Superpixel Region Merging Based on Deep Network for Medical Image Segmentation
ACM Transactions on Intelligent Systems and Technology(2020)
摘要
AbstractAutomatic and accurate semantic segmentation of pathological structures in medical images is challenging because of noisy disturbance, deformable shapes of pathology, and low contrast between soft tissues. Classical superpixel-based classification algorithms suffer from edge leakage due to complexity and heterogeneity inherent in medical images. Therefore, we propose a deep U-Net with superpixel region merging processing incorporated for edge enhancement to facilitate and optimize segmentation. Our approach combines three innovations: (1) different from deep learning--based image segmentation, the segmentation evolved from superpixel region merging via U-Net training getting rich semantic information, in addition to gray similarity; (2) a bilateral filtering module was adopted at the beginning of the network to eliminate external noise and enhance soft tissue contrast at edges of pathogy; and (3) a normalization layer was inserted after the convolutional layer at each feature scale, to prevent overfitting and increase the sensitivity to model parameters. This model was validated on lung CT, brain MR, and coronary CT datasets, respectively. Different superpixel methods and cross validation show the effectiveness of this architecture. The hyperparameter settings were empirically explored to achieve a good trade-off between the performance and efficiency, where a four-layer network achieves the best result in precision, recall, F-measure, and running speed. It was demonstrated that our method outperformed state-of-the-art networks, including FCN-16s, SegNet, PSPNet, DeepLabv3, and traditional U-Net, both quantitatively and qualitatively. Source code for the complete method is available at https://github.com/Leahnawho/Superpixel-network.
更多查看译文
关键词
Medical image segmentation, superpixel-based classification algorithm, deep U-Net, bilateral filtering, normalization layer
AI 理解论文
溯源树
样例
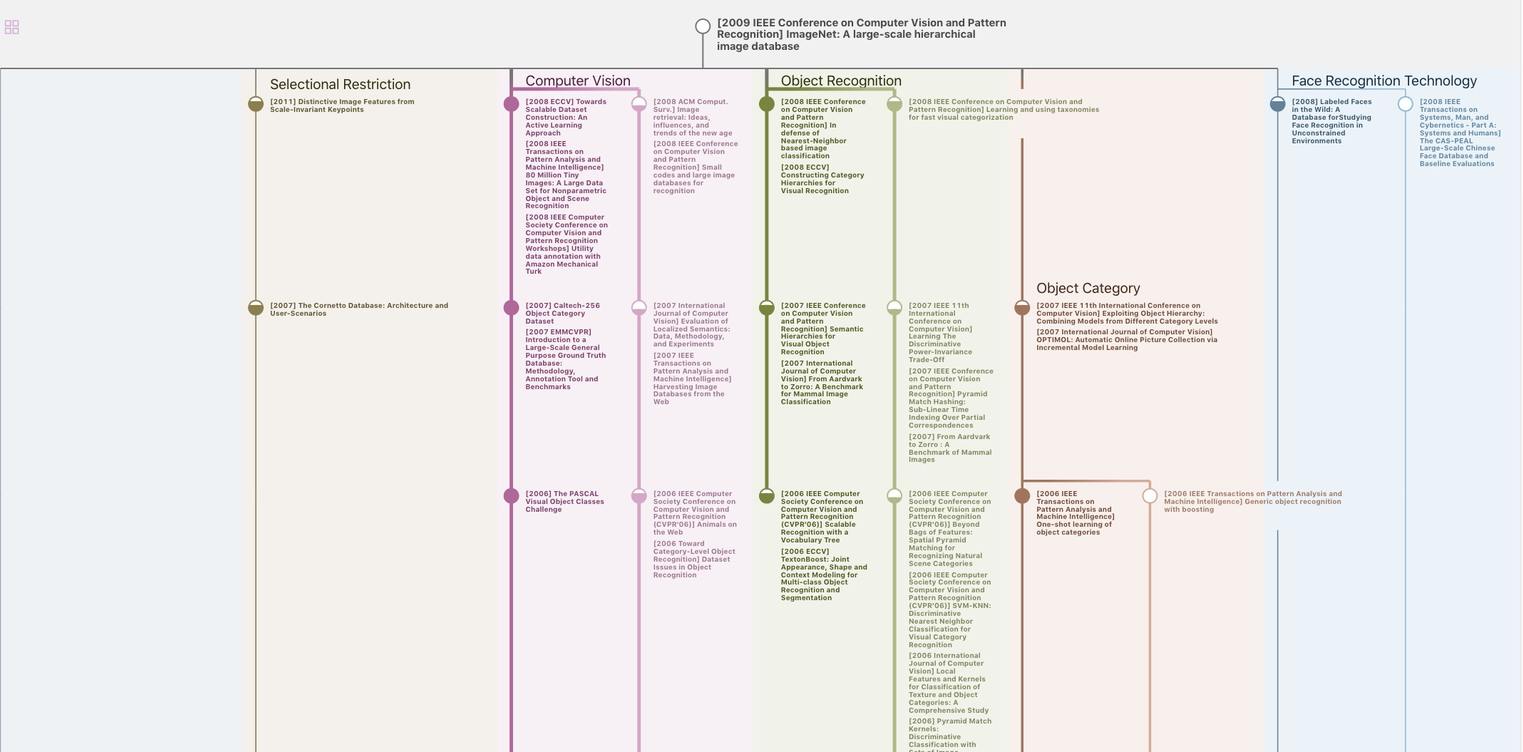
生成溯源树,研究论文发展脉络
Chat Paper
正在生成论文摘要