Single-Spectrum-Driven Binary-Class Sparse Representation Target Detector for Hyperspectral Imagery
IEEE Transactions on Geoscience and Remote Sensing(2021)
摘要
In this article, a single-spectrum-driven binary-class sparse representation target detector (SSBSTD) via target and background dictionary construction (BDC) is proposed. The SSBSTD leans upon the binary-class sparse representation (BSR) model. Due to the fact that a background spectrum usually consists in background samples composed low-dimensional subspace and a target spectrum also consists in target samples composed low-dimensional subspace, only background samples should be used for sparsely representing the test pixel under the target absent hypothesis and the samples from target-only dictionary for target present hypothesis. To alleviate the problem that there are insufficient available target samples in the sparse representation model, this article proposed a predetection method to construct the target dictionary utilizing the given target spectrum. With regard to the BDC, we proposed an approach based on the classification to generate a global over-complete background dictionary. The detection output is composed of the residual difference between the BSR. Extensive experiments were made on four benchmark hyperspectral images and the experimental results indicate that our SSBSTD algorithm demonstrates superior detection performances.
更多查看译文
关键词
Binary hypothesis,hyperspectral imagery (HSI),sparse representation,target detection
AI 理解论文
溯源树
样例
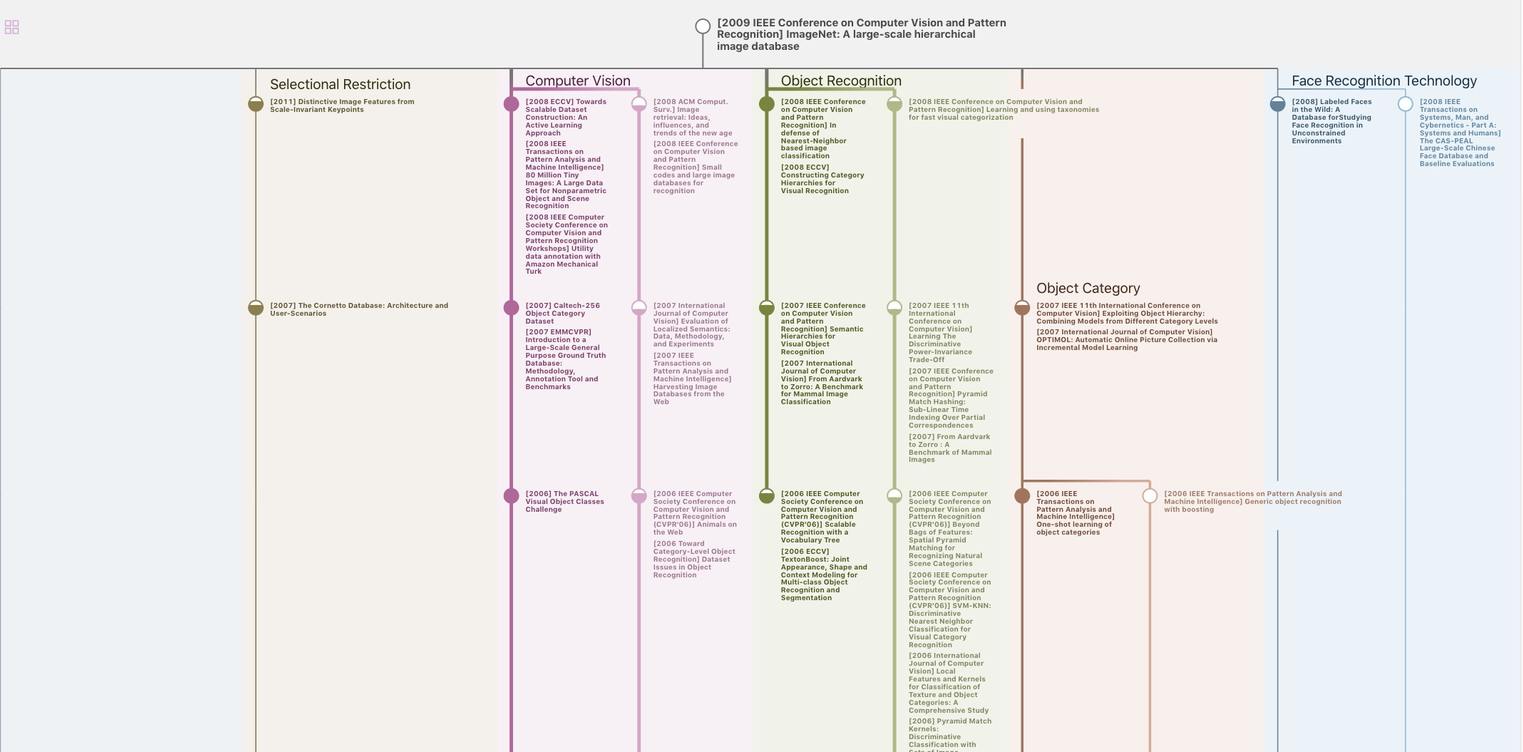
生成溯源树,研究论文发展脉络
Chat Paper
正在生成论文摘要