Audio Replay Spoof Attack Detection by Joint Segment-Based Linear Filter Bank Feature Extraction and Attention-Enhanced DenseNet-BiLSTM Network
IEEE/ACM transactions on audio, speech, and language processing(2020)
摘要
Most automatic speaker verification (ASV) systems are vulnerable to various spoofing attacks. In recent years, there have been many methods were proposed for detecting spoofing attacks in ASV, and significant progress has been made. However, current methods have shown little improvements in replay spoof attack detection as they lack a more suitable model for replay detection. To address this issue, in this article, we propose a novel model based on attention-enhanced DenseNet-BiLSTM network and segment-based linear filter bank features. First, silent segments are selected from each speech signal by using a short-term zero-crossing rate and energy. If the total duration of silent segments only contains a very limited amount of data, the decaying tails will be selected instead. Second, the linear filter bank features are extracted from the selected segments in the relatively high-frequency domain. Finally, an attention-enhanced DenseNet-BiLSTM architecture which can avoid the problems of overfitting is built. To validate this model, we used two datasets, including BTAS2016 and ASVspoof2017. Experiments show that using the attention-enhanced DenseNet-BiLSTM model with the segment-based linear filter bank feature achieves the best performance. Compared with the baseline system based on constant Q cepstral coefficient and Gaussian mixture model (GMM), the proposed model can produce a relative improvement of 91.68% and 74.04% on the two data sets respectively.
更多查看译文
关键词
Feature extraction,Speech processing,Mel frequency cepstral coefficient,Deep learning,Speech recognition,Task analysis,Filter banks,Attack detection,attention-enhanced,denseNet-BiLSTM network,linear filter bank feature,replay spoof
AI 理解论文
溯源树
样例
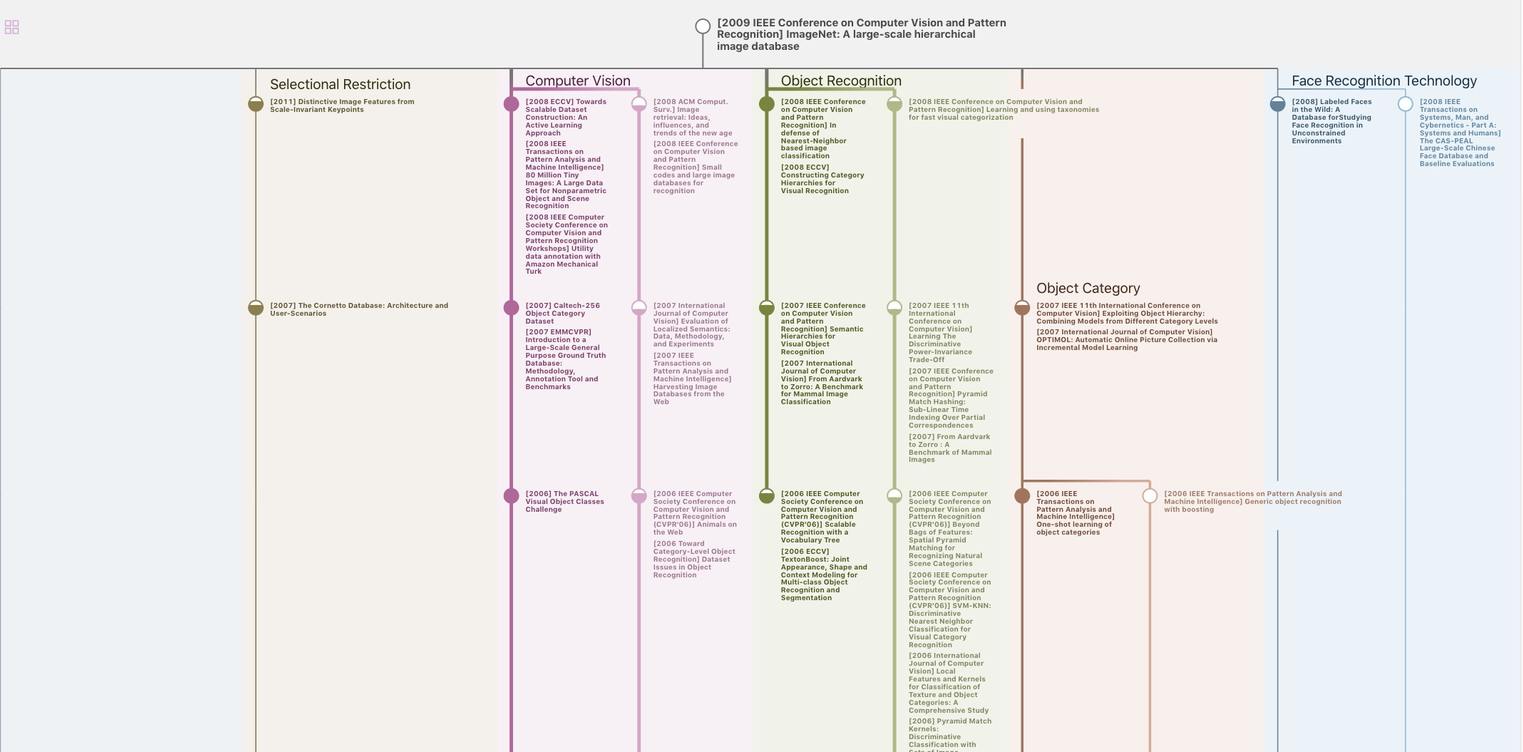
生成溯源树,研究论文发展脉络
Chat Paper
正在生成论文摘要