Multimodal Graph Convolutional Networks for High Quality Content Recognition
Neurocomputing(2020)
摘要
With the development of the Internet, more and more creators publish articles on social media. How to automatically filter high quality content from a large number of multimedia articles is one of the core functions of information recommendation, search engine, and other systems. However, existing approaches typically suffer from two limitations: (1) They usually model content as word sequences, which ignores the semantics provided by non-consecutive phrases, long-distance word dependency, and visual information. (2) They rely on a large amount of manually annotated data to train a quality assessment model while users may only provide labels of interest in a single class for a small number of samples in reality. To address these limitations, we propose a Multimodal Graph Convolutional Networks (MGCN) to model the semantic representations in a unified framework for High Quality Content Recognition. Instead of viewing text content as word sequences, we convert them into graphs, which can model non-consecutive phrases and long-distance word dependency for better obtaining the composition of semantics. Besides, visual content is also modeled into the graphs to provide complementary semantics. A well-designed graph convolutional network is proposed to capture the semantic representations based on these graphs. Furthermore, we employ a non-negative risk estimator for high quality content recognition and the loss is back-propagated for model learning. Experiments on real datasets validate the effectiveness of our approach.
更多查看译文
关键词
High quality content recognition,Graph convolutional networks,Positive unlabeled learning
AI 理解论文
溯源树
样例
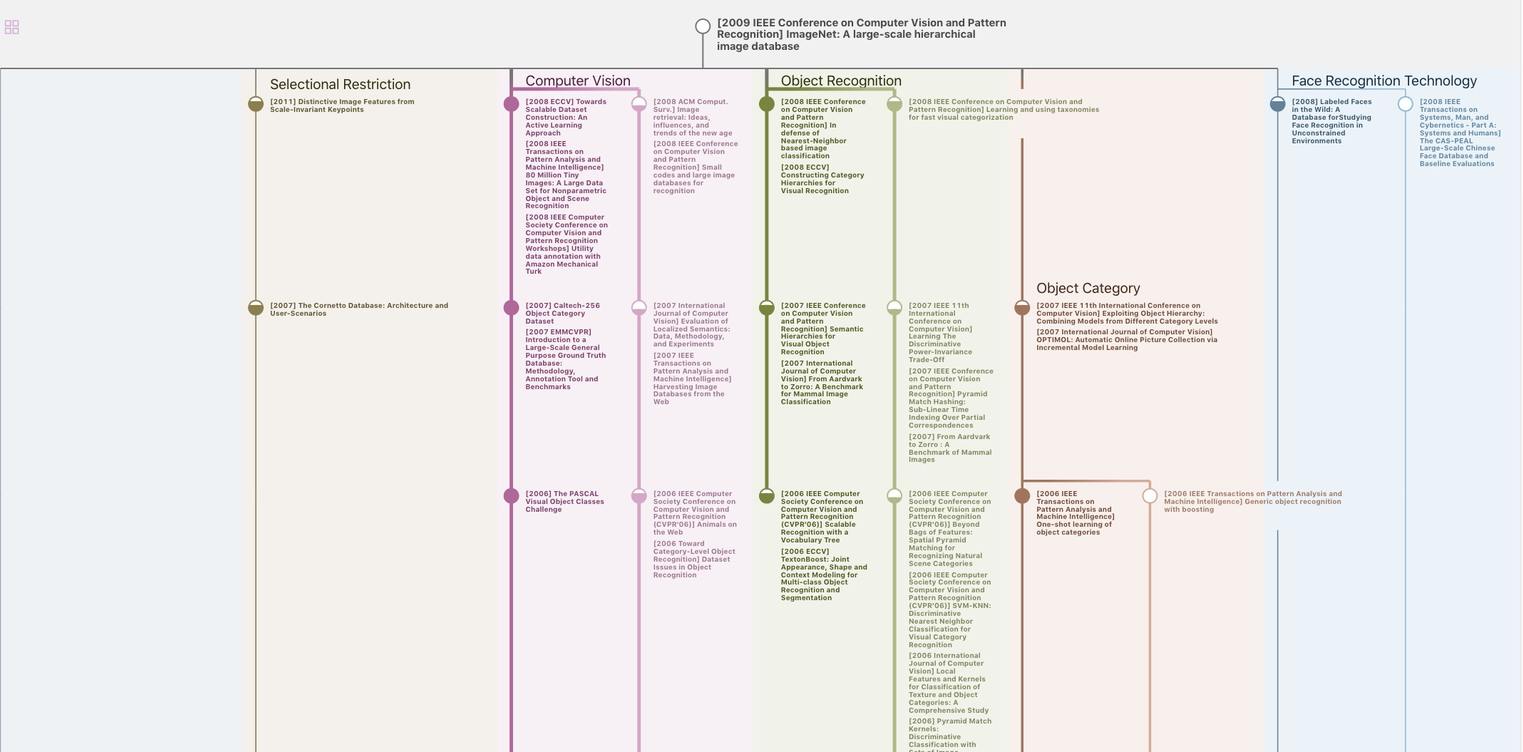
生成溯源树,研究论文发展脉络
Chat Paper
正在生成论文摘要