Efficient Online Interest-Driven Exploration for Developmental Robots
IEEE Transactions on Cognitive and Developmental Systems(2022)
摘要
A major challenge for online and data-driven model learning in robotics is the high sample complexity. This hinders its efficiency and practical feasibility for lifelong learning, in particular, for developmental robots that autonomously bootstrap their sensorimotor skills in an open-ended environment. In this work, we propose new methods to mediate this problem in order to permit the learning of robot models online, from scratch, and in learning while behaving fashion. Exploration is utilized and autonomously driven by a novel intrinsic motivation signal which combines knowledge-based and competence-based elements and surpasses other state-of-the-art methods. In addition, we propose an episodic online mental replay to accelerate online learning, to ensure sample efficiency, and to update the model online rapidly. The efficiency as well as the applicability of our methods are demonstrated with a physical 7-DoF Baxter manipulator. We show that our learning schemes are able to drastically reduce the sample complexity and learn the data-driven model online, even within a limited time frame.
更多查看译文
关键词
Data driven,developmental robot,efficient learning,intrinsic motivation,inverse kinematics (IK),inverse robot models,inverse statics,online learning
AI 理解论文
溯源树
样例
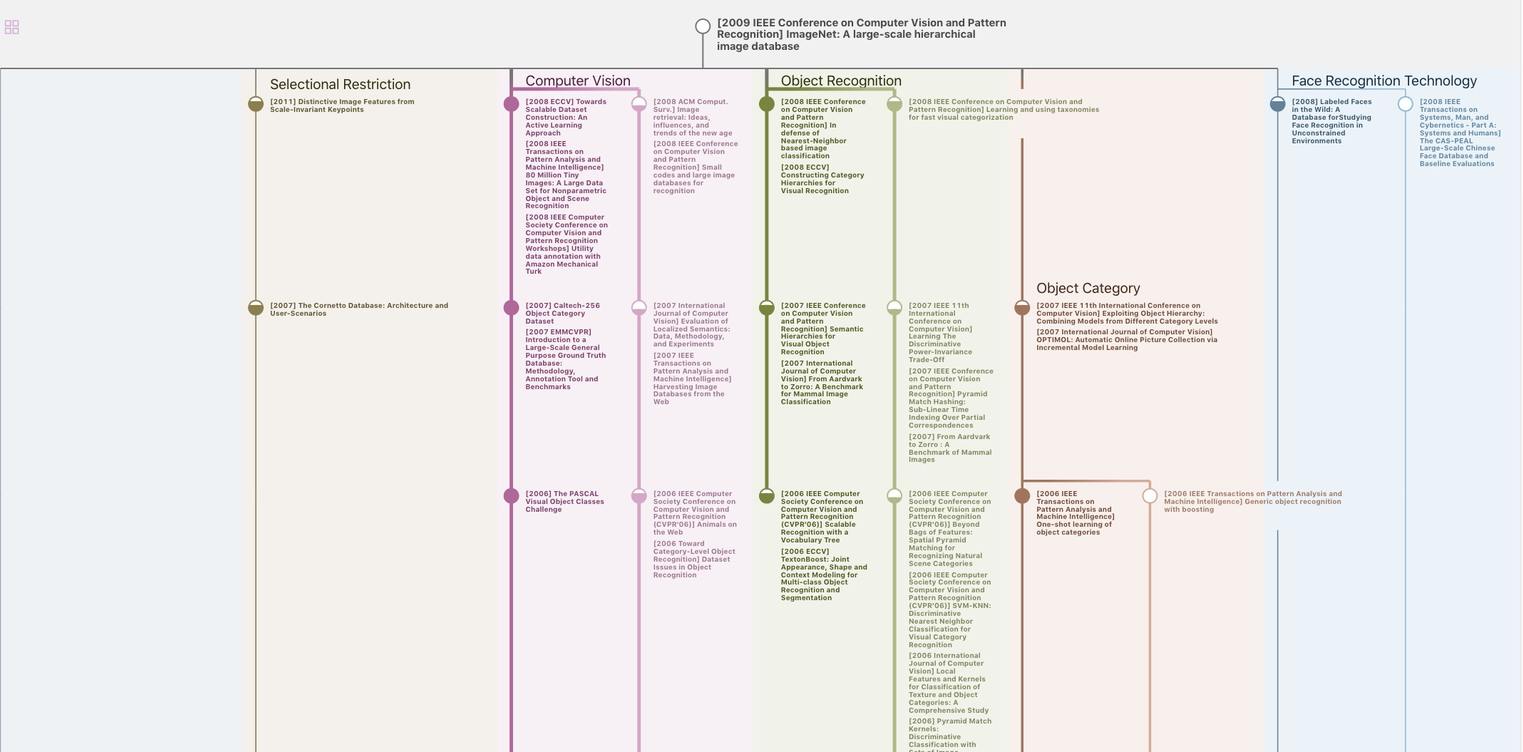
生成溯源树,研究论文发展脉络
Chat Paper
正在生成论文摘要