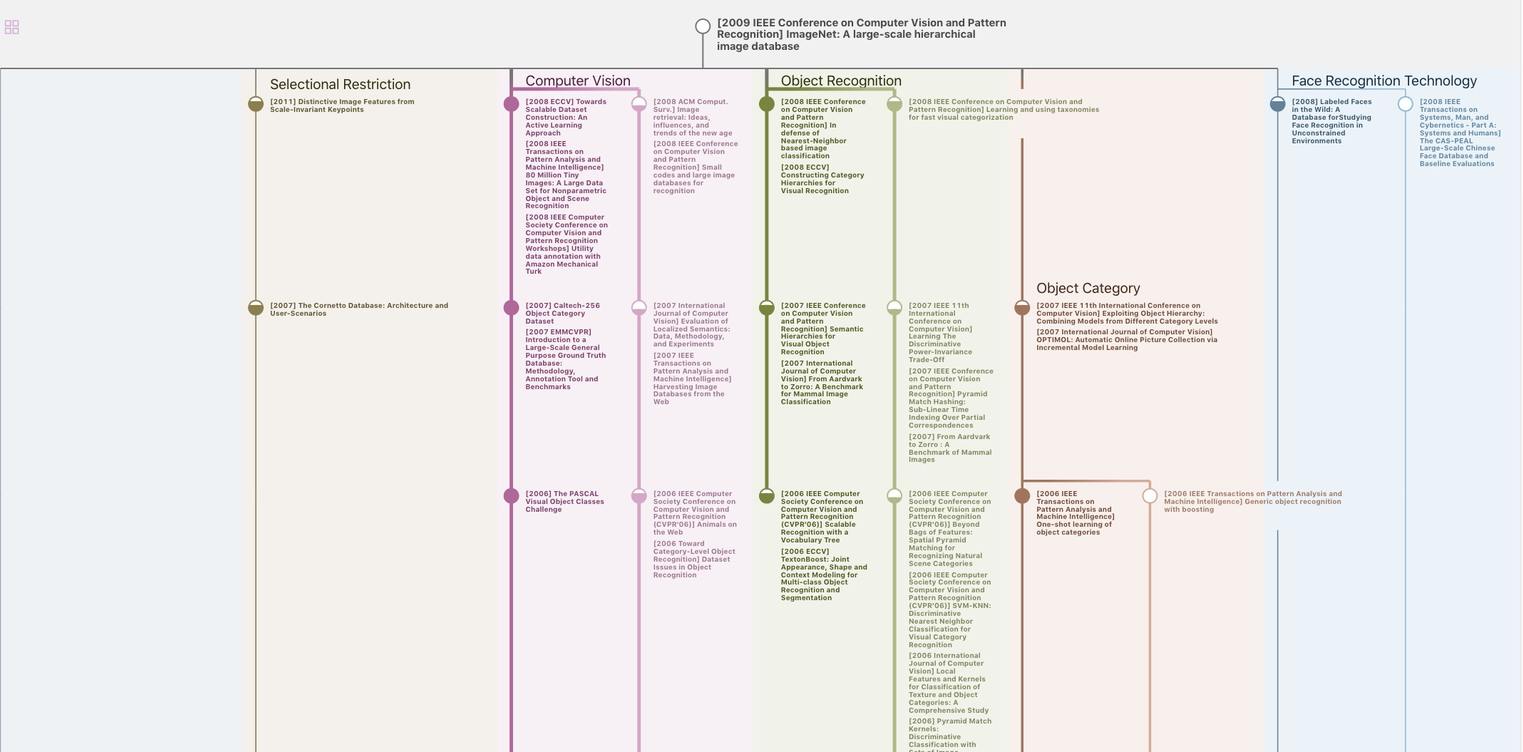
生成溯源树,研究论文发展脉络
Chat Paper
正在生成论文摘要